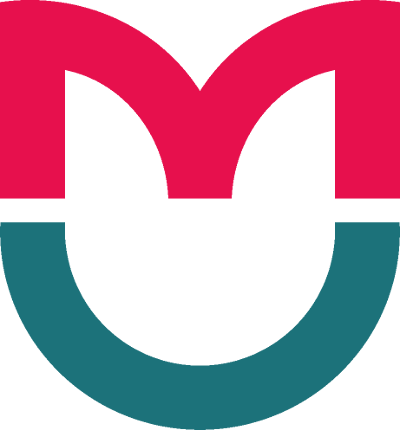
МНЕНИЕ
Глубокое обучение в моделировании белок-лигандного взаимодействия: новые пути в разработке лекарственных препаратов
1 Институт биоорганической химии имени М. М. Шемякина и Ю. А. Овчинникова Российской академии наук, Москва, Россия
2 Московский физико-технический институт, Долгопрудный, Россия
3 Российский национальный исследовательский медицинский университет имени Н. И. Пирогова, Москва, Россия
Для корреспонденции: Зинаида Михайловна Осипова
ул. Миклухо-Маклая, 16/10, г. Москва, 117997, Россия; ur.hcbi@avoksakz
Финансирование: исследование выполнено за счет гранта Российского научного фонда № 22-44-02024, https://rscf.ru/project/22-44-02024/.
Вклад авторов: А. Д. Барыкин — анализ литературы, написание рукописи, Т. В. Чепурных — идея, анализ литературы, написание и редактирование рукописи, З. М. Осипова — руководство проектом, редактирование рукописи.
- Lazo JS. Rear-view mirrors and crystal balls: a brief reflection on drug discovery. Mol Interv. 2008; 8 (2): 60–3. DOI: 10.1124/mi.8.2.1.
- Blanes-Mira C, Fernández-Aguado P, de Andrés-López J, Fernández-Carvajal A, Ferrer-Montiel A, Fernández-Ballester G. Comprehensive survey of consensus docking for highthroughput virtual screening. Molecules. 2023; 28 (1): 175. DOI: 10.3390/molecules28010175.
- Zhao L, Zhu Y, Wang J, Wen N, Wang C, Cheng L. A brief review of protein–ligand interaction prediction. Comput Struct Biotechnol J. 2022; 20: 2831–8. DOI: 10.1016/j.csbj.2022.06.004.
- Pagadala NS, Syed K, Tuszynski J. Software for molecular docking: a review. Biophys Rev. 2017; 9: 91–102. DOI: 10.1007/s12551-016-0247-1. PubMed PMID: 28510083.
- Wang Z, Sun H, Yao X, Li D, Xu L, Li Y, et al. Comprehensive evaluation of ten docking programs on a diverse set of protein– ligand complexes: the prediction accuracy of sampling power and scoring power. Phys Chem Chem Phys. 2016; 18: 12964–75. DOI: 10.1039/c6cp01555g.
- Perez JJ, Perez RA, Perez A. Computational modeling as a tool to investigate PPI: from drug design to tissue engineering. Front Mol Biosci. 2021; 8: 681617. DOI: 10.3389/fmolb.2021.681617. PubMed PMID: 34095231.
- Santos LHS, Ferreira RS, Caffarena ER. Integrating molecular docking and molecular dynamics simulations. Methods Mol Biol. 2019; 2053: 13–34. DOI: 10.3389/fmolb.2021.681617.
- Jumper J, Evans R, Pritzel A, Green T, Figurnov M, Ronneberger O, et al. Highly accurate protein structure prediction with AlphaFold. Nature. 2021; 596: 583–9. DOI: 10.1038/s41586-021-03819-2.
- Crampon K, Giorkallos A, Deldossi M, Baud S, Steffenel LA. Machine-learning methods for ligand–protein molecular docking. Drug Discov Today. 2021; 27(1): 151–64. DOI: 10.1016/j.drudis.2021.09.007.
- Jiménez J, Doerr S, Martínez-Rosell G, Rose AS, De Fabritiis G. DeepSite: protein-binding site predictor using 3D-convolutional neural networks. Bioinformatics. 2017; 33 (19): 3036–42. DOI: 10.1093/bioinformatics/btx350.
- Kandel J, Tayara H, Chong KT. PUResNet: prediction of proteinligand binding sites using deep residual neural network. J Cheminform. 2021; 13: 65. DOI: 10.1186/s13321-021-00547-7.
- Greener JG, Kandathil SM, Moffat L, Jones DT. A guide to machine learning for biologists. Nat Rev Mol Cell Biol. 2022; 23: 40–55. DOI: 10.1038/s41580-021-00407-0.
- Wang Y, Jiao Q, Wang J, Cai X, Zhao W, Cui X. Prediction of proteinligand binding affinity with deep learning. Comput Struct Biotechnol J. 2023; 21: 5796–806. DOI: 10.1016/j.csbj.2023.11.009.
- Zhao L, Zhu Y, Wang J, Wen N, Wang C, Cheng L. A brief review of protein–ligand interaction prediction. Comput Struct Biotechnol J. 2022; 20: 2831–8. DOI: 10.1016/j.csbj.2022.06.004.
- Stokes JM, Yang K,Swanson K, Jin W, Cubillos-Ruiz A, Donghia NM, et al. A deep learning approach to antibiotic discovery. Cell. 2020; 180: P688–702.E13. DOI: 10.1016/j.cell.2020.01.021.
- Arnold C. Inside the nascent industry of AI-designed drugs. Nat Med. 2023; 29: 1292–5. DOI: 10.1038/s41591-023-02361-0.
- Yang B, Li K, Zhong X, Zou J. Implementation of deep learning in drug design. MedComm — Fut Med. 2022; 1: e18. DOI: 10.1002/mef2.18.