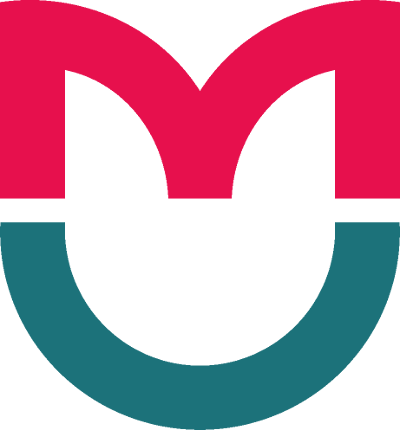
ORIGINAL RESEARCH
Adapting the p300 brain-computer interface technology to assess condition of anorexia nervosa patients
1 Faculty of Biology, Lomonosov Moscow State University, Moscow, Russia
2 Scientific-practical Children's and Adolescents Mental Health Center n.a. G. Sukhareva, Moscow, Russia
3 Pirogov Russian National Research Medical University, Moscow, Russia
Correspondence should be addressed: Ilya P. Ganin
Leninskie gory 1, bl. 12, ap. 246, Moscow, 119234; ur.liam@ninagpi
Author contribution: all authors participated in the experiment planning; Ganin IP — immediate research activities, data analysis and interpretation, literature analysis, manuscript authoring; Kosichenko EA — immediate research activities, literature analysis, data analysis; Sokolov AV — immediate research activities, data interpretation, text editing; Ioannisyanc OM — diagnosing and selection of patients for the study; Arefev IM — support of experiments, data interpretation; Basova AYa — data interpretation, text editing; Kaplan AYa — data interpretation.
Brain-computer interface technology (BCI) is currently being accepted as a standard research tool by more and more neurophysiology laboratories in the world [1]. BCI is a system developed and designed to enable people with lost or damaged motor functions to command external devices by decoding specific electroencephalogram (EEG) patterns [2]. There is a high demand for such interfaces in medicine: they are used to control auxiliary units [3, 4], for rehabilitation purposes [5], and also for communication [6]. Therefore, BCI is the subject of many research efforts.
At its current level, in certain conditions BCI allow highly accurate interpretation of the operator's commands as analyzed from EEG, which signals of the possible extension of the technology's potential sphere of application. First of all, the approaches and algorithms used in BCI can be used not only to recognize specific commands resulting from an arbitrary concentration of attention, but also to detect implicit foci of attention to external stimuli or one’s own internal states. Such involuntary shifts of attention or, on the contrary, absence of attention markers in the EEG readings under certain conditions, can help diagnose various mental disorders in the context of instrument-assisted examinations.
There is a number of BCI versions that would allow designing a system for this purpose; the most appropriate of them is the interface enabling interpretation of the P300 wave, the P300 BCI [2, 6, 7], which offers external stimuli-commands and thus enables its operator to select commands. Determining such commands takes sequential activation of the stimuli (e.g., flashing letters on a display) and analysis of the response thereto. If the amplitude of the event-related potentials (ERP) is high, the stimuli is considered to be important for the operator, which translates into selection of the corresponding command. P300, a well-studied wave, is the main component of ERP: its amplitude is highest in response to rare and important stimuli.
EEG registers a specific response to an external stimulus in case the BCI user actively concentrates on the relevant command. However, the P300 wave and other EEG-detectable signs of attention to an event may be caused by stimuli that attract the person's attention indirectly, i.e. they are not immediately and clearly interesting to that person but probably possess some significance due to subjective experience, current psycho-emotional status [8, 9], which justifies the involuntary attention. Thus, the P300 BCI stimuli presentation paradigm also allows deriving the peculiarities of perception of specific information from the person's EEG readings, as well as detecting the focus of implicit interest to the certain classes of external stimuli. The patients would not have to react to a specific class of stimuli; rather, such a system would simply require them to look at the presented sequences of stimuli [10], thus exploiting the so-called passive attention paradigm. The feasibility of such an approach is based on the fact that even without a conscious response to a stimulus, biological or emotional, it can anyway trigger a reaction [11] because it is of significance to the person. Therefore, for the BCI purposes such a stimulus can be considered a "target" stimulus, which allows further classification based on the analysis of EEG readings.
The most logical option for such examination methods is to use emotional content in a mix with neutral content [12]. The systems making use of these methods would automatically detect overexcitement in people whose job implies high emotional load [13]. They would also enable instrument-assisted diagnosing of the emotion perception disorders, such as autism [14, 15].
