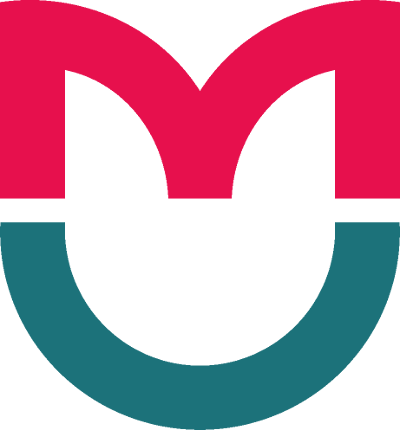
ОРИГИНАЛЬНОЕ ИССЛЕДОВАНИЕ
Алгоритм сегментации ОКТ изображений макулы для анализа пациентов с возрастной макулярной дегенерацией
1 Башкирский государственный медицинский университет, Уфа, Россия
2 Уфимский государственный авиационный технический университет, Уфа, Россия
3 Закрытое акционерное общество «Оптимедсервис», Уфа, Россия
Для корреспонденции: Рада Радиковна Ибрагимова
ул. Ленина, д. 3, г. Уфа, 450008, Россия; ur.xednay@6102adar.avomigarbi
Финансирование: исследование частично выполнено в рамках работ по государственному заданию Минобрнауки России для ФГБОУ ВО «УГАТУ» (код научной темы #FEUE-2021-0013, соглашение № 075-03-2021-014) в молодежной научно-исследовательской лаборатории НОЦ «Сенсорные системы на основе устройств интегральной фотоники» (разделы «Материалы и методы», «Результаты исследования», «Обсуждение результатов»), а также в рамках проекта, поддержанного субсидией в области науки из бюджета Республики Башкортостан для государственной поддержки научных исследований, проводимых под руководством ведущих ученых (НОЦ-РМГ-2021, соглашение с ФГБОУ ВО «УГАТУ») (раздел «Введение»).
Вклад авторов: Р. Р. Ибрагимова — обзор литературы, получение и анализ данных, написание статьи; И. И. Гильманов — разработка программного обеспечения, поиск базы данных, тестирование существующих компонентов кода; Е. А. Лопухова — разработка программного обеспечения, написание статьи, получение и анализ данных; И. А. Лакман, Т. Р. Мухамадеев, Р. В. Кутлуяров — концепция и дизайн исследования, научное редактирование; А. Р. Билялов — научное редактирование; Г. М. Идрисова — анализ данных, научное редактирование.
Соблюдение этических стандартов: исследование проведено в соответствии с принципами Хельсинкской декларации; все пациенты подписали добровольное информированное согласие на проведение ОКТ.
- Zapata MA, Royo-Fibla D, Font O, Vela JI, Marcantonio I, Moya-Sánchez EU, et al. Artificial intelligence to identify retinal fundus images, quality validation, laterality evaluation, macular degeneration, and suspected glaucoma. Clinical Ophthalmology (Auckland, NZ). 2020; 14: 419. Available from: https://www.ncbi.nlm.nih.gov/pmc/articles/PMC7025650/.
- Stark K, Olden M, Brandl C, Dietl A, Zimmermann M E, Schelter SC, et al. The German AugUR study: study protocol of a prospective study to investigate chronic diseases in the elderly. BMC geriatrics. 2015; 15 (1): 1–8. Available from: https://link.springer. com/article/10.1186/s12877-015-0122-0.
- Mehta S. Age-related macular degeneration. Primary Care: Clinics in Office Practice. 2015; 42 (3): 377–91. Available from: https:// www.primarycare.theclinics.com/article/S0095-4543(15)00042-1/fulltext.
- Lawrenson JG, Evans JR, Downie LE. A critical appraisal of national and international clinical practice guidelines reporting nutritional recommendations for age-related macular degeneration: are recommendations evidence-based? Nutrients. 2019; 11 (4): 823. Available from: https://www.mdpi.com/20726643/11/4/823.
- Li JQ, Welchowski T, Schmid M, Mauschitz M M, Holz FG, Finger RP. Prevalence and incidence of age-related macular degeneration in Europe: a systematic review and meta-analysis. British Journal of Ophthalmology. 2020; 104 (8): 1077–84. Available from: https:// bjo.bmj.com/content/104/8/1077.abstract.
- Всемирный доклад о проблемах зрения [World report on vision]. Женева: Всемирная организация здравоохранения, 2020. Лицензия: CC BY-NC-SA 3.0 IGO.
- Балашевич Л. И., Измайлов А. С., Улитина А. Ю. Модифицированная клиническая классификация возрастной макулярной дегенерации. Офтальмологические ведомости. 2011; 4 (4): 41–47. Available from: https://cyberleninka.ru/article/n/modifitsirovannaya-klinicheskaya-klassifikatsiya-vozrastnoymakulyarnoydegeneratsii.
