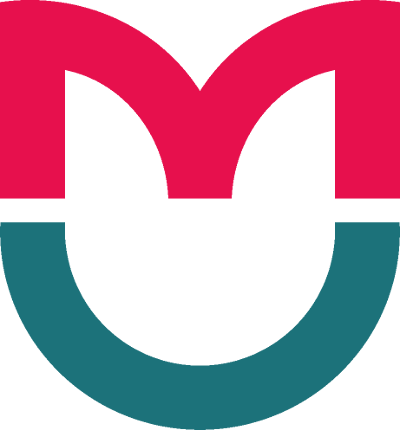
METHOD
Development of medical nomenclature and algorithms for diagnosis and treatment of gout in outpatient settings
Pirogov Russian National Research Medical University, Moscow, Russia
Correspondence should be addressed: Ivan S. Osmolovsky
Ostrovityanova, 1, Moscow, 117997; оur.kb@navi_yksvoloms
Author contribution: Osmolovsky IS built and performed technical verification of the medical nomenclature and algorithms for the diagnosis and treatment of gout, analyzed information provided by the expert panel, wrote the technical section of the paper and prepared figures and tables; Zarubina TV proposed the design of the study, supervised the study, analyzed information provided by the expert panel and performed technical verification of the informational objects; Shostak NA, Kondrashov AA, Klimenko AA collected data for the informational objects, performed clinical verification of the informational objects and wrote the clinical section of this paper.
Compliance with ethical standards: the study was approved by the Ethics Committee of Pirogov Russian National Research Medical University (Protocol № 192 dated January 27, 2020).
Gout is a chronic systemic disease characterized by the deposition of monosodium urate crystals (tophi) in various tissues and the ensuing inflammation in patients with hyperuricemia associated with environmental and/or genetic factors. Gout affects 1–2% of the world’s adult population [1]. According to clinical guidelines, gout can be effectively managed using a treat-to-target approach that consists in achieving and maintaining a target serum urate level. Sustained reduction in serum urate below 360 µmol/L results in the dissolution of monosodium urate crystals and prevents gout flares. However, delays in the initiation of urate-lowering therapy and poor adherence to treatment are common worldwide; therefore, target serum urate levels are rarely achieved, as demonstrated by laboratory tests [1]. Effective strategies are needed to improve the quality of medical care for gout patients.
In Russia, the estimated time from the first gout attack to formal diagnosis is 4–8 years [2–5]; diagnostic delays result in severe complications and comorbidities.
The timely diagnosis of gout is constrained by a number of factors, one of them being the shortage of rheumatologists at primary healthcare and other medical facilities. According to Russian health law [6], there should be at least one rheumatologist per 30,000 population. In practice, there are 0.07 rheumatologists per 10,000 population or 0.21 rheumatologists per 30,000 population, i.e. their number is 5 times lower than recommended [7, 8], hence the delays in delivering adequate medical care to gout patients.
Another problem is the lack of access to polarized light microscopy, the gold standard diagnostic test for gout [1, 9]. It is not a routine method; it cannot be used in every clinical setting and must be performed by specially trained staff [4, 9]. So, practicing physicians have little choice but to exclusively rely on clinical symptoms, which they may misinterpret during the first visit [10].
Modern technology can reduce the rate of medical errors and improve the quality of healthcare delivered to the patient. Among technological successes are diagnostic decision support systems that have been in development since the late 1960s — early 1970s [11–13]. In the past 50 years, a large pool of ready-for-use decisions has been created, many of which are still used in clinical practice. Decision support systems can be categorized in 2 groups [14]:
- systems based on machine learning: genetic algorithms, artificial neural networks, support vectors, etc. [15];
- knowledge-based systems, such as expert systems [13].
Systems that rely on machine learning (medical image processing) exploit massive databases (up to a few thousand cases) and are not required to explain the decision to the clinician have proved to be effective in many medical fields. But in the case of gout, knowledge-based systems that can operate in the absence of sufficient amount of data are more suitable. These systems are based on texts and expert knowledge and can aid the clinician in taking informed decisions [12, 13, 16].
A knowledge base is the product of knowledge engineering that develops methods for knowledge extraction, structuring and formalization. There are a few established models of knowledge representation, including production models, frames, sematic networks, etc. [12, 13, 17]. However, the modularity, ambiguity, incompleteness, and some other characteristics of a given subject area are difficult to represent using existing models [12]. To circumvent these challenges, an ontological approach can be applied; it allows developing a hierarchical nomenclature of strictly defined medical entities [12].