Applying the methods exploiting P300 BCI to assess the state of patients with eating disorders is as interesting. In particular, they can be shown a number of visual stimuli related to anorexia, and their EEG readings, analyzed, can serve as an additional indicator of treatment effectiveness. Earlier, it was shown that anorexia nervosa patients fail to perceive emotional stimuli normally [16], and that failure is EEG-detectable. Besides, the P300 wave and other ERP components registered in such patients feature some specific characteristics if recorded when they solve a task requiring concentration of attention, which signals of deterioration of some brain functions [17].
Some previous research efforts aimed to identify the peculiarities of EEG readings resulting from presentation of visual stimuli in various conditions. However, to date, there is no prototype of a system that would recognize the focus of interest to certain classes of stimuli based on the EEG signal. Therefore, this study aimed to find the specific features of ERP triggered by presenting the stimuli of varying emotional value to anorexia nervosa patients whose attention is in the passive mode, as well as to validate application of P300 BCI to detect the focus of attention to subjectively important stimuli.
METHODS
Twelve adolescent girls (11–16 years old), patients at the Scientific-practical Children's and Adolescents Mental Health Center n.a. G. Sukhareva, participated in the study. The inclusion criteria were: diagnosed anorexia nervosa (diagnosis F50.0); active rehabilitation. The exclusion criteria were: severe somatic pathology; high degree of protein and energy deficiency; strict bed rest. We recorded EEG of the participants while they were looking at the screen showing sets of photographic images.
The angular dimensions of the images were 12.9 × 9.6°; they were presented against a gray background following the oddball paradigm: the stimuli appeared sequentially in the center of the screen, which remained empty otherwise. The stimuli were shown for 200 ms, the interval between them was 500 ms. There were two types of images: pictures of food and body parts of emaciated people. As for the neutral (“insignificant”) stimuli, they were images of objects, animals, geometric figures, landscapes, etc., taken from the IAPS database [18], which was also the source of some images of food. The majority of the significant stimuli was selected following the analysis of online channels covering anorexia. Before being shown to the patients, all images were assessed by the doctor that treated them. The sets included 6 images, 5 insignificant and 1 significant. Each set of stimuli presented was covered by a single EEG recording lasting less than a minute; the recordings were separated by short pauses. The presentation took form of the sequences of stimuli, each showing the images once, in a random order. One EEG recording consisted of 10 sequences of stimuli. Each participant received the sets in a pseudo-random order; the records with the stimuli (food and body parts) were rotated. The total number of "food" and "body parts" stimuli records usually equaled 10 and 12, respectively (each patient).
The EEG registration was monopolar, sites Cz, P3, Pz, P4, PO7, PO8, O1, O2, reference electrode on the lobe of the left ear. We used the NVX52 amplifier with a sampling frequency of 500 Hz. BCI2000 software (www.bci2000.org) enabled data recording and stimuli presentation control.
MATLAB 7.1 (MathWorks; USA) was used to isolate and analyze ERP. We bandpass filtered the EEG signal in the range of 0.5–20 Hz (Butterworth filter), and then divided it into epochs relative to the moment the stimulus was presented with boundaries from 0.1 to 0.7 s. After removing the epochs containing oculomotor artifacts from the array, we divided the epochs into target ("significant") and non-target ("insignificant").
The type of the image (food or body part) was another criterium for division. The number of target and non-target epochs was equalized through eliminating some of the latter. The epochs for target and non-target stimuli were equalized separately. Thus, for each participant we received target and non-target ERP in all EEG channels and in two blocks. Besides, the difference ERP were determined through subtracting non-target curves from target curves.
The amplitudes of P300, N1 and LPP components were measured as a maximum or a minimum value in time window selected individually for each participant. The P300 component amplitudes were measured in the Cz and Pz channels, those of the N1 and LPP components — in channels PO7, PO8, O1 and O2. Not all participants had the entire range of components registered in all channels, therefore the results contain the number of patients (n) that underwent this or that analysis.
Using the Fisher's linear discriminant, we modeled an approach to classification of the P300 BCI target commands with the aim to assess the effectiveness of EEG-enabled identification of special responses to significant stimuli. The classifier learned and was tested through cross-validation of two classes, target and non-target stimuli. In each testing attempt, the classifier was tasked with identifying one most prominent stimulus out of six. If the identified stimulus was significant, the attempts was deemed successful.
Based on the ERP amplitude calculations and the level of accuracy of classification, we calculated the averages of each value in both blocks by processing the data in STATISTICA 7.0 software (StatSoft; USA). When the samples distribution was normal, we used the paired samples t-tests, when it was not — Wilcoxon signed-rank test.
