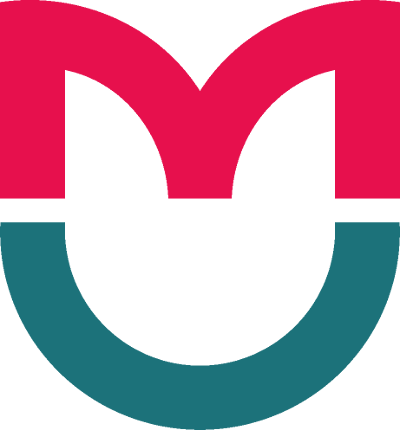
Статья размещена в открытом доступе и распространяется на условиях лицензии Creative Commons Attribution (CC BY).
ОРИГИНАЛЬНОЕ ИССЛЕДОВАНИЕ
Прогнозирование исходов программ экстракорпорального оплодотворения с использованием модели машинного обучения «Случайный лес»
1 Национальный исследовательский университет «Высшая школа экономики», Москва, Россия
2 Национальный медицинский исследовательский центр акушерства, гинекологии и перинатологии имени В. И. Кулакова, Москва, Россия
Для корреспонденции: Аюна Эрдэмовна Дашиева
ул. Академика Опарина, д. 4Б, г. Москва, 117198, Россия, ur.liam@aveihsad.rd
Вклад авторов: Г. М. Владимирский — обучение прогностических моделей, анализ литературы, выбор методов исследования; М. А. Журавлева — предобработка и анализ данных, анализ литературы, написание рукописи; А. Э. Дашиева — обработка исходного материала, анализ результатов; И. Е. Корнеева, Т. А. Назаренко — разработка анкеты для базы данных, редактирование рукописи.
- Корсак В. С., Смирнова А. А., Шурыгина О. В. Регистр ВРТ Общероссийской общественной организации «Российская ассоциация репродукции человека». Отчет за 2020 год. Проблемы репродукции. 2022; 28 (6): 12–27. DOI: 10.17116/ repro20222806112.
- Hassan MR, Al-Insaif S, Hossain MI, Kamruzzaman J. A machine learning approach for prediction of pregnancy outcome following IVF treatment. Neural Computing and Applications. 2018; 32: 2283–97.
- Ratna MB, Bhattacharya S, Abdulrahim B, McLernon DJ. A systematic review of the quality of clinical prediction models in in vitro fertilisation. Human Reproduction. 2020; 35: 100–116.
- Lintsen A, Eijkemans M, Hunault C, Bouwmans C, Hakkaart L, Habbema J, et al. Predicting ongoing pregnancy chances after IVF and ICSI: A national prospective study. Human Reproduction. 2007; 22: 2455–62.
- van Weert JM, Repping S, van der Steeg JW, Steures P, van der Veen F, Mol BW. A prediction model for ongoing pregnancy after in vitro fertilization in couples with male subfertility. J Reprod Med. 2008; 53 (4): 250–6. PMID: 18472647.
- Jones CA, Christensen AL, Salihu H, Carpenter W, Petrozzino J, Abrams E, et al. Prediction of individual probabilities of livebirth and multiple birth events following in vitro fertilization (IVF): A new outcomes counselling tool for IVF providers and patients using HFEA metrics. Journal of experimental clinical assisted reproduction. 2011; 8: 3.
- Nelson SM, Lawlor DA. Predicting live birth, preterm delivery, and low birth weight in infants born from in vitro fertilisation: A prospective study of 144,018 treatment cycles. PLoS Medicine. 2011; 8: e1000386.
- van Loendersloot LL, van Wely M, Repping S, Bossuyt PMM, van der Veen F. Individualized decision-making in IVF: Calculating the chances of pregnancy. Human Reproduction. 2013; 28: 2972–80
- Dhillon R, McLernon D, Smith P, Fishel S, Dowell K, Deeks J, et al. Predicting the chance of live birth for women undergoing IVF: A novel pretreatment counselling tool. Human Reproduction. 2015; 31: 84–92.
- McLernon DJ, Steyerberg EW, te Velde ER, Lee AJ, Bhattacharya S. Predicting the chances of a live birth after one or more complete cycles of in vitro fertilisation: Population based study of linked cycle data from 113 873 women. BMJ. 2016; p. i5735.
- McLernon DJ, Raja EA, Toner JP, Baker VL, Doody KJ, Seifer DB. Predicting personalized cumulative live birth following in vitro fertilization. Fertility and Sterility. 2022; 117 (2): 326–38.
- Vaegter KK, Lakic TG, Olovsson M, Berglund L, Brodin T, Holte J. Which factors are most predictive for live birth after in vitro fertilization and intracytoplasmic sperm injection (IVF/ICSI) treatments? Analysis of 100 prospectively recorded variables in 8,400 IVF/ICSI single-embryo transfers. Fertility and Sterility. 2017; 107 (3): 641–48.
- Ottosen LDM, Kesmodel U, Hindkjaer J, Ingerslev HJ. Pregnancy prediction models and eSET criteria for IVF patients–do we need more information? Journal of assisted reproduction and genetics. 2007; 24 (1): 29–36.
- Xu T, d. F. Veiga A, Hammer KC, Paschalidis ICh, Mahalingaiah Sh. Informative predictors of pregnancy after first IVF cycle using eIVF practice highway electronic health records. Scientific Reports. 2022; 12: 1–9.
- C N Barreto N, Castro GZ, Pereira RG, Pereira FAN, Reis FM, Junior WM, et al. Predicting in vitro fertilization success in the Brazilian public health system: a machine learning approach. Med Biol Eng Comput. 2022; 60 (7): 1851–61. DOI: 10.1007/s11517-022-02569-1. Epub 2022 May 4. PMID: 35508786.
- Barnett-Itzhaki Z, Elbaz M, Butterman R, Amar D, Amitay M, Racowsky C, et al. Machine learning vs. classic statistics for the prediction of IVF outcomes. Journal of Assisted Reproduction and Genetics. 2020; 37: 2405–12.
- Lundberg S, Lee S-I. A unified approach to interpreting model predictions. Available from: https://arxiv.org/abs/1705.07874, may 22 2017.
- Morris JA, Gardner MJ. Statistics in Medicine: Calculating confidence intervals for relative risks (odds ratios) and standardised ratios and rates. BMJ. 1988; 296: 1313–6.
- Sheskin DJ. Handbook of parametric and nonparametric statistical procedures. Chapman and Hall/CRC, 2003.
- Breiman L, Friedman JH, Olshen RA, Stone CJ. Classification and regression trees. Routledge, 2017.
- Cournapeau D, Brucher M, Perrot M, Duchesnay E. Scikitlearn: Machine learning in Python. Journal of Machine Learning Research. 2011; 12: 2825–30.
- Prokhorenkova L, Gusev G, Vorobev A, Dorogush AV, Gulin A. Catboost: Unbiased boosting with categorical features. 2017. Available from: https://arxiv.org/abs/1706.09516.