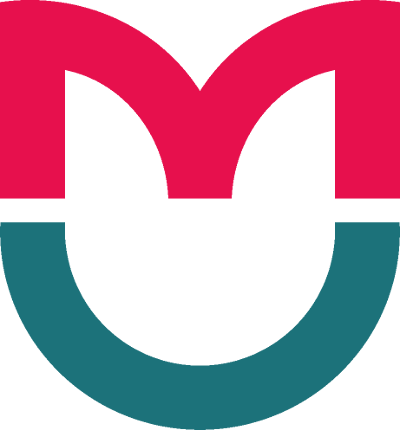
ISSN Print 2070–7320
ISSN Online 2070–7339
НАУЧНЫЙ МЕДИЦИНСКИЙ ЖУРНАЛ РНИМУ ИМЕНИ Н. И. ПИРОГОВА
Балтийский федеральный университет имени Иммануила Канта, Калининград, Россия
Вклад авторов в работу: В. Б. Савинов, С. А. Ботман, В. В. Сапунов и В. А. Петров — сбор и обработка материала, написание текста статьи; И. Г. Самусев — написание, редактирование текста статьи; Н. Н. Шушарина — руководство и редактирование статьи.