An ontology is a formal specification of a shared conceptual model, i.e. an abstract model of a given subject field that explicitly describes the conceptual framework of this field, is accepted by a given community and has a formal representation [12, 13]. Conventionally, ontologies are represented by semantic networks, i.e. the entirety of linked concepts; however, there are other methods of knowledge representation, including frames and production rules.
Thus, building an ontology is one of the key stages in the development of an expert system that can provide a clinician with comprehensive information about a disease. But building a medical ontology is impossible without a nomenclature of medical entities and diagnostic/treatment algorithms [12, 13]; so far, no such information objects have been constructed.
The aim of this study was to develop formal algorithms for the diagnosis and treatment of gout in an outpatient setting that could be further used to build an expert system.
METHODS
Federal clinical guidelines approved by the Russian Ministry of Health [1] were used as the main source of data for creating a medical nomenclature and diagnostic/treatment algorithms for gout. The State Registry of Medicinal Products [18] and academic publications were used as additional sources of information.
Eligibility criteria for experts participating in the development of an expert system or a similar product are not explicitly specified in Russian normative documents, so the selection was based on the formal indicators of expertise, including academic credentials, position held, and over 8 years of experience in rheumatology. The panel of experts consisted of 3 highly skilled rheumatologists from Nesterov Department of Faculty Therapy at Pirogov Russian National Research Medical University.
Diagnostic and treatment algorithms for gout were developed using MS Excel (Microsoft; USA), MS Excel Online (Microsoft; USA) and Visio (Microsoft; USA) software.
The process of developing the nomenclature of medical entities pertaining to the diagnosis and treatment of gout included the following steps:
- entities were identified using text-based methods of knowledge extraction;
- working independently — each member of the panel made corrections to the nomenclature by modifying, adding or removing the identified entities;
- the obtained results were validated by comparison and voting.
For the comparison procedure, each expert was given a nomenclature version drawn from textual sources. The members of the expert panel revised and modified the received nomenclature independent of each other. The resultant versions were compared, and the variations were brought forward for voting so as to enrich the final version of the nomenclature with new entities.
Diagnostic algorithms were developed using 2015 ACR/ EULAR gout classification criteria. Treatment algorithms were based on the clinical guidelines proposed by the Russian Association of Rheumatologists [1, 19]. Diagnostic and treatment algorithms for gout were developed following the same steps as in the development of the medical nomenclature.
RESULTS
Medical nomenclature
Creating a nomenclature of medical entities pertaining to the diagnosis and treatment of gout was a multistep process that allowed us to identify 1,174 entities. As part of this process, we were faced with the need to formulate a number of requirements in order to keep the nomenclature from overgrowing and to get rid of some nomenclature items that were not used in decision making. Briefly, we had to
- Identify entities related to the diagnosis and treatment of gout only. Entities not related to gout were not included in the nomenclature. For example, the entities “heartburn” or “gastric ulcer” are not used to diagnose or treat gout, so they were not included in the nomenclature.
- Extract revised terminology. For example, the term “urate”/“uric acid” was not included in the nomenclature because it lacks accuracy: uric acid can be measured in various bodily fluids like urine, synovial fluid etc., and its reference levels used for diagnostic or monitoring purposes are different. Therefore, a more precise term should be used, i.e. “serum urate”. By mapping the studied terms to the SNOMED databased, we were able to refine a number of terms.
- Arrange synonyms into groups. The terminology that describes a specific phenomenon may differ across schools of medical thought. Varying terminology should be arranged in groups of synonyms. For example, “intermittent gout, intercritical period” can be clustered with “intercritical gout”. It is important to select an entry that will be used by the expert system as the main term and to identify its synonyms that will redirect the system to the main term. Usually, the most abbreviations of the term are regarded as its synonyms.
- Extract umbrella terms to arrange revised terminology into groups. For example, “tophus” is an umbrella term for “subcutaneous tophi”, “intraosseous tophi”, and some other entities.
- Medicinal drugs should be represented by their active pharmaceutical substances only; it is important to exclude trade names from the nomenclature.
- Extract quantitative terms. For example, the entry “serum urate” should be annotated with its reference intervals.
