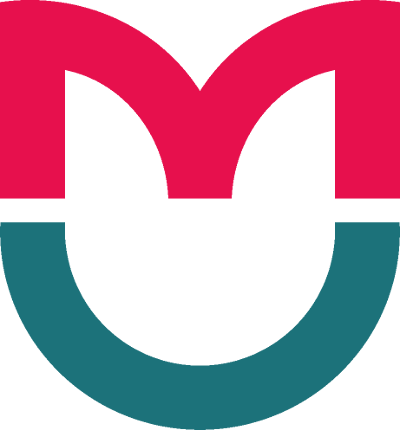
This article is an open access article distributed under the terms and conditions of the Creative Commons Attribution license (CC BY).
ORIGINAL RESEARCH
Electroencephalogram-based emotion recognition using a convolutional neural network
Immanuel Kant Baltic Federal University, Kaliningrad, Russia
Author contribution: Savinov VB, Botman SA, Sapunov VV, Petrov VA — data acquisition and processing, manuscript preparation; Samusev IG — manuscript preparation and revision; Shusharina NN — project supervision and manuscript revision.
The existing emotion recognition techniques based on the analysis of the tone of voice or facial expressions do not possess sufficient specificity and accuracy. These parameters can be significantly improved by employing physiological signals that escape the filters of human consciousness. The aim of this work was to carry out an EEG-based binary classification of emotional valence using a convolutional neural network and to compare its performance to that of a random forest algorithm. A healthy 30-year old male was recruited for the experiment. The experiment included 10 two-hour-long sessions of watching videos that the participant had selected according to his personal preferences. During the sessions, an electroencephalogram was recorded. Then, the signal was cleared of artifacts, segmented and fed to the model. Using a neural network, we were able to achieve a F1 score of 87%, which is significantly higher than the F1 score for a random forest model (67%). The results of our experiment suggest that convolutional neural networks in general and the proposed architecture in particular hold great promise for emotion recognition based on electrophysiological signals. Further refinement of the proposed approach may involve optimization of the network architecture to include more classes of emotions and improvement of the network’s generalization capacity when working with a large number of participants.
Keywords: electroencephalogram, machine learning, artificial neural network, emotional state, valence, deep learning, convolutional networks