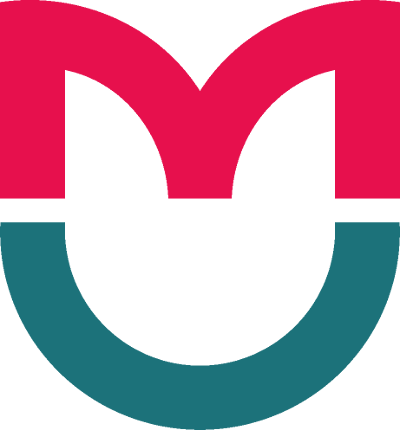
This article is an open access article distributed under the terms and conditions of the Creative Commons Attribution license (CC BY).
ORIGINAL RESEARCH
Development of a neurodevice with a biological feedback for compensating for lost motor functions
1 Institute of Chemistry and Biology,Immanuel Kant Baltic Federal University, Kaliningrad, Russia
2 Department of Hospital Surgery No. 1, Faculty of General Medicine,Pirogov Russian National Research Medical University, Moscow, Russia
3 Department of Human Pathology, Faculty of Postgraduate Professional Training of Physicians,The First Sechenov Moscow State Medical University, Moscow
Correspondence should be addressed: Evgeny Bogdanov
ul. A. Nevskogo, d. 14, Kaliningrad, Russia, 236041; moc.liamg@vonadgobue
Funding: the work was supported by the Ministry of Education and Science of the Russian Federation (Grant Agreement no. RFMEFI57815X0140 dated October 27, 2015).
Acknowledgements: the authors thank Alexandr Romanov of the Rehabilitation Center of the Administrative Department of the President of the Russian Federation, Moscow; Raphael Oganov of State Research Center for Preventive Medicine, Moscow; Daniil Borchevkin, Alexey Belousov, Vladimir Savinov, Sergey Sokolov, and Alexey Medvedev for their scientific contribution and productive collaboration.
- Singh RM, Chatterji S, Kumar A. Trends and challenges in EMG based control scheme of exoskeleton robots — a review. Int J Sci Eng Res. 2012; 3 (9): 933–40.
- Artemiadis P. EMG-based robot control interfaces: past, present and future. Adv Robot Autom. 2012, 1 (2): e107.
- Li G. Electromyography pattern-recognition-based control of powered multifunctional upper-limb prostheses. In: Mizrahi J, editor. Advances in applied electromyography. InTech; 2011. 224 p.
- Kim Y, Doh NL, Youm Y, Chung WK. Robust discrimination method of the electrooculogram signals for human-computer interaction controlling mobile robot. Intell Autom Soft Comp. 2007; 13 (3): 319–36.
- Oonishi Y, Oh S, Hori Y. New control method for power-assisted wheelchair based on upper extremity movement using surface myoelectric signal. 2008 10th IEEE International Workshop on Advanced Motion Control. 2008; 498–503.
- Gu Y, Dremstrup K, Farina D. Single-trial discrimination of type and speed of wrist movements from EEG recordings. Clin Neurophysiol. 2009 Aug; 120 (8): 1596–600.
- Shenoy P, Miller KJ, Crawford B, Rao RP. Online electromyographic control of a robotic prosthesis. IEEE Trans Biom Eng. 2008 Mar; 55 (3): 1128–35.
- Kiguchi K, Hayashi Y. An EMG-based control for an upper-limb power-assist exoskeleton robot. IEEE Trans Syst Man Cybern B Cybern. 2012 Aug; 42 (4): 1064–71.
- Rosen J, Brand M, Fuchs MB, Arcan М. A myosignal-based powered exoskeleton system. IEEE Trans Syst Man Cybern A Syst Hum. 2001; 31 (3): 210–22.
- Collinger JL, Wodlinger B, Downey JE, Wang W, Tyler-Kabara EC, Weber DJ, et al. High-performance neuroprosthetic control by an individual with tetraplegia. Lancet. 2013 Feb 16; 381 (9866): 557–64.
- Nunez PL, Srinivasan R. Electric fields of the brain: the neurophysics of EEG. Oxford, UK: Oxford university press; 2006. 611 р.
- Arai M, Nishinaka Y, Miki N. Long-term electroencephalogram measurement using polymer-based dry microneedle electrode. In: 2015 Transducers – 2015 18th International Conference on Solid-State Sensors, Actuators and Microsystems (TRANSDUCERS); 2015 June 21–25; Anchorage, AK. IEEE; 2015. p. 81–4.
- Agashe H, Contreras-Vidal JL. Reconstructing hand kinematics during reach to grasp movements from electroencephalographic signals. Conf Proc IEEE Eng Med Biol Soc. 2011; 2011: 5444–7. DOI: 10.1109/IEMBS.2011.6091389.