Prior to working on the nomenclature, the expert panel enunciated a list of final diagnoses based on their clinical experience and verified literature sources [1, 19]. There were two components to the formulated diagnoses: the clinical stage of gout and the functional class of the affected joint (tab. 1).
The analysis of textual sources yielded a nomenclature of 132 main terms and 77 synonyms pertaining to gout diagnosis. This list was revised by the expert panel; the final version of the “diagnostic” nomenclature encompassed 170 main terms and 470 synonyms. For gout therapy, the initial nomenclature compiled from literature sources included 324 main terms and 213 synonyms; its revised version comprised 387 main terms and 515 synonyms.
Some of the terms were present in both nomenclatures; after the nomenclatures were merged, the total number of main terms and their synonyms reached 495 and 679, respectively.
The main terms were broken down in 8 types (tab. 2). In the future, this terminology will be used for building an ontology to aid the diagnosis and treatment of gout.
Diagnostic algorithms
Based on Federal Clinical Guidelines [1, 19], diagnostic algorithms for gout were elaborated in a series of steps and revised. Diagnostic algorithms were developed for each of 20 definitions of clinical forms of gout formulated by the expert panel. A total of 40 scenarios were proposed (tab. 3) and 52 synonyms were used.
The refined definitions of clinical forms of gout are variants of the final diagnosis that are based on various combinations of the clinical stage of gout and the functional classification of the affected joint [19, 20] (see tab. 1). From the diagnostic perspective, both components can be regarded as independent entities. The algorithm for classifying the functional state of the affected joint is fully consistent with the Federal Clinical Guidelines [19].
The algorithm for identifying the clinical stage of gout was based on the 2015 ACR/EULAR international classification criteria [1]. The algorithm consists of 3 steps. The first step is essentially the recognition of acute gout, which involves the analysis of symptoms indicative of inflammation in the ankle, first metatarsophalangeal and mid-foot joints. While refining and revising the nomenclature, the panel expanded the list of symptoms to include knee joint inflammation and peripheral joint inflammation, which refers to the inflammation of 59-61 joints that are at lower risk for being affected by gout. Each feature (symptom) contributes to the final diagnosis and is assigned points. The threshold score for diagnosing gout is ≥ 8 points on the ACR/EULAR scale. However, the following criteria must be taken in account when using the ACR/EULAR classification:
Time-course of the disease
Some features contribute to the diagnosis when used in combination with other features. For example, “time to maximal pain during the attack” < 24 h and “duration of gouty arthritis attack” of 10–14 days jointly score 1 point. The co-occurrence of these symptoms with the feature called “complete resolution of gouty arthritis symptoms” (in 10–14 days) does not affect the total score. Here, an individual feature is not assigned any points. No less significant is the combination of temporal characteristics of the disease with recurrent typical episodes: it increases the total score by 2 points.
Serum urate levels
“Serum urate” can score from –2 to 4 points, depending on its actual concentration in the blood serum. Negative values should also be accounted for when developing rules for a clinical decision support system.
Polarized light microscopy of synovial fluid
According to clinical guidelines, the findings of polarized light microscopy of synovial fluid should be described in terms of 1) presence of monosodium urate crystals in synovial fluid (8 points), 2) absence of monosodium urate crystals in synovial fluid (–2 points) and 3) no test results available (0 points).
Importantly, it is not the binary choice (the presence or absence of a symptom) that should be implemented in a clinical decision support system, but the choice between 3 states: the presence of a symptom, its absence or no data available.
The algorithms, their scores and the logic for score calculation needed for further ontology building were described in MS Excel. Symptoms that could be expressed numerically were represented as a range of possible values and units of measurement consistent with the information in the Units of Measurements reference book [21].
The designed diagnostic algorithms were approved by the expert panel using the comparison method. The experts confirmed that the algorithms were verified and could be used for ontology building.
Algorithms for gout treatment
The process of developing algorithms for gout treatment differed from the process of developing diagnostic algorithms. Treatment algorithms were broken down into the following steps: 1) selecting a general therapeutic strategy; 2) selecting an optimal pharmaceutical substance(s); 3) determining hospitalization criteria.
