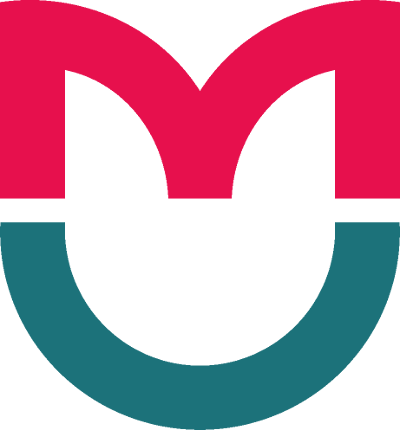
ORIGINAL RESEARCH
Brain-computer-interface technology with multisensory feedback for controlled ideomotor training in the rehabilitation of stroke patients
1 Research Center of Cerebrovascular Pathology and Stroke, Ministry of Health of the Russian Federation, Moscow, Russia
2 Pirogov Russian National Research Medical University, Moscow, Russia
3 Institute of Higher Nervous Activity and Neurophysiology, Russian Academy of Sciences, Moscow, Russia
Correspondence should be addressed: Yuliya V. Bushkova
Ostrovityanova, 1, str. 10, Moscow, 117342; moc.liamg@777hsubajiluJ
Funding: the study was supported by the Russian Foundation for Basic Research (Grant ID 16-29-08247 ofi_m).
Author contribution: Bushkova YuV — study planning; literature analysis; data acquisition, analysis and interpretation; manuscript preparation; Ivanova GE — study planning; literature analysis; data interpretation; manuscript preparation; Stakhovskaya LV — study planning; data interpretation; manuscript preparation; Frolov AA — manuscript preparation.
Stroke is one of the leading causes of lasting disabilities worldwide. Post-stroke motor and cognitive deficits impair patients’ mobility and everyday activities, prevent their reintegration in the community and reduce their chances to return to work [1, 2].
A well-structured, gradual, specific, and adequate neurorehabilitation (NR) program can significantly mitigate stroke-related neurological deficit.
NR relies on the reserve capacity of the brain determined by the systemic organization of brain functions. As the patient relearns movement skills, their brain undergoes functional reorganization resulting in the restoration of or compensation for stroke-damaged functions. The ultimate goal of NR is true recovery. This sounds like a very adequate approach since it implies complete restoration of the affected function or the best improvement possible. The affected functions are regained due to the reorganization of intact functional systems enabled by the plasticity of the nervous system and the anatomic connections between its compartments.
Rehabilitation of neurology patients boasts a variety of methods aimed at initiating plasticity processes that make up for or replace the lost function. However, the majority of patients experience limited recovery after stroke. Advances in brain-computer interface (BCI) technology for activating the motor cortex that controls a specific movement, such as a BCI-controlled robotic hand, have substantially improved the quality of post-stroke rehabilitation [3, 4].
A BCI is directly operated by neurophysiological activity of the brain in such a way that neuromuscular pathways typically involved in the motion are bypassed [5]. A BCI-controlled robotic hand with visual and kinesthetic feedback harnesses the ability of patients to generate various EEG signals (the EEG μ-rhythm in the motor regions of the brain, in our case), allowing the brain to “connect” to external devices without involving neuromuscular pathways. The BCI rehabilitation device relies on the mental imagery (MI) of active movements. According to functional neuroimaging studies, MI activates cortical motor areas [6], except when stroke disrupts the ability of the brain to generate mental images [7].
It is known that repeated mental rehearsal of an action (ideomotor training, IT) promotes development of a motor skill under physiological conditions and recovery or regain of an impaired/lost skill in patients with nervous system pathology. An ideomotor task means a physical movement is performed mentally. This is also known as mental practice. The patient is asked to imagine a movement (such as reaching out with their hand, opening a hand, clenching a fist, grasping a cup, etc.) from a first- or third-person perspective. If the movement is imagined from the first-person perspective, the patient is very likely to perform it kinesthetically. If the task is performed from the third-person perspective, this type of imagery is visual [8]. In comparison with visual imagery, kinesthetic imagery is more reliably associated with a patient’s ability to successfully interact with brain-computer interfaces [9].
There are a few major hypotheses about the mechanism underlying the effect of mental practice. One of them, the so-called psychoneuromuscular theory [10], is based on the assumption that mental practice activates muscles involved in performing the movement at the subthreshold level and consolidates the motor “script”. According to another hypothesis, motor imagery evokes specific neurophysiological patterns in the motor cortex; these patterns are similar to those involved in implementing the actual movement. Functional MRI studies suggest that mental practice leads to the reorganization of the motor system in both brain hemispheres [6, 11].
Various dosage regimens of ideomotor training have been tested in randomized clinical trials. A statistically significant effect on the FMA and ARAT scales has been demonstrated for the following regimen: two 30-min sessions once a week repeated over the course of 6 weeks [12]. The program included functionally significant movements like reaching out or grasping an object, using writing utensils, etc.
However, the use of IT for regaining control of movement in adults after a catastrophic cerebral event produces controversial results [13]. Damage to motor function negatively affects both the patient’s ability to execute movements and to imagine them. This could be due to post-stroke cognitive impairment [1, 14].
