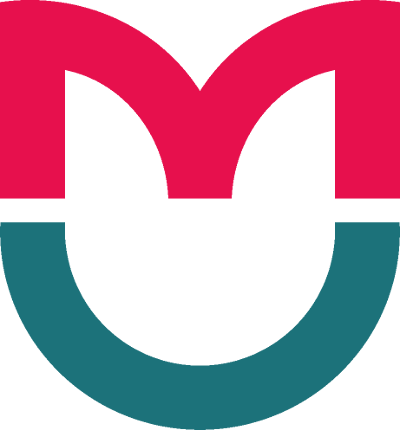
ORIGINAL RESEARCH
Activation of sensorimotor integration processes with a brain-computer interface
Federal Center for Brain Research and Neurotechnologies of FMBA, Moscow, Russia
Correspondence should be addressed: Alexandra Rubakova
Ostrovityanova, 1, str. 10, Moscow, 117997; ur.xobni@goloibardnas
Author contribution: Rubakova AA — data acquisition, analysis, interpretation; literature analysis; Ivanova GE — study design; manuscript editing; Bulatova MA — data acquisition and analysis.
Compliance with ethical standards: the study was approved by the Ethics Committee of the Federal Center for Brain Research and Neurotechnologies (Protocol № 33 dated June 21, 2021.). Informed consent was obtained from all study participants.
Integration of sensory and motor information is central to successful goal-directed behavior and movements necessary to interact with the environment. Compromised sensorimotor integration is common to many neurological conditions, including stroke, which may be caused, among other things, by traumatic brain injury (TBI) [1]. In motor control, sensory feedback during movement is predicted from an internal copy of the motor command. If a prediction generated by the motor cortex matches the actual sensory feedback, a stable motor pattern is formed and the entire sensory experience is retained [2]. The human ability to link a performed action to its immediate consequences presented in the form of perceptual information is called sensorimotor integration.
Stroke is a common disorder that causes disruption of sensorimotor integration through sensory impairments, hemiparesis, limb spasticity, hemianopsia, ataxia, and apraxia [2]. Most stroke patients develop persistent hand paresis, which reduces the functional range of hand motion and adversely affects the patients’ quality of life. Modern post-stroke rehabilitation has harnessed the principles of neuroplasticity to promote motor learning and regain the lost motor function. However, the contribution of perception to motor control and learning is often overlooked and, to this day, remains understudied [1]. In developmental psychology, sensorimotor integration in the cortex is considered an important factor promoting learning [3]. It is thought that learning movement patterns is essential for post-stroke recovery [4].
It is hypothesized that neuroplasticity mechanisms are underpinned by long-term potentiation and long-term depression of brain neurons [5]. In motor learning, the key role is attributed to the primary motor cortex; during active motor learning and at rest, IEGs expression is stimulated in the neurons of the primary motor cortex and neurotrophic factors are synthesized, modulating neural networks and promoting consolidation of the acquired information [5].
Generation of the sensorimotor μ-rhythm registered during motor imagery and motor execution is linked to efferent processes and motor performance but can also take place during afferent nerve activity, such as passive extension of the affected limb with a robotic orthosis. Some studies argue the effectiveness of post-stroke rehabilitation involving premovement sensorimotor rhythm training. It is reported that motor function of the affected limb can be successfully recovered by means of sensorimotor rhythm training in which the amplitude of the rhythm is measured to ensure effective control of a robotic orthosis relying on the principles of biofeedback (based on the actual movement or its mental rehearsal). There is evidence that combined activity of the sensory, motor and temporal cortices is associated with proprioceptive and tactile afferent signals from the moving limb, which allows the effects of motor function training to be measured [6].
Neuroplasticity-based motor learning occurs due to physiological stimulation of peripheral proprioceptors during physical exercise and sensorimotor integration of afferent and efferent signals in the cerebral cortex. Over the past decade, there has been an extensive development of robotic hands and rehabilitation devices for patients with sensorimotor deficits after CNS injury [7]. Innovative robotic rehabilitation devices rely on the principle of biofeedback, which improves the effectiveness of motor learning; however, little attention has been paid to motor learning capacity of stroke patients and factors that may affect it [4].