- Mohamed AK, Marwala T, John LR. Single-trial EEG discrimination between wrist and finger movement imagery and execution in a sensorimotor BCI. Conf Proc IEEE Eng Med Biol Soc. 2011; 2011: 6289–93.
- Yang J, Singh H, Hines EL, Schlaghecken F, Iliescu DD, Leesonc MS, Stocks NG. Channel selection and classification of electroencephalogram signals: an artificial neural network and genetic algorithm-based approach. Artif Intell Med. 2012; 55 (2):117–26.
- Feldman Y, Ishai PB, Raicu V. Electrode polarization. Dielectric relaxation in biological systems: physical principles, methods, and applications. 2015 Jul 23: 140.
- Allison BZ, Leeb R, Brunner C, Müller-Putz GR, Bauernfeind G, Kelly JW, Neuper C. Toward smarter BCIs: extending BCIs through hybridization and intelligent control. J Neural Eng. 2012 Feb; 9 (1): 013001.
- Pfurtscheller G, Allison BZ, Brunner С, Bauernfeind G, Solis-Escalante T, Scherer R, et al. The hybrid BCI. Front Neurosci. 2010 Apr 21; 4: 30.
- Leeb R, Sagha H, Chavarriaga R, Mill J. Multimodal fusion of muscle and brain signals for a hybrid-BCI. Conf Proc IEEE Eng Med Biol Soc. 2010; 2010: 4343–6.
- Xie P, Chen X, Ma P, Li X, Su P. Identification Method of Human Movement Intention based on the Fusion Feature of EEG and EMG. In: Proceedings of the World Congress on Engineering 2013, vol. 2; 2013 Jul 3–5; London, UK. IAENG; 2013.
- Kiguchi K, Hayashi Y. A study of EMG and EEG during perception-assist with an upper-limb power-assist robot. In: 2012 IEEE International Conference on Robotics and Automation (ICRA); 2012 May 14–18; Saint Paul, MN. IEEE; 2012. p. 2711–6.
- Gopura RARC, Kiguchi K, Li Y. SUEFUL-7: A 7DOF upper-limb exoskeleton robot with muscle-model-oriented EMG-based control. In: 2009 IEEE/RSJ International Conference on Intelligent Robots and Systems; 2009 Oct 11–19; St. Louis, USA. IEEE; 2009. p. 1126–31.
- Witkowski M, Cortese M, Cempini M, Mellinger J, Vitiello N, Soekadar SR. Enhancing brain-machine interface (BMI) control of a hand exoskeleton using electrooculography (EOG). J Neuroeng Rehabil. 2014 Dec 16; 11: 165.
- Rumyantseva SA, Stupin VA, Oganov RG, Silina EV, Petrov VA, Kasymov VA, et al. The ways of long-term blood pressure control systems development. Cardiovascular Therapy and Prevention. 2015; (14) 1: 78–82.
- Stupin VА, Silina EV, Oganov RG, Bogdanov YA, Shusharina NN. Development of an invasive device for long-term remote monitoring of cardiovascular system parameters, including blood pressure, in patients with comorbid conditions. Biosciences Biotechnology Research Asia, Аug 2015. Vol. 12(2), р. 1255-1263.
- Silina E.V., Stupin V.A., Kolesnikova E.A., Rumyantseva S.A., Oganov R.G. New devices for invasive blood pressure detection and the prototype for a long-term invasive distance control of cardiovascular system. Biology and medicine. 2014; 6 (3): BM-045-14, 5 p.
- Pfurtscheller G, Leeb R, Slater M. Cardiac responses induced during thought-based control of a virtual environment. Int J Psychophysiol. 2006 Oct; 62 (1): 134–40.
- Pfurtscheller G, Ortner R, Bauernfeind G, Linortner P, Neuper C. Does conscious intention to perform a motor act depend on slow cardiovascular rhythms? Neurosci Lett. 2010 Jan 1; 468 (1): 46–50.
- Shahid S, Prasad G, Sinha RK. On fusion of heart and brain signals for hybrid BCI. In 2011 5th International IEEE/EMBS Conference Neural Engineering (NER); 2011 Apr 27 – May 11; Cancun, Mexico. IEEE; 2011. р. 48–52.
- Judy JW. Neural interfaces for upper-limb prosthesis control: opportunities to improve long-term reliability. IEEE Pulse. 2012 Mar; 3 (2): 57–60.
- Yanagisawa T, Hirata M, Saitoh Y, Goto T, Kishima H, Fukuma R, et al. Real-time control of a prosthetic hand using human electrocorticography signals: technical note. J Neurosurg. 2011 Jun; 114 (6): 1715–22.