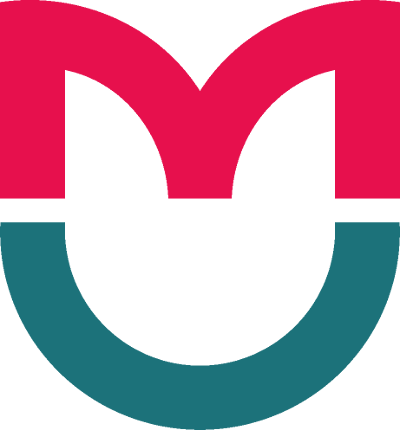
This article is an open access article distributed under the terms and conditions of the Creative Commons Attribution license (CC BY).
ORIGINAL RESEARCH
Predicting the outcomes of in vitro fertilization programs using a random forest machine learning model
1 Higher School of Economics National Research University, Moscow, Russia
2 Kulakov National Medical Scientific Centre for Obstetrics, Gynecology and Perinatal Medicine, Moscow, Russia
Correspondence should be addressed: Ayuna E. Dashieva
Akademika Oparina, 4B, Moscow, 117198, Russia, ur.liam@aveihsad.rd
Author contribution: Vladimirsky GM — predictive models training, literature analysis, choice of research methods; Zhuravleva MA — preprocessing and analysis of data, literature analysis, manuscript authoring; Dashieva AE — processing of source material, analysis of results; Korneeva IE, Nazarenko TA — development of the survey for the database, manuscript editing.
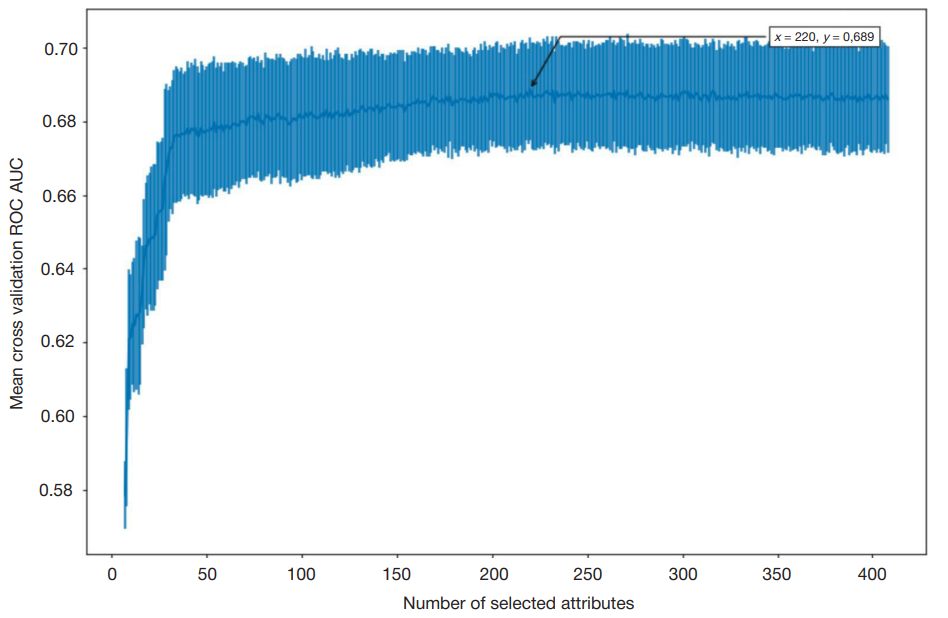
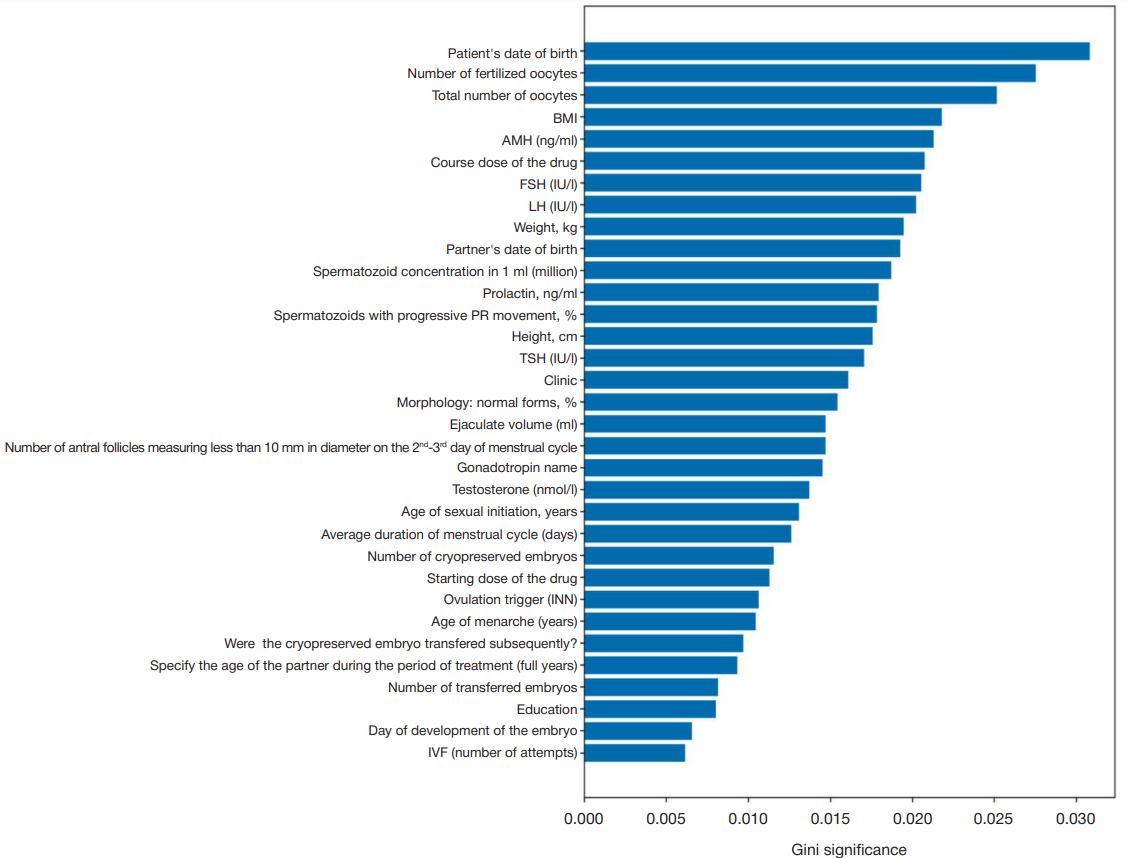