- Авдеева О. Н., Аветисов С. Э., Аклаева Н. А., Акопов Е. Л., Алексеев В. Н., Астахов С. Ю., и др. редакторы. Офтальмология: национальное руководство. М.: ГЭОТАР-Медиа, 2018; 625 с.
- Ferris III FL, Wilkinson CP, Bird A, Chakravarthy U, Chew E, Csaky K, et al. Beckman Initiative for Macular Research Classification Committee. Clinical classification of age-related macular degeneration. Ophthalmology. 2013; 120 (4): 844–51. Available from: https://www.sciencedirect.com/science/article/ abs/pii/S016164201201055X.
- Hyttinen JM, Kannan R, Felszeghy S, Niittykoski M, Salminen A, Kaarniranta K. The regulation of NFE2L2 (NRF2) signalling and epithelial-to-mesenchymal transition in age-related macular degeneration pathology. International journal of molecular sciences. 2019; 20 (22): 5800. Available from: https://www.mdpi. com/1422-0067/20/22/5800.
- Friedman DS, O’Colmain BJ, Munoz B, Tomany SC, McCarty C, De Jong Pt, et al. Prevalence of age-related macular degeneration in the United States. Arch ophthalmol. 2004; 122 (4): 564– 72. Available from: https://jamanetwork.com/journals/ jamaophthalmology/article-abstract/416232.
- Schultz NM, Bhardwaj S, Barclay C, Gaspar L, Schwartz J. Global Burden of Dry Age-Related Macular Degeneration: A Targeted Literature Review. Clin Ther. 2021; 43 (10): 1792–818. DOI: 10.1016/J.CLINTHERA.2021.08.011.
- The Age-Related Eye Disease Study Research Group. A randomized, placebocontrolled, clinical trial of supplementation with vitamins C and E and beta-carotene for age related cataract and vision loss: AREDS report number 9. Arch. Ophthalmol. 2001; 119: 1439–52.
- Varma R, Bressler NM, Doan QV, Danese M, Dolan CM, Lee A, et al. Visual impairment and blindness avoided with ranibizumab in Hispanic and non-Hispanic whites with diabetic macular edema in the United States. Ophthalmology. 2015; 122 (5): 982– 89. Available from: https://www.sciencedirect.com/science/ article/pii/S0161642014011476.
- Азнабаев Б. М., Мухамадеев Т. Р., Дибаев Т. И. Оптическая когерентная томография + ангиография глаза в диагностике, терапии и хирургии глазных болезней. М.: Август Борг, 2019; 57 с.
- Drexler W, Fujimoto JG, editors. Optical coherence tomography: technology and applications. Berlin: Springer, 2015; 2.
- Victor AA. The Role of Imaging in Age-Related Macular Degeneration. In Visual Impairment and Blindness-What We Know and What We Have to Know. London, UK: IntechOpen, 2019.
- Schmidt-Erfurth U, Sadeghipour A, Gerendas BS, Waldstein SM, Bogunović H. Artificial intelligence in retina. Progress in retinal and eye research. 2018; 67: 1–29. Available from: https://www. sciencedirect.com/science/article/pii/S1350946218300119.
- Venhuizen FG, van Ginneken B, van Asten F, van Grinsven MJ, Fauser S, Hoyng CB, et al. Automated staging of age-related macular degeneration using optical coherence tomography. Investigative ophthalmology & visual science. 2017; 58 (4): 2318–28. Available from: https://iovs.arvojournals.org/article. aspx?articleid=2623584.
- Шляпникова О. А., Каменских Т. Г., Рощепкин В. В., Решникова Л. Б. Перспективные направления развития офтальмологии (обзор). Саратовский научно-медицинский журнал. 2021; 17 (3): 675–8. Available from: https://cyberleninka.ru/article/n/perspektivnye-napravleniya-razvitiya-oftalmologii-obzor.
- Quellec G, Lee K, Dolejsi M, Garvin MK, Abramoff MD, Sonka M. Three-dimensional analysis of retinal layer texture: identification of fluid-filled regions in SD-OCT of the macula. IEEE transactions on medical imaging. 2010; 29 (6): 1321–30. Available from: https://ieeexplore.ieee.org/abstract/document/5440910.
- Hu Z, Niemeijer M, Abramoff MD, Garvin MK. Multimodal retinal vessel segmentation from spectral-domain optical coherence tomography and fundus photography. IEEE transactions on medical imaging. 2012; 31 (10): 1900–11. Available from: https:// ieeexplore.ieee.org/abstract/document/6228540.
- Esmaeili M, Dehnavi AM, Rabbani H, Hajizadeh F. Threedimensional segmentation of retinal cysts from spectral-domain optical coherence tomography images by the use of threedimensional curvelet based K-SVD. Journal of medical signals and sensors. 2016; 6 (3): 166. Available from: https://www.ncbi.nlm.nih.gov/pmc/articles/PMC4973460/.
