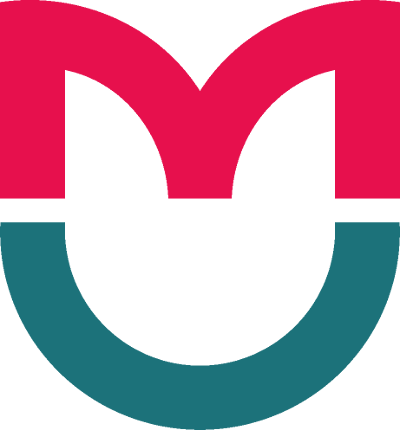
ORIGINAL RESEARCH
Studying the ability to control human phantom fingers in P300 brain-computer interface
1 Laboratory for Neurophysiology and Nero-Computer Interfaces, Faculty of Biology,Lomonosov Moscow State University, Moscow, Russia
2 Laboratory for Brain-Machine Interfaces and Applied Neuroengineering,Lobachevsky National Research State University of Nizhny Novgorod, Nizhny Novgorod, Russia
Correspondence should be addressed: Alexandr Kaplan
Leninskie gory, d. 1, str. 12, Moscow, Russia, 117234; ur.liam@nalpaka
Funding: this work was partially supported by the Skolkovo Foundation, grant no. 1110034, and the Russian Science Foundation, grant no. 15-19-20053.
Including the elements of mental practice into rehabilitation programs for patients with impaired motor skills after stroke or neurological trauma is becoming a new trend in modern neurorehabilitation [1, 2, 3, 4]. This approach is based on Nikolai Bernstein’s concept according to which creating a mental plan for executing the motor act rather than performing the act itself would be a true response to the tasks set by the environment [5]. Such activity boosts neural plasticity processes that drive restoration of neuronal pools for motor control [6, 7]. Motor imagery that triggers restructuring of the motor act plan in neuronal networks can be as effective for the restoration of impaired motor coordination as the actual execution of the movement [1, 3, 4]. Indeed, the studies based on transcranial magnetic stimulation used to test cortical excitability demonstrated the activation of cortical structures associated with motor representation during motor imagery [8, 9].
However, despite the seeming simplicity of motor imagery, whether it is effective in triggering cortical restructuring depends on mental effort intensity, stability and direction [10, 11]. Yet, an individual needs a feedback on motor imagery quality, otherwise his or her mental effort will weaken if repeated multiple times, motor images will fade, and he or she will gradually lose interest in the training procedure. The feedback loop can be provided by brain-computer interface (BCI) technologies that make use of mu-rhythm depression recorded by EEG to detect mental representations of movements and thansform those events into commands for controlling virtual reality objects or real objects [2, 9, 10, 12]. Thus, the operator’s mental effort shaped as movement representation can be translated into the actions of physical objects or virtual objects on the screen via BCI. Given a person is motivated, his or her motor imagery skills can develop sufficient intensity and sustainability over time. Using such skills during training sessions is an effective trigger for adaptive plasticity processes in the corresponding brain structures [1, 9, 12].
The weakness of this approach is the extremely low level of differentiation of mental movement representations in relation to their subsequent BCI-based identification. Only 2 to 3 motor images can be reliably (with 0.6–0.7 probability) identified by motor imagery based BCIs. Usually, those are left or right arm/ leg movements [10, 12]. It is insufficient for establishing several feedback channels for mental rehearsal of fine motor skills, such as movements of individual fingers, which are the hardest to restore.
At the same time, there is a BCI technology that makes use of reliable EEG-based detection of human focus of attention on external screen characters and provides for a library of no less than 36 commands [13]. Detection of the attention focus is based on EEG responses to short flashes of external objects, such as symbols on the screen; response to a target stimulus is identified on the basis of specific response parameters, a P300 wave in particular [1, 14, 15]. Still, due to the necessity of using a stimulus medium formed by symbols, application of BCI-P300 in rehabilitation could be reduced to communicators, such as for text entry in patients with severe motor and speech impairment, and for activation of remote control buttons [16].
In this work we test the hypothesis that BCI-P300 can be used non-conventionally, namely, as a basis for a training simulator for improving fine motor skills (of fingers) with a multichannel feedback. In such a simulator, the anthropomorphic hand phantom with movable fingers can be used as an actuator. We speculate that using a BCI-P300 system proposed in this work, an individual will be able to control phantom finger flexion by focusing his or her attention on the fingers.
The onset of finger movement will indicate sufficient intensity of mental effort aimed at focusing attention on the process.
METHODS
The study enrolled 12 right-handed volunteers (6 male and 15 female, 18–25 years of age) with normal or corrected-to-normal vision. The study was approved by Bioethics Committee of Lomonosov Moscow State University. All participants gave written informed consent. The subjects were seated in a comfortable armchair, arms on armrests. Above the right arm of the subject covered with non-transparent fabric, the anthropomorphic hand was placed, its movable fingers were connected to servo-motors by flexible cords. Light markers (light-emitting diodes) were attached to the distal phalanx of each phantom finger, light intensity being 5 cd/m2. Turning them on and off was a visual stimulus for event-related potentials (ERPs) recorded by EEG.
For unipolar EEG recording, 8 electrodes (Cz, Pz, PO3, PO4, PO7, PO8, O1, O2), the referential indifferent ear electrode and the Ground Electrode in Fpz position were used. To record biopotentials, NVX52 electroencephalograph (MKS, Russia) was set up to a sampling frequency of 500 Hz, a passband of 0.1–30 Hz (second order Butterworth filter), a band-reject filter of 50 Hz.