Rehabilitation technologies based on motor imagery hold promise for patients with cerebral injury [12]. IT is seen as an adjunct to conventional rehabilitation. IT combined with physical therapy for patients with neurological deficits shapes the basis for repetitive task-specific practice (RTP). It has been shown that prolonged regular motor imagery training has a positive gradual and sustainable effect on neuronal plasticity [15].
Motor imagery is a subjective process and, therefore, is difficult to evaluate. One of the solutions lies in measuring desynchronization of sensorimotor EEG rhythms induced by motor imagery [4, 16]. Desynchronization of sensorimotor EEG rhythms can be used to detect the act of motor imagery, but its specificity remains understudied.
Another problem addressed in the literature is assessing a patient’s ability for motor imagery [17]. This can be done using the movement imagery questionnaire (MIQ) adapted for the clinical population. MIQ and MIQ-R are normally used in athletes, whereas MIQ-RS, in individuals with motor deficit.
The BCI controlled robotic hand rehabilitation technology with multisensory visual and kinesthetic feedback can be described as controlled ideomotor training. This training is based on the enhancement of afferent stimulation of the upper limb by the mechanical force of the exoskeleton in response to successful performance of a mental task.
Thus, one of the crucial problems facing mental imagery research is detection of patients’ ability to implement mental tasks because it determines the efficacy of interaction between the patient and the rehabilitation device.
The aim of this study was to explore the factors affecting motor recovery of the upper limb in stroke patients undergoing rehabilitation with a BCI-controlled robotic hand in the early rehabilitation period.
METHODS
The study was carried out by the Research Center of Cerebrovascular Pathology (Pirogov Russian National Research Medical University) at the facilities of Moscow City Clinical Hospital № 31 (Neurology Unit for stroke patients) from September 2018 to April 2019.
Inclusion criteria were as follows: patients of both sexes aged 18–80 years with a history of one subcortical stroke experienced < 2 years before the study, retained cognitive function (at least 22 points on the Montreal Cognitive Assessment scale, МоСА) [18], motor deficit of the upper limb (0 to 4 points on the Medical Research Council Scale for Muscle Strength) [19], and right-handedness according to the Edinburgh Handedness Inventory [20].
The following exclusion criteria were applied: older stroke (> 2 years before the study); left-handedness (the Edinburgh Handedness Inventory); pronounced reduction in cognitive function; sensory aphasia; severe motor aphasia; severe visual impairment that prevented the patient from following visual instructions on the computer screen; excessive arm spasticity (4 points on the Ashworth scale, mAS) [21].
We analyzed performance of 24 patients: 14 (58.3%) men and 10 (41.7%) women. The median age was 56.5 (51.0; 62.0) years. Stroke was ischemic in 20 patients (83.3%) and hemorrhagic in 4 (16.7%) patients. In all the participants, stroke was localized to the supratentorial brain region (the location was confirmed by CT or MRI). The lesion was left-hemispheric in 11 (45.6%) patients and right-hemispheric in 13 (54.4%) patients. The median time elapsed from stroke was 4 months (3.0; 12.0). The МоСА median was 25.0 points (23.0; 27.0), corresponding to moderate cognitive deficit. Some of the participants were in inpatient care (n = 11); others were outpatients (n = 13). The BCIHE-based rehabilitation consisted of 9.5 sessions (8.0; 10.0).
The device used for rehabilitation was a brain-computer interface-controlled hand exoskeleton with kinesthetic and visual feedback (Exohand-2) developed at Pirogov Russian National Research Medical University, Moscow (figure)
BCI relies on the analysis of EEG patterns and recognition of sensorimotor μ-rhythm synchronization/desynchronization during hand motor imagery. EEG signals were bandpass-filtered at 5–30 Hz. The Bayesian classifier used in our study is described in [22]. Classification accuracy was measured using Cohen’s kappa coefficient (perfect recognition accuracy: κ = 1, random chance: κ = 0 [23]) and the percentage of correct responses of the classifier (the recognition rate > 33% indicated above random recognition accuracy because the patients had a definite instruction to follow).
Recognition results were presented to the patient through visual and kinesthetic feedback: if the classifier recognized the motor imagery task given in the instruction, the cursor in the middle of the screen changed its color to green and the exoskeleton performed a hand opening movement. If other tasks were recognized, the cursor did not change its color and the exoskeleton did not produce any movement [4]. In essence, this type of therapy is controlled ideomotor training utilizing the principles of multichannel (visual, kinesthetic, EEG) biofeedback.
During the session, the patient was wearing a cap with EEG electrodes. Conductive gel was applied to the electrode surface. The electromechanically powered hand exoskeleton for hand opening was attached to both hands of the patient. The patient was sitting in the armchair in front of the computer screen. In the middle of the dark screen there was a circle for gaze fixation; three arrows outside the circle represented different instructions. A change of arrow color indicated task presentation. The patient performed one of 3 mental tasks: motor relaxation, kinesthetic imagery of slow right hand or left hand opening. Hand opening commands (the arrows in the right and left sections of the screen changed their colors accordingly) were presented in random order for 10 seconds. Mental imagery tasks were alternated with a task to relax indicated by a flash of the upper arrow; during the resting task, the patient had to sit for 10 s and look at the center of the screen. A session consisted of up to 3 trials, each lasting for 10 minutes. The patient rested for at least 3 min between the trials. The rehabilitation course lasted for 14–18 days; the interval between the sessions could extend up to 2 days.