- Chakravarty A, Sivaswamy J. A supervised joint multi-layer segmentation framework for retinal optical coherence tomography images using conditional random field. Comput. Methods Programs Biomed. 2018; 165: 235–50. DOI: 10.1016/J. CMPB.2018.09.004.
- Bogunović H, et al. Machine Learning of the Progression of Intermediate Age-Related Macular Degeneration Based on OCT Imaging. Investigative ophthalmology & visual science. 2017; 58 (6): BIO141–BIO150.
- Tvenning AO, Hanssen SR, Austeng D, Morken TS. Deep learning identify retinal nerve fibre and choroid layers as markers of agerelated macular degeneration in the classification of macular spectral‐domain optical coherence tomography volumes. Acta Ophthalmologica. 2022. DOI: 10.1111/AOS.15126.
- Rim TH, et al. Detection of features associated with neovascular age-related macular degeneration in ethnically distinct data sets by an optical coherence tomography: trained deep learning algorithm. British Journal of Ophthalmology. 2020; 105 (8): 1133–9. DOI: 10.1136/BJOPHTHALMOL-2020-316984.
- Zhang G, et al. Clinically relevant deep learning for detection and quantification of geographic atrophy from optical coherence tomography: a model development and external validation study. Lancet Digit Heal. 2021; 3 (10): e665–e675. DOI: 10.1016/ S2589-7500(21)00134-5.
- Sousa JA, Paiva A, Silva A, Almeida JD, Braz Junior G, Diniz JO, et al. Automatic segmentation of retinal layers in OCT images with intermediate age-related macular degeneration using U-Net and DexiNed. Plos one. 2021; 16 (5): e0251591.
- Alsaih Khaled, et al. Deep learning architectures analysis for age-related macular degeneration segmentation on optical coherence tomography scans. Computer methods and programs in biomedicine. 2020; 195: 105566.
- Lee B, D'Souza M, Singman EL, Wang J, Woreta FA, Boland MV, et al. Integration of a physician assistant into an ophthalmology consult service in an academic setting. American journal of ophthalmology. 2018; 190: 125–33. Available from: https://www.sciencedirect.com/science/article/abs/pii/S0002939418301387.
- Pandey SK, Sharma V. Robotics and ophthalmology: Are we there yet? Indian Journal of Ophthalmology. 2019; 67 (7): 988. Available from: https://www.ncbi.nlm.nih.gov/pmc/articles/PMC6611303/.
- Yan Y, Jin K, Gao Z, Huang X, Wang F, Wang Y, et al. Attention — based deep learning system for automated diagnoses of age– related macular degeneration in optical coherence tomography images. Medical Physics. 2021; 48 (9): 4926–34. DOI: 10.1002/ MP.15002.
- Treder M, Lauermann JL, Eter N. Automated detection of exudative age-related macular degeneration in spectral domain optical coherence tomography using deep learning. Graefe's Archive for Clinical and Experimental Ophthalmology. 2017; 256 (2): 259–65. DOI: 10.1007/S00417-017-3850-3.
- Bhatia KK, Graham MS, Terry L, Wood A, Tranos P, Trikha S, et al. Disease classification of macular optical coherence tomography scans using deep learning software: validation on independent, multicenter data. Retina. 2020; 40 (8): 1549–57. DOI: 10.1097/ IAE.0000000000002640.
- Tvenning AO, Hanssen SR, Austeng D, Morken TS. Deep learning identify retinal nerve fibre and choroid layers as markers of age– related macular degeneration in the classification of macular spectral–domain optical coherence tomography volumes. Acta Ophthalmologica. 2022. DOI: 10.1111/AOS.15126.
- Sunija AP, Kar S, Gayathri S, Gopi VP, Palanisamy P. Octnet: A lightweight cnn for retinal disease classification from optical coherence tomography images. Computer methods and programs in biomedicine. 2021; 200: 105877. DOI: 10.1016/J. CMPB.2020.105877.
- Juba B, Le HS. Precision-recall versus accuracy and the role of large data sets. In Proceedings of the AAAI conference on artificial intelligence. 2019; 33 (01): 4039–48. DOI: 10.1609/AAAI. V33I01.33014039.
- Lowe DG. Distinctive image features from scale-invariant keypoints. International journal of computer vision. 2004; 60 (2): 91–110. Available from: https://link.springer.com/article/10.1023/ B:VISI.0000029664.99615.94
- Shahedi MB, Amirfattahi R, Azar FT, Sadri S. Accurate breast region detection in digital mammograms using a local adaptive thresholding method. In Eighth International Workshop on Image Analysis for Multimedia Interactive Services (WIAMIS'07). IEEE. 2007; 26–26. Available from: https://ieeexplore.ieee.org/abstract/document/4279134.