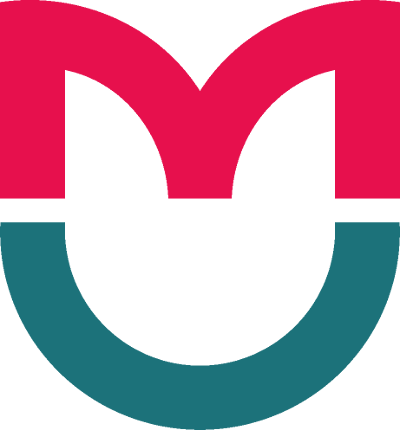
This article is an open access article distributed under the terms and conditions of the Creative Commons Attribution license (CC BY).
ORIGINAL RESEARCH
Algorithm of segmentation of OCT macular images to analyze the results in patients with age-related macular degeneration
1 Bashkir State Medical University, Ufa, Russia
2 Ufa State Aviation Technical University, Ufa, Russia
3 Optimedservice, Ufa, Russia
Correspondence should be addressed: Rada R. Ibragimova
Lenina, d. 3, Ufa, 450008, Russia; ur.xednay@6102adar.avomigarbi
Financing: the study was partially conducted as part of the State Assignment of the Ministry of Education and Science of the Russian Federation for the Ufa State Aviation Technical University (code of scientific assignment #FEUE-2021-0013, agreement № 075-03-2021-014) at the scientific research laboratory named ‘Sensor systems based on appliances of integrated photonics’ (sections ‘Materials and methods’, ‘Study results’, ‘Discussion of results’) and as part of the project backed by subsidies in the area of science taken from the budget of the Republic of Bashkortostan to ensure state support of scientific research conducted under the guidance of the leading scientists (НОЦ-РМГ-2021, agreement with the Ufa State Aviation Technical University) (Introduction section).
Author contribution: Ibragimova RR — review of literature, data acquisition and analysis, writing an article; Gilmanov II — development of software, searching a database, testing the existing code components; Lopukhova EA — development of software, writing an article, data acquisition and analysis; Lakman IA, Mukhamadeev TR, Kutluyarov RV — study concept and design, scientific editing; Bilyalov AR — scientific editing; Idrisova GM — data analysis, scientific editing.
Compliance with ethical standards: the study was performed in accordance with the principles of Declaration of Helsinki; all patients signed voluntary informed consent to OCT.
- Zapata MA, Royo-Fibla D, Font O, Vela JI, Marcantonio I, Moya-Sánchez EU, et al. Artificial intelligence to identify retinal fundus images, quality validation, laterality evaluation, macular degeneration, and suspected glaucoma. Clinical Ophthalmology (Auckland, NZ). 2020; 14: 419. Available from: https://www.ncbi. nlm.nih.gov/pmc/articles/PMC7025650/.
- Stark K, Olden M, Brandl C, Dietl A, Zimmermann M E, Schelter SC, et al. The German AugUR study: study protocol of a prospective study to investigate chronic diseases in the elderly. BMC geriatrics. 2015; 15 (1): 1–8. Available from: https://link.springer. com/article/10.1186/s12877-015-0122-0.
- Mehta S. Age-related macular degeneration. Primary Care: Clinics in Office Practice. 2015; 42 (3): 377–91. Available from: https:// www.primarycare.theclinics.com/article/S0095-4543(15)00042-1/fulltext.
- Lawrenson JG, Evans JR, Downie LE. A critical appraisal of national and international clinical practice guidelines reporting nutritional recommendations for age-related macular degeneration: are recommendations evidence-based? Nutrients. 2019; 11 (4): 823. Available from: https://www.mdpi.com/20726643/11/4/823.
- Li JQ, Welchowski T, Schmid M, Mauschitz M M, Holz FG, Finger RP. Prevalence and incidence of age-related macular degeneration in Europe: a systematic review and meta-analysis. British Journal of Ophthalmology. 2020; 104 (8): 1077–84. Available from: https:// bjo.bmj.com/content/104/8/1077.abstract.
- Vsemirnyj doklad o problemax zreniya [World report on vision]. Zheneva: Vsemirnaya organizaciya zdravooxraneniya, 2020. Licenziya: CC BY-NC-SA 3.0 IGO.
- Balashevich LI, Izmajlov AS, Ulitina AYu. Modificirovannaya klinicheskaya klassifikaciya vozrastnoj makulyarnoj degeneracii. Oftal'mologicheskie vedomosti. 2011; 4 (4): 41–47. Available from: https://cyberleninka.ru/article/n/modifitsirovannayaklinicheskaya-klassifikatsiya-vozrastnoy-makulyarnoydegeneratsii. Russian.
