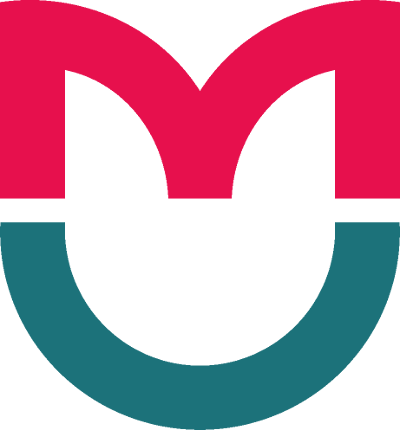
This article is an open access article distributed under the terms and conditions of the Creative Commons Attribution license (CC BY).
ORIGINAL RESEARCH
Determining optimal ambient ionization mass spectrometry data pre-processing parameters in neurosurgery
1 Moscow Institute of Physics and Technology, Moscow, Russia
2 Semenov Federal Research Center for Chemical Physics of the Russian Academy of Sciences, Moscow, Russia
3 Skolkovo Institute of Science and Technology, Moscow, Russia
4 Siberian State Medical University, Tomsk, Russia
Correspondence should be addressed: Denis S. Zavorotnyuk
Institutskiy per., 9, str. 7, Dolgoprudny, Moscow Region, 141701; moc.liamg@kuyntorovaz.sined
Funding: the study was performed within the framework of the state assignment of the Ministry of Science and Higher Education of the Russian Federation (agreement № 075-03-2022-107, project № 0714-2020-0006). The study involved the use of equipment of the Semenov Federal Research Center for Chemical Physics RAS.
Author contribution: Zavorotnyuk DS — data acquisition and interpretation, software development, manuscript writing and editing; Sorokin AA — study planning, data analysis and interpretation, manuscript editing; Bormotov DS — data acquisition and interpretation, manuscript writing; Eliferov VA — financial support of the experiment; Bocharov KV — data acquisition; Pekov SI — study planning, data analysis and interpretation, manuscript draft writing and manuscript text finalization; Popov IA — project management, financial support.
Compliance with ethical standards: the study was approved by the Ethics Committee of the Burdenko Research Institute of Neurosurgery (protocols № 40 dated 12 April 2016 and № 131 dated 17 July 2018) and conducted in accordance with the principles of the Declaration of Helsinki (2000) and its subsequent revisions. All patients submitted the informed consent to study participation and the use of biomaterial for scientific purposes.
- Young RM, Jamshidi A, Davis G, Sherman JH. Current trends in the surgical management and treatment of adult glioblastoma. Ann Transl Med 2015: 1–15. https://doi.org/10.3978/j.issn.2305-5839.2015.05.10.
- Chanbour H, Chotai S. Review of intraoperative adjuncts for maximal safe resection of gliomas and its impact on outcomes. Cancers. 2022; 14: 5705. Available from: https://doi.org/10.3390/cancers14225705.
- Pekov SI, Bormotov DS, Nikitin PV, Sorokin AA, Shurkhay VA, Eliferov VA, et al. Rapid estimation of tumor cell percentage in brain tissue biopsy samples using inline cartridge extraction mass spectrometry. Anal Bioanal Chem. 2021; 413: 2913–22. Available from: https://doi.org/10.1007/s00216-021-03220-y.
- Eberlin LS, Norton I, Orringer D, Dunn IF, Liu X, Ide JL, et al. Ambient mass spectrometry for the intraoperative molecular diagnosis of human brain tumors. Proc Natl Acad Sci. 2013; 110: 1611–6. Available from: https://doi.org/10.1073/pnas.1215687110.
- Hänel L, Kwiatkowski M, Heikaus L, Schlüter H. Mass spectrometrybased intraoperative tumor diagnostics. Future Sci OA. 2019; 5: FSO373. Available from: https://doi.org/10.4155/fsoa-2018-0087.
- Li L-H, Hsieh H-Y, Hsu C-C. Clinical application of ambient ionization mass spectrometry. Mass Spectrom. 2017; 6: S0060–S0060. Available from: https://doi.org/10.5702/massspectrometry.S0060.
- Huang M-Z, Yuan C-H, Cheng S-C, Cho Y-T, Shiea J. Ambient ionization mass spectrometry. Annu Rev Anal Chem. 2010; 3: 43–65. Available from: https://doi.org/10.1146/annurev.anchem.111808.073702.
- Shi L, Habib A, Bi L, Hong H, Begum R, Wen L. Ambient Ionization Mass Spectrometry: Application and Prospective. Crit Rev Anal Chem. 2022: 1–50. Available from: https://doi.org/10.1080/10408347.2022.2124840.
- Boiko DA, Kozlov KS, Burykina JV, Ilyushenkova VV, Ananikov VP. Fully automated unconstrained analysis of high-pesolution mass spectrometry data with nachine learning. J Am Chem Soc. 2022; 144: 14590–606. Available from: https://doi.org/10.1021/jacs.2c03631.
- Liebal UW, Phan ANT, Sudhakar M, Raman K, Blank LM. Machine learning applications for mass spectrometry-based metabolomics. Metabolites. 2020; 10: 1–23. Available from: https://doi.org/10.3390/metabo10060243.