To provide a clear description of the therapeutic strategy, the panel of experts working in collaboration with the authors of this paper proposed a logical model that comprised 50 different scenarios of treating a gout attack. The algorithms were visualized in MS Visio (Fig. 1), which enabled us to discuss every scenario without going into technicalities. The following steps are included in each scenario:
- a drug category is chosen (a specific drug will be proposed in the next step); the choice is made between colchicine, NSAIDs, proton pump inhibitors, glucocorticoids, canakinumab, and the combination of these drugs. Due to the specifics of glucocorticoid therapy, glucocorticoid entries are annotated with information about the route of administration (intra-articular, intramuscular, oral);
- the timeline for medical consultations is specified; consultations are seen as transition points between different treatment scenarios and hospitalization;
- criteria are set for scenario selection and switching between different scenarios.
Scenarios for gout attack prevention (small doses of colchicine, NSAIDs, glucocorticoids) and serum urate lowering (allopurinol, febuxostat) should be analyzed separately.
In the second step, one or several active pharmaceutical substances are selected as a possible treatment option. These substances will be recommended later, in the form of a regimen that accounts for the drug dose, dosing frequency, route of administration, etc. Each active substance is annotated with a list of contraindications so that dangerous prescriptions could be avoided.
Treatment algorithms were described in MS Excel (Fig. 2). The resultant table contains information returned to the user by the system if the specified criteria are met.
The following rules and limitations were introduced during the development of treatment algorithms for gout patients:
- Priority should be given to a higher-ranked drug, i.e. the system will recommend the drug if prescription criteria are met and no contraindications are detected.
- If none of the drugs (active substances) can be prioritized or prescription criteria for these drugs are different, the drugs should be grouped together (see the Group number box in Fig. 2).
- If several active substances are prescribed, this information will be visually presented to the user as shown in Fig. 3. Example: a combination therapy of one NSAID and one PPI is recommended. Priority is set according to the specified criteria but information is presented to the user in separate blocks.
Thus, the entire process of treatment can have a structured formal representation; at the same time, the rules underlying decision making are available to the user and can be analyzed.
The proposed treatment algorithms were compared, verified and recommended for further ontology building by the panel of experts.
DISCUSSION
The proposed verified nomenclature of medical entities pertaining to the diagnosis and treatment of gout has laid the foundation for the future ontology. The rigorous classification scheme and the generalization of entities suggest that the conceptual model, which is currently in development, has a specification: each entity included in the model has an explicitly described role. Categorizing the entities into the groups of main terms and their synonyms is important for ontology building because it allows using terminologies from different schools of medical thought and expanding the existing list of terms. Thus, the principle of a shared conceptual model is implemented. Because clinical diagnoses had unambiguous definitions, we were able to proceed to the development of treatment algorithms for gout patients.
The proposed verified diagnostic algorithms will be later used to build the diagnostic domain of the future ontology. The identified patterns (diagnostic stages, specific work of logic with individual entities (or their groups) that contribute to the diagnosis of gout, the need to use 3 states for some entities that explicitly affect the diagnosis) will underpin the structure of the future ontology, help to fill it with data and be used to elaborate algorithms for the expert system. Unambiguous definition of units of measurement for all quantitative symptoms also suggest that our conceptual model has a specification.
The proposed verified treatment algorithms will be used to build the treatment domain of the future ontology, fill it with data and develop an expert system for the optimization of gout treatment regimens and patient monitoring.
The algorithms proposed in this paper are described in MS Excel. This means that the accumulated knowledge now has a formal representation and can be transferred to an ontology by means of special software tools. On the other hand, MS Excel tools can be used by experts to further expand the ontology.
We were unable to compare our medical nomenclature of gout-related terms with similar informational objects because currently existing expert systems either are based on a different approach [22] or do not provide data for comparison [23]. On the other hand, there is a wealth of studies that utilize similar methods of knowledge extraction, structuring and formalization but pertain to other medical fields, like angina pectoris [24], intestinal bleeding [25], etc. However, it is impossible to objectively compare the results of those studies with the results of our work.
CONCLUSION
We have developed a medical nomenclature of terms pertaining to the diagnosis and treatment of gout using literature sources and expert opinions. The diagnostic domain includes 179 main terms and 470 synonyms, whereas the treatment domain comprises 387 main terms and 515 synonyms. We have also designed formal diagnostic and treatment algorithms for gout patients (40 and 50 scenarios, respectively) that will be instrumental in building a clinical decision support system suitable for use in an outpatient setting.