Robot-assisted motor rehabilitation of upper limb function is being increasingly introduced into clinical practice. One example is robotic orthoses for active and passive hand muscle training after an acute cerebrovascular accident (CVA) in the sensorimotor cortex and/or pyramidal tract injury [8]. Today, robot-assisted rehabilitation is the only method that can activate neuroplastic mechanisms: motor function recovery, which is the ultimate goal of neurorehabilitation in such patients, requires at least 400 repetitions for a movement pattern to be ingrained in the patient’s memory. Long-term motor adaptation with biofeedback for simultaneous error correction is possible only with a brain-computer interface (BCI) [9–12].
However, in order to achieve a clinically noticeable improvement, movement repetition is not enough. For a positive outcome, multisensory stimulation, e.g. simultaneous stimulation of visual, vestibular and proprioceptive analyzers, and cognitive function training are needed [13]. It is known that apart from motor function, BCI-controlled feedback training stimulates intrapsychic activity [14]. So, it can be hypothesized that robot-assisted neurorehabilitation of patients with hemiparesis after CVA effectively promotes sensorimotor integration, which plays the leading role in the formation of movement forming.
Our goal was to examine a hypothesis proposed by Bertani et al., which suggests that although post-stroke neurorehabilitation with a robotic hand potentiates activation of neuroplasticity mechanisms in the affected hemisphere, it does not exert any significant effect on muscular tone reduction in the paretic limb [13]. We decided to test this hypothesis because clinicians often prescribe biofeedback-based ideomotor training to stroke patients to reduce spasticity. So, there is a need to check whether such interventions are reasonable and can improve the outcome of neurorehabilitation.
The aim of this study was to assess the impact of sensorimotor integration on the effectiveness of neurorehabilitation based on the learning of a hand opening movement in stroke patients using BCI and to study the effect of ideomotor training on spasticity in the paretic hand.
METHODS
The study was conducted at the Federal Center for Brain Research and Neurotechnologies; it began on December 19, 2019 and ended on October 8, 2020. Fifty-eight patients were included in the study. The sample was dominated by male patients (42 men vs. 16 women). The mean age of the participants was 62.5 ± 5 years. The youngest participant was 22 years old, the oldest one was 83 years old. The mean time elapsed after stroke was 2 months. Thus, 80% of our patients were in the early rehabilitation period (1 to 6 months after CVA), and 20% were in the late rehabilitation period. All study participants were right-handed, according to the Edinburgh Handedness Inventory.
Of all the patients included in the study, 76% (44 persons) were undergoing rehabilitation after ischemic stroke, 15.5% (9 persons) after hemorrhagic stroke, 5% (3 persons) after ischemic stroke with hemorrhagic transformation, and 3.5% (2 persons) after TBI.
The sample was dominated by patients with subcortical lesions. The right middle cerebral artery was involved in most patients (20 persons; 35.7%); the left middle cerebral artery was involved in 17 (30.3%) patients.
On neurological examination, deep sensation was preserved in 93% of the patients, whereas superficial sensation, in 48% of the patients; 52% of the patients had superficial hemihypesthesia contralateral to the affected hemisphere; 98% of the patients had hemihipestesia to pain, temperature and touch, i.e. the total loss of sensation. Deep sensation impairments were detected in 11 (19%) patients.
The following inclusion criteria were applied: retained cognitive function (at least 12 points on the Montreal Cognitive Assessment scale); the absence of severe aphasia which could have prevented the patient from understanding instructions for ideomotor training; the absence of severe visual impairment; spasticity in the paretic hand ≤ 4 points on the modified Ashworth scale; mild to moderate disability on the Rankin scale (≤ 3 points); the absence of muscle contractures in the affected limb; the absence of pronounced pain that might have interfered with ideomotor training; informed consent given by the patient or their legal representative.
Exclusion criteria: inability to perform training tasks; refusal to participate in the study; severe aphasia; severe visual impairment preventing the patient from following instructions on the computer screen; spasticity of 5 points on the modified Ashworth scale; severe disability (>3 points on the Rankin scale); muscle contractures in the affected limb; pronounced pain.