- Avdeeva ON, Avetisov SEh, Aklaeva NA, Akopov EL, Alekseev VN, Astaxov SYu, i dr. redaktory. Oftal'mologiya: nacional'noe rukovodstvo. M.: GEhOTAR-Media, 2018; 625 s. Russian.
- Ferris III FL, Wilkinson CP, Bird A, Chakravarthy U, Chew E, Csaky K, et al. Beckman Initiative for Macular Research Classification Committee. Clinical classification of age-related macular degeneration. Ophthalmology. 2013; 120 (4): 844–51. Available from: https://www.sciencedirect.com/science/article/ abs/pii/S016164201201055X.
- Hyttinen JM, Kannan R, Felszeghy S, Niittykoski M, Salminen A, Kaarniranta K. The regulation of NFE2L2 (NRF2) signalling and epithelial-to-mesenchymal transition in age-related macular degeneration pathology. International journal of molecular sciences. 2019; 20 (22): 5800. Available from: https://www.mdpi. com/1422-0067/20/22/5800.
- Friedman DS, O’Colmain BJ, Munoz B, Tomany SC, McCarty C, De Jong Pt, et al. Prevalence of age-related macular degeneration in the United States. Arch ophthalmol. 2004; 122 (4): 564– 72. Available from: https://jamanetwork.com/journals/ jamaophthalmology/article-abstract/416232.
- Schultz NM, Bhardwaj S, Barclay C, Gaspar L, Schwartz J. Global Burden of Dry Age-Related Macular Degeneration: A Targeted Literature Review. Clin Ther. 2021; 43 (10): 1792–818. DOI: 10.1016/J.CLINTHERA.2021.08.011.
- The Age-Related Eye Disease Study Research Group. A randomized, placebocontrolled, clinical trial of supplementation with vitamins C and E and beta-carotene for age related cataract and vision loss: AREDS report number 9. Arch. Ophthalmol. 2001; 119: 1439–52.
- Varma R, Bressler NM, Doan QV, Danese M, Dolan CM, Lee A, et al. Visual impairment and blindness avoided with ranibizumab in Hispanic and non-Hispanic whites with diabetic macular edema in the United States. Ophthalmology. 2015; 122 (5): 982– 89. Available from: https://www.sciencedirect.com/science/ article/pii/S0161642014011476.
- Aznabaev BM, Muxamadeev TR, Dibaev TI. Opticheskaya kogerentnaya tomografiya + angiografiya glaza v diagnostike, terapii i xirurgii glaznyx boleznej. M.: Avgust Borg, 2019; 57 s. Russian.
- Drexler W, Fujimoto JG, editors. Optical coherence tomography: technology and applications. Berlin: Springer, 2015; 2.
- Victor AA. The Role of Imaging in Age-Related Macular Degeneration. In Visual Impairment and Blindness-What We Know and What We Have to Know. London, UK: IntechOpen, 2019.
- Schmidt-Erfurth U, Sadeghipour A, Gerendas BS, Waldstein SM, Bogunović H. Artificial intelligence in retina. Progress in retinal and eye research. 2018; 67: 1–29. Available from: https://www.sciencedirect.com/science/article/pii/S1350946218300119.
- Venhuizen FG, van Ginneken B, van Asten F, van Grinsven MJ, Fauser S, Hoyng CB, et al. Automated staging of age-related macular degeneration using optical coherence tomography. Investigative ophthalmology & visual science. 2017; 58 (4): 2318–28. Available from: https://iovs.arvojournals.org/article. aspx?articleid=2623584.
- Shlyapnikova OA, Kamenskix TG, Roshhepkin VV, Reshnikova LB. Perspektivnye napravleniya razvitiya oftal'mologii (obzor). Saratovskij nauchno-medicinskij zhurnal. 2021; 17 (3): 675–8. Available from: https://cyberleninka.ru/article/n/perspektivnye-napravleniya-razvitiya-oftalmologii-obzor. Russian.
- Quellec G, Lee K, Dolejsi M, Garvin MK, Abramoff MD, Sonka M. Three-dimensional analysis of retinal layer texture: identification of fluid-filled regions in SD-OCT of the macula. IEEE transactions on medical imaging. 2010; 29 (6): 1321–30. Available from: https://ieeexplore.ieee.org/abstract/document/5440910.
- Hu Z, Niemeijer M, Abramoff MD, Garvin MK. Multimodal retinal vessel segmentation from spectral-domain optical coherence tomography and fundus photography. IEEE transactions on medical imaging. 2012; 31 (10): 1900–11. Available from: https:// ieeexplore.ieee.org/abstract/document/6228540.
- Esmaeili M, Dehnavi AM, Rabbani H, Hajizadeh F. Threedimensional segmentation of retinal cysts from spectral-domain optical coherence tomography images by the use of threedimensional curvelet based K-SVD. Journal of medical signals and sensors. 2016; 6 (3): 166. Available from: https://www.ncbi.nlm.nih.gov/pmc/articles/PMC4973460/.