RESULTS
The participants took the motor rehabilitation course consisting of standard physical therapy for post-stroke patients (kinesiotherapy, PNF, Мotomed movement trainer) [24] and 9.5 sessions (8.0; 10.0) of BCI-controlled robotic hand -based training.
Motor activity of the upper limb was assessed using the following scales: the Fugl-Meyer Assessment (FMA) [25], the Medical Research Council Scale for Muscle Strength (MRCMS), the modified Ashworth scale (mAS) for spasticity. Functional activity of the upper limb was assessed using the Action Research Arm Test (ARAT) [26]. The Barhtel Index (BI) for Activities of Daily Living was applied to understand the level of patients’ functional independence. Motor imagery performance was evaluated using the Movement Imagery Questionnaire (MIQ-RS) for clinical populations.
Statistical analysis was carried out in Statistica ver. 13.0 (StatSoft; USA) using the Mann-Whitney U and Wilcoxon tests and Spearman’s correlation coefficient. In this article, the data are presented as medians and interquartile ranges (25; 75%) and differences are considered significant at p < 0.05.
The median efficacy of patient-BCI interaction (Cohen’s kappa, the recognition rate) was 58.5% (45.7; 62.6) (р < 0.05), suggesting that such interaction was successful.
In order to study the ability of patients for kinesthetic motor imagery based on the MIQ-RS score, we divided them into two groups depending on stroke lateralization (tab. 1).
The obtained differences were statistically significant. Patients with left-hemispheric lesions did better in generating kinesthetic motor images than patients with right-hemispheric lesions; these findings are consistent with the literature [27].
However, the attempt to establish a correlation between motor imagery performance in patients with different stroke localization and the efficacy of patient-BCI interaction using the classifier output was unsuccessful. So we distributed the patients’ data into 2 groups depending on the quality of motor imagery inferred from the MIQ-RS total scores. The first group comprised patients who scored over 50% of the maximum score on the subscale representing the kinesthetic component; the second group consisted of patients who scored less than 50%. Thirteen patients perceived their ability for kinesthetic imagery as quite good: the median score was 47.5 (32.0; 54.0) points. For the rest 11 patients, the median score was 27.0 (15.0; 29.0) points. The difference between the groups was statistically significant (p < 0.05). In the next step, we investigated the association between the quality of motor imagery and the efficacy of patient-BCI interaction (tab. 2).
The analysis of the obtained data revealed a significant moderate correlation between the quality of motor imagery (MIQ-RS) and the efficacy of patient-BCI interaction. In other words, the accuracy of the classifier was higher for patients with high MIQ-RS scores; patients with lower MIQ-RS scores interacted with the exoskeleton much less effectively.
The relationship between motor recovery/functional improvement of the upper limb and the efficacy of interaction with the exoskeleton is shown in tab. 3.
In both groups, the dynamics were positive and statistically significant in terms of motor recovery and functional improvement of the upper limb. The dynamics were more pronounced in patients with a better ability for kinesthetic imagery; however, the difference between the groups was insignificant. Besides, we failed to establish a significant correlation between the efficacy of patient-BCI interaction (the classifier output) and the dynamics of motor recovery of the upper limb, which might be explained by the small sample size. Improved BI scores observed for both groups were due to the movements that involved both hands.
DISCUSSION
Noninvasive neurointerfaces decode brain activity in real time and thus allow users to manipulate external devices. In noninvasive approaches, the user performs a cognitive task while their brain signals are recorded by EEG and decoded in real time to execute control over the external device [28]. In spite of technical breakthroughs, the ability to effectively interact with BCI is limited for some healthy individuals. It has been shown that as many as 15 to 30% of users cannot execute effective control [29]. Studies carried out in larger populations demonstrate that up to 50% of individuals cannot achieve accuracy above 70% — the threshold indicating successful patient-BCI interaction [30]. Importantly, these parameters reflect the situation in the general population. Our study recruited neurology patients with moderate cognitive deficit. Of them, some patients performed better: Cohen’s kappa and the recognition rate were 63.0% (54.0; 67.0) vs 39.0% (32.0; 48.0) in other patients. These data correlated with the MIQ-RS scores. Our findings have important theoretical and practical implications as they prove the unity of cognitive and motor components in the human body, demonstrates the need for a dualistic approach to the recovery of motor and cognitive functions in patients with brain injury, sets the goal of developing a personalized approach to applying the BCI technology for better clinical outcomes, which will result in a more rational use of rehabilitation resources.
CONCLUSION
This study has established a correlation between the ability of patients for motor imagery inferred from the MIQ-RS scale and the efficacy of patient-BCI interaction. A larger patient sample is needed to assess the effect of patient-BCI interaction on the dynamics of upper limb motor recovery dynamics.