On average, the patients scored 25.4 points on the Montreal Cognitive Assessment scale (MoCA) during the initial neurological assessment, with 75% of patients scoring in the range between 23 and 26 points.
The training sessions were carried out in accordance with a standard (2020) protocol using BMI-controlled Exokist-2 orthoses for both hands (Android Technics; Russa). The interactions between the patient and the Exokist-2 rehabilitation complex were guided by the principles of biofeedback.
Rehabilitation was aided by a biofeedback-based Exokist-2 robotic device. The method relies on 2 approaches to robot-assisted post-stroke rehabilitation of upper limbs: 1) a neurophysiological approach based on the ideas of neuroplasticity and compensatory potential of the brain; 2) motor learning through performing goal-directed tasks by the paretic hand and motor imagery with multisensory feedback. Exokist-2 utilizes multimodal (primarily visual and proprioceptive) biofeedback to a registered intention to execute a movement.
During the session, the patient was sitting in a medical chair, with both hands inside the robotic gloves secured to the armrests. The screen was positioned 1 m in front of the patient. A pictorial instruction for gaze fixation appeared on the screen and was then followed by oral instructions: to perform kinesthetic imagery of left/right hand opening (depending on the direction of the arrow on the screen) or to rest [13].
EEG data were recorded during the session using an NVX52 amplifier (Medical computer systems; Russia), which is a component of the Exohand-2 system. It helps to identify the task a patient performs at a given moment in time. EEG data were collected from 32 electrodes positioned at F3, Fz, F4, Fc5, Fc3, Fc1, Fcz, Fc2, Fc4, Fc6, C5, C3, C1, Cz, C2, C4, C6, Cp5, Cp3, Cp1, Cpz, Cp2, Cp4, Cp6, P3, Pz, P4, Po3, Poz, Po4, O1, and O2. Task recognition was carried out using a Bayesian classifier for the analysis of EEG covariance matrices. The result was presented to the patient as visual and proprioceptive feedback: if the classifier recognized the task given to the patient, the color of the cursor on the screen changed to green and the Exohand opened [15].
The motor imagery training course lasted for 7–9 days, with 2-3 sessions a day. Each session was 9 min long, as recommended in the protocol for adult patients [16]. On average, the patient received 15 (12; 21) sessions with Exokist-2 exercises.
The effectiveness of ideomotor training with Exokist-2 was estimated using scales for the assessment of functional movements necessary for everyday activities. We also compared the results of neurological examinations conducted before and after the rehabilitation course. The following scales were used: the modified Ashworth scale, the Fugl-Meyer Assessment scale, the ARAT scale, the Frenchay Activities Index, the Rankin scale, the Rivermead Mobility Index, and FIM.
RESULTS
The analysis of clinical data revealed a direct correlation between age and proprioception impairment, previously demonstrated in [17]. The factor analysis exposed a statistically significant moderate correlation (the Chaddock scale) between deep sensory impairments and the improvement of hand function on the Fugl-Meyer Assessment (ρ = 0.32; p < 0.02) and ARAT (ρ = 0.454; p = 0.0006) scales.
We found that ideomotor training enhances hand muscle strength during movement in the radiocarpal and metacarpophalangeal joints. Muscle strength was assessed on a 5-point scale before and after 15 neurorehabilitation sessions with Exokist-2. The results were compared, to reveal an improvement in the flexion and extension of the radiocarpal joint in 23 (40%) patients and in the adduction and abduction of this joint in 17 (29%) patients. Besides, we found that muscle strength equally increased with the abduction and adduction of the radiocarpal joint, which may be explained by sensorimotor integration recovery due to neuroplasticity and activation of receptors in wrist muscles. Muscle strength improvements are analyzed in tab. 1.
Hand function improvement on the Fugl-Meyer scale was observed in 63% of the patients after the neurorehabilitation course with Exokist-2; however, it was clinically significant (by 5 points or more) in only 26% of the patients.
Improvements in the Frenchay Activities Index were observed in 26% of the patients (15 persons).