- Chakravarty A, Sivaswamy J. A supervised joint multi-layer segmentation framework for retinal optical coherence tomography images using conditional random field. Comput. Methods Programs Biomed. 2018; 165: 235–50. DOI: 10.1016/J. CMPB.2018.09.004.
- Bogunović H, et al. Machine Learning of the Progression of Intermediate Age-Related Macular Degeneration Based on OCT Imaging. Investigative ophthalmology & visual science. 2017; 58 (6): BIO141–BIO150.
- Tvenning AO, Hanssen SR, Austeng D, Morken TS. Deep learning identify retinal nerve fibre and choroid layers as markers of agerelated macular degeneration in the classification of macular spectral‐domain optical coherence tomography volumes. Acta Ophthalmologica. 2022. DOI: 10.1111/AOS.15126.
- Rim TH, et al. Detection of features associated with neovascular age-related macular degeneration in ethnically distinct data sets by an optical coherence tomography: trained deep learning algorithm. British Journal of Ophthalmology. 2020; 105 (8): 1133–9. DOI: 10.1136/BJOPHTHALMOL-2020-316984.
- Zhang G, et al. Clinically relevant deep learning for detection and quantification of geographic atrophy from optical coherence tomography: a model development and external validation study. Lancet Digit Heal. 2021; 3 (10): e665–e675. DOI: 10.1016/ S2589-7500(21)00134-5.
- Sousa JA, Paiva A, Silva A, Almeida JD, Braz Junior G, Diniz JO, et al. Automatic segmentation of retinal layers in OCT images with intermediate age-related macular degeneration using U-Net and DexiNed. Plos one. 2021; 16 (5): e0251591.
- Alsaih Khaled, et al. Deep learning architectures analysis for age-related macular degeneration segmentation on optical coherence tomography scans. Computer methods and programs in biomedicine. 2020; 195: 105566.
- Lee B, D'Souza M, Singman EL, Wang J, Woreta FA, Boland MV, et al. Integration of a physician assistant into an ophthalmology consult service in an academic setting. American journal of ophthalmology. 2018; 190: 125–33. Available from: https://www.sciencedirect.com/science/article/abs/pii/S0002939418301387.
- Pandey SK, Sharma V. Robotics and ophthalmology: Are we there yet? Indian Journal of Ophthalmology. 2019; 67 (7): 988. Available from: https://www.ncbi.nlm.nih.gov/pmc/articles/PMC6611303/.
- Yan Y, Jin K, Gao Z, Huang X, Wang F, Wang Y, et al. Attention — based deep learning system for automated diagnoses of age– related macular degeneration in optical coherence tomography images. Medical Physics. 2021; 48 (9): 4926–34. DOI: 10.1002/ MP.15002.
- Treder M, Lauermann JL, Eter N. Automated detection of exudative age-related macular degeneration in spectral domain optical coherence tomography using deep learning. Graefe's Archive for Clinical and Experimental Ophthalmology. 2017; 256 (2): 259–65. DOI: 10.1007/S00417-017-3850-3.
- Bhatia KK, Graham MS, Terry L, Wood A, Tranos P, Trikha S, et al. Disease classification of macular optical coherence tomography scans using deep learning software: validation on independent, multicenter data. Retina. 2020; 40 (8): 1549–57. DOI: 10.1097/ IAE.0000000000002640.
- Tvenning AO, Hanssen SR, Austeng D, Morken TS. Deep learning identify retinal nerve fibre and choroid layers as markers of age– related macular degeneration in the classification of macular spectral–domain optical coherence tomography volumes. Acta Ophthalmologica. 2022. DOI: 10.1111/AOS.15126.
- Sunija AP, Kar S, Gayathri S, Gopi VP, Palanisamy P. Octnet: A lightweight cnn for retinal disease classification from optical coherence tomography images. Computer methods and programs in biomedicine. 2021; 200: 105877. DOI: 10.1016/J. CMPB.2020.105877.
- Juba B, Le HS. Precision-recall versus accuracy and the role of large data sets. In Proceedings of the AAAI conference on artificial intelligence. 2019; 33 (01): 4039–48. DOI: 10.1609/ AAAI.V33I01.33014039.
- Lowe DG. Distinctive image features from scale-invariant keypoints. International journal of computer vision. 2004; 60 (2): 91–110. Available from: https://link.springer.com/article/10.1023/ B:VISI.0000029664.99615.94
- Shahedi MB, Amirfattahi R, Azar FT, Sadri S. Accurate breast region detection in digital mammograms using a local adaptive thresholding method. In Eighth International Workshop on Image Analysis for Multimedia Interactive Services (WIAMIS'07). IEEE. 2007; 26–26. Available from: https://ieeexplore.ieee.org/abstract/document/4279134.