- Piras C, Hale OJ, Reynolds CK, Jones AK (Barney), Taylor N, Morris M, et al. LAP-MALDI MS coupled with machine learning: an ambient mass spectrometry approach for high-throughput diagnostics. Chem Sci. 2022; 13: 1746–58. Available from: https://doi.org/10.1039/D1SC05171G.
- Seddiki K, Saudemont P, Precioso F, Ogrinc N, Wisztorski M, Salzet M, et al. Cumulative learning enables convolutional neural network representations for small mass spectrometry data classification. Nat Commun. 2020; 11. Available from: https://doi.org/10.1038/s41467-020-19354-z.
- Huang YC, Chung HH, Dutkiewicz EP, Chen CL, Hsieh HY, Chen BR, et al. Predicting breast cancer by paper spray ion mobility spectrometry mass spectrometry and machine learning. Anal Chem. 2020; 92: 1653–7. Available from: https://doi.org/10.1021/acs.analchem.9b03966.
- Iwano T, Yoshimura K, Inoue S, Odate T, Ogata K, Funatsu S, et al. Breast cancer diagnosis based on lipid profiling by probe electrospray ionization mass spectrometry. Br J Surg. 2020; 107: 632–5. Available from: https://doi.org/10.1002/bjs.11613.
- Zhou M, Guan W, Walker LDE, Mezencev R, Benigno BB, Gray A, et al. Rapid mass spectrometric metabolic profiling of blood sera detects ovarian cancer with high accuracy. Cancer Epidemiol Biomarkers Prev. 2010; 19: 2262–71. Available from: https://doi.org/10.1158/1055-9965.EPI-10-0126.
- Torp SH, Solheim O, Skjulsvik AJ. The WHO 2021 Classification of central nervous system tumours: a practical update on what neurosurgeons need to know — a minireview. Acta Neurochir (Wien). 2022; 164: 2453–64. Available from: https://doi.org/10.1007/s00701-022-05301-y.
- Bormotov DS, Eliferov VA, Peregudova OV, Zavorotnyuk DS, Bocharov KV, Pekov SI, et al. Incorporation of a disposable ESI emitter into inline cartridge extraction mass spectrometry improves throughput and spectra stability. J Am Soc Mass Spectrom. 2023; 34: 119–22. Available from: https://doi.org/10.1021/jasms.2c00207.
- Gibb S, Strimmer K. Maldiquant: A versatile R package for the analysis of mass spectrometry data. Bioinformatics. 2012; 28. Available from: https://doi.org/10.1093/bioinformatics/bts447.
- Kuhn M. Building predictive models in R using the caret package. J Stat Softw. 2008; 28: 1–26. Available from: https://doi.org/10.18637/jss.v028.i05.
- Friedman JH, Hastie T, Tibshirani R. Regularization paths for generalized linear models via coordinate descent. J Stat Softw. 2010; 33: 1–22. Available from: https://doi.org/10.18637/jss.v033.i01.
- Wickham H. ggplot2: Elegant graphics for data analysis. SpringerVerlag, New York; 2016.
- Rew R, Davis G, Emmerson S, Cormack C, Caron J, Pincus R, et al. Unidata NetCDF 1989. Available from: https://doi.org/10.5065/D6H70CW6.
- Zavorotnyuk DS, Pekov SI, Sorokin AA, Bormotov DS, Levin N, Zhvansky E, et al. Lipid profiles of human brain tumors obtained by high-resolution negative mode ambient mass spectrometry. Data. 2021; 6: 1–7. Available from: https://doi.org/10.3390/data6120132.
- Eberlin LS, Norton I, Dill AL, Golby AJ, Ligon KL, Santagata S, et al. Classifying human brain tumors by lipid imaging with mass spectrometry. Cancer Research. 2012; 72 (3): 645–54. Available from: https://doi.org/10.1158/0008-5472.can-11-2465.
- Friedman JH. Smart user’s guide. Stanford Univ CA, Laboratory for Computational Statistics; 1984.
- Morris JS, Coombes KR, Koomen J, Baggerly KA, Kobayashi R. Feature extraction and quantification for mass spectrometry in biomedical applications using the mean spectrum. Bioinformatics. 2005; 21: 1764– 75. Available from: https://doi.org/10.1093/bioinformatics/bti254.
- Burnham KP, Anderson DR, editors. Model Selection and Multimodel Inference. New York, NY: Springer New York, 2004. Available from: https://doi.org/10.1007/b97636.
- Gustafsson F, Hjalmarsson H. Twenty-one ML estimators for model selection. Automatica. 1995; 31: 1377–92. Available from: https://doi.org/10.1016/0005-1098(95)00058-5.
- Shitikov VK, Mastitsky SE. Klassifikacija, regressija i drugie algoritmy Data Mining s ispol'zovaniem R. 2017. Dostupna po ssylke: https://github.com/ranalytics/data-mining. Russian.
- Akaike H. A new look at the statistical model identification. IEEE Trans Autom Control. 1974; 19: 716–23. Available from: https://doi.org/10.1109/TAC.1974.1100705.
- Zavorotnyuk DS. MS Spectrum observer repository. Available from: https://github.com/zdens/MS-Spectrum-Observer/releases/tag/1.0 (data obrashhenija: 29 fevralja 2024 g.).
- Zavorotnyuk DS. MS spectrum observer Demo. Available from: https://zdens.shinyapps.io/ms-spectrum-observer (data obrashhenija: 29 fevralja 2024 g.).