Statistically significant improvements on the ARAT scale were detected in 38% of the patients. However, their distribution was non-uniform: 13 patients increased their scores by 2-7 points, and 9 patients – by 17-55 points. It should be noted that improvement was demonstrated primarily by those patients who had scored 0 on the ARAT scale on the initial neurological examination.
Improved hand function performance was also reflected in higher FIM and Rivermead Mobility Index. Perhaps, it may be explained by the fact that robot-assisted motor function training for the paretic limb activates kinesthetic receptors, improving fine motor skills and restoring sensation in the upper limb through better sensorimotor integration. Improvements in sensorimotor hand activity and functional independence are analyzed in tab. 2.
Our test of Bertani’s hypothesis [13] showed that the degree of spasticity reduction in the paretic hand assessed on the modified Ashworth scale was statistically insignificant (p = 0.001); muscle tone reduction was observed in only 8 (14%) patients. Thus, neuroplasticity mechanisms do not exert a significant effect on spasticity in the paretic hand.
DISCUSSION
Patients with hemiparesis and neurological deficits after CVA demonstrated a statistically significant improvement in the motor activity and sensibility of the hand, as well as in their functional everyday activities, after robot-assisted neurorehabilitation with Exohand-2. After 15 sessions, improved upper limb function was reported by most patients.
Perhaps, integration between the primary cortex and Brodmann area 6 of the frontal cortex are disrupted in advancedage stroke patients not only because of stroke itself, but also due to age-related involution of the grey matter. This explains why neurorehabilitation with Exokist-2 was lowly effective in patients with premotor apraxia, as demonstrated by all applied scales, because the primary task of ideomotor training is motor imagery, which occurs in Brodmann cytoarchitectonic area 6 [17, 18].
The literature data suggests that patients who benefit from ideomotor training recover impaired integration between the posterior parietal cortex, which is responsible for producing a motor intention, the supplementary motor cortex and the primary motor cortex responsible for movement execution recovers through the activation of neuroplasticity mechanisms [19]. As motor function is being regained, sensorimotor integration is improving between the visual cortex, which perceives visual stimuli containing instructions for the patient, the frontal cortex, which plays the key role in the perception of body scheme and kinesthetic inputs, and the parietal, dorsal premotor and motor cortices. Therefore, the term “sensorimotor cortex” can be applied not only to the precentral and postcentral gyri, but to all brain areas mentioned above, although they are spatially isolated. It is the sensorimotor cortex that generates the 8-12 Hz μ-rhythm registered by EEG during a trainings session with Exokist-2 and further analyzed to classify the result of the performed task. Spontaneous μ-rhythm desynchronization (suppression) occurs before and during the actual movement, whereas spontaneous desynchronization (enhancement) occurs after the movement has been executed. The mechanism underlying μ-rhythm generation is understudied, but there is evidence that it occurs as a result of the coordinated activity of the premotor and motor cortices and subcortical and spinal centers [7, 20].
Our findings suggest that BCI-based neurorehabilitation reduces the degree of disability on the Rankin scale.
Sensorimotor integration, which was expected to be activated by ideomotor training, improved significantly after the rehabilitation course. This was reflected in the clinical data: the functional independence and daily activities of our patients directly depended on their motor activity, accuracy and speed of afferent signal transmission from peripheral receptors. Training with Exokist-2 improves hand motor skills and activates visual, kinesthetic and auditory perception. Regular multimodal biofeedback-based training activates neuroplasticity mechanisms and results in the consolidation of sensory information acquired during task performance. Auditory, visual and kinesthetic analyzers, systems involved in body schema perception, motor functions and intrapsychic processes, such as motivation and memory, interact with each other, which directly indicates stimulation of sensorimotor integration processes.
CONCLUSIONS
Reduction of neurological deficit revealed by the analysis of clinical data and observations indicating activation of sensorimotor integration processes after a course of ideomotor training sessions suggests the effectiveness of post-stroke rehabilitation with Exokist-2. Therefore, a robotic orthosis controlled by non-invasive BCI can be recommended for clinical use as part of complex neurorehabilitation after CVA.