To identify ERPs associated with target stimuli, i. e., flashes of the light markers on the phantom finger that the subject’s attention was focused on, a classifier based on Fisher's linear discriminant analysis (LDA) was used; its output was transformed into a finger-flexion command for the phantom if the preset threshold was exceeded. The classifier analyzed short intervals of 10 ms long that the 0–800 ms EEG signal was split into (0–800 ms is time from stimulus presentation). Commands for stimuli and servomotors activation were executed by phantom hand components, namely, two programmed microcomputers Freeduino Nano v5ATmega328 (Russia). Both microcomputers were connected to the computer via USB ports. The phantom was connected to NVX52 amplifier for EEG recording, which facilitated synchronized ERP recording, their processing by the classifier and command generation.
During the experimental session, a randomized sequence of ten flashes on each phantom finger was presented to the subject to implement one command, i. e., to flex one phantom finger that the subject’s attention was drawn to by flashes. Each command was preceded by instructing the subject on what phantom finger had to be chosen. Light-emitting diodes were on for 50 ms; the interval between the stimuli was 150 ms. Every subject had 20 experimental sessions spaced by small breaks; their results were used to assess how effectively the subject operated the hand phantom.
The classifier was trained right before the experimental session on non-random sampling sets of target and non-target ERPs. The procedure lasted for 4 min.
To estimate which operating mode was the most effective for attention focus control, two types of signals were tested, with subject’s attention focused on flash offset and flash onset. In both cases the length of the signal was set to 50 ms.
To assess how effectively the phantom fingers were operated, control accuracy was measured by the number of right, wrong or zero phantom finger flexions resulting from the subject focusing the attention on a certain phantom finger. Statistical analysis of data obtained from all subjects was carried out using Stasoft Statistica 7.0 software. To estimate the difference in the effectiveness of both operating modes Wilcoxon sign ranked test was used.
RESULTS
Fig. 1 shows statistical data on subjects’ performance accuracy during the procedure of selecting a target phantom finger for its further flexion by shifting the focus of attention to it. The data are presented as a percentage of the total number of trials. The accuracy rates of control of phantom fingers were averaged across the groups for various operating modes (using flash onset and offset as stimuli). The results did not show any statistical difference and were 69 % and 57 % , respectively, with maximal control accuracy being 95 % for some subjects in both modes. The range of accuracy scores for each subject in the second mode was substantially wider than in the first mode. It leads us to conclude that using BCI-P300 technology with light markers placed on phantom fingers controlled via BCI can be seen as a basis for developing a neurosimulator for fine motor skills, with a customized set-up of light signaling for each operating mode.
As shown in fig. 1, the subjects could not issue a command to flex the target finger by shifting their attention to the light marker placed on the phantom hand even in the optimal operating mode. In case of errors, either a non-target finger is flexed or no movement occurs; therefore, analysis of errors of both types was carried out; its results are presented in fig. 2. Scores are presented as absolute values of the number of errors of both types that occurred during 20 attempts to initiate target finger flexion. It should be reminded that each trial could result in one right and five wrong responses (four non-target finger responses and one zero movement response).
As shown in fig. 2, subjects rarely failed to select the target finger for flexion. In average, there were no more than 1.5 errors in 20 trials. But in a greater number of cases (5–6 depending on the operating mode), subjects failed to initiate flexion of any finger leaving the phantom hand motionless. Wilcoxon test showed significant differences (p < 0.05) between the number of type 1 and type 2 errors for each operating mode.
Initiating a non-target finger movement is possibly related to the weak sustained attention; attention is drawn to the non-target finger, and the latter is wrongly detected as a flexion target. At the same time, the absence of commands for phantom fingers on completing another attempt to activate the target finger indicates insufficient attention focus on flashing events. As a result, no distinct ERPs are generated in response to target stimuli, and classifier output does not reach the threshold value for command issue.
DISCUSSION
The obtained data confirmed that it is possible to design a BCI-P300 — Phantom Hand system, in which an individual can control flexion of phantom fingers by voluntarily focusing his or her attention on them. Because flexion of a finger that an individual selects in his mind indicates the sufficient intensity of his mental effort to focus his attention on triggering the movement, development of the effective training simulator for fine motor skills that makes use of this technology is highly possible.
However, up to now no attempts to use a BCI-P300 technology for feedback in motor function training have been reported. It is probably due to the fact that the operator does not need to perform motor imagery or his attention is shifted to external objects
However, since pivotal works of Botvinnik and Cohen [17] were published, their findings confirmed multiple times thereafter [18, 19], it has been known that under certain conditions the external object, such as a rubber hand, can be easily and reliably identified with the internal representation of one’s own hand. Moreover, there is no need to imagine the movement of one’s own hand to activate the neurons in the motor cortex, it is enough to watch the hand of the other person or its artificial replica move [20, 21]. Coupled with the data obtained in this work confirming the possibility of using the BCI-P300 technology for controlling individual fingers of the hand phantom, the facts mentioned above hold promise for creating a fine motor skills training neurosimulator based on the BCI-P300 — Phantom Hand system.
CONCLUSIONS
The BCI-P300 technology can be used to generate commands for mental control of fingers of the human hand phantom with reliability of no less than 69 %, which is sufficient to develop a fine motor skills neurosimulator.
The majority of the BCI-P300 operator’s errors in controlling the fingers of the human hand phantom are associated with insufficient focus on the signals of the light markers placed on phantom fingers, which necessitates the improvement of the stimulus medium.