RESULTS
The individual difference curves of the majority of anorexia nervosa patients had peaking N1 (latency 120–180 ms), P300 (350–450 ms), and LPP (550–700 ms). These peaks are also seen on the target and non-target ERP averaged over the entire group, as well as on difference curves (fig. 1). The ratio of amplitudes of target and non-target ERP seen on the difference curves allows deducing the intensity with which significant stimuli attracted attention compared to insignificant stimuli. Since the individual latency of peaks varied and not all participants had all peaks in their EEG readings, some ERP components in the averaged picture appear blurred and poorly pronounced, so fig. 1 is the reflection of the situation on the whole. Table below contains the average values of all analyzed components in the target, non-target and difference curves.
In both blocks, the amplitude of the P300 component was higher on target curves than on non-target ones both in the Cz (p < 0.01; n = 11 and p < 0.01, n = 12, paired samples t-tests) and Pz (p < 0.01, n = 12 and p < 0.01, n = 11) channels, food and body parts images, respectively (table). Comparison of the P300 amplitudes of two difference curves describing two types of stimuli did not reveal significant differences (fig. 2).
The N1 component target ERP amplitudes were higher than non-target for both food images (p < 0.05, n = 9; p < 0.05, n = 10; p < 0.05, n = 9; p < 0.05, n = 11, in sites PO7, PO8, O1, O2, respectively, paired samples t-tests) and images of body parts (p < 0.01, n = 9; p < 0.01, n = 12; p < 0.01, n = 12; p < 0.01, n = 11, Wilcoxon test). Despite the fact that body part images triggered higher average N1 difference curves amplitudes than food images (fig. 1 and table), only P08 site has shown significant differences between the two types of stimuli (p < 0.05, n = 10; Wilcoxon test) (fig. 2).
The LPP component had the greatest amplitude in the occipital sites. Its target ERP amplitudes were higher than non-target for both food images (p < 0.01, n = 8; p < 0.01, n = 9; p < 0.05, n = 8; p < 0.01, n = 9, in sites PO7, PO8, O1, O2, respectively, paired samples t-tests) and images of body parts (p < 0.01, n = 9; p < 0.01, n = 10; p < 0.01, n = 10; p < 0.01, n = 10). On average, the images of body parts caused higher LPP amplitudes than food images, however, the differences were significant only at sites PO7 and PO8 (p < 0.05, n = 7; p < 0.05, n = 8, respectively) (fig. 2).
Figure 3 shows the average accuracy of significant stimuli classification among the neutral ones (both blocks). The average classifier recognition accuracy for the food image stimuli was 59.1 ± 5.3%, which is lower than that for the emaciated body parts images that equaled 89.1 ± 2.3% (p = 0.0002, n = 12, paired samples t-tests).
DISCUSSION
The main result of this study is identification of a number of features of ERP peculiar to passive perception of emotionally significant images, as well as the proven possibility to use EEG readings to detect attention to such stimuli when mixed with neutral content, the accuracy of such detection being close to that of the current BCI systems.
It is known that people running a high risk of eating disorders are sensitive to emotionally significant stimuli associated with the body shape weight [19]. This level of sensitivity is subjective; in case of anorexia nervosa, it can be explained by the special attitude the patients have towards their bodies, including development of the dysmorphophobia / dysmorfomania syndrome against the background of low self-esteem [19, 20]. We have discovered that food or emaciated body part images result in higher ERP amplitudes, which means such images are important to the patients, although the intensity of response was different for the two types considered.
Based on the available literature, we could explain the higher ERP amplitude associated with the significant stimuli by the fact that they are presented rarely compared to the neutral stimuli in the mix, i.e., as the oddball paradigm has it, rare target stimuli provoke a more intense response [21]. At the same time, the oddball paradigm we utilized in our study was modified: its classic version implies using two greatly different simple classes of stimuli, whereas we had the stimuli equally heterogeneous both within the significant/insignificant classes and between the classes. This fact suggests that the differences registered in the present study result not from the specific features of the images but solely from the subjective significance of the semantic content of this group of stimuli. Thus, presentation of the images of body parts caused a significantly stronger reaction (as reflected by ERP) than those of food, although both such stimuli were equally likely to be important to the participants. An assumption based thereon is that the stimulus that is unlike the majority of other stimuli shown triggers intense response because it is of special emotional value to the patient and not solely because it is a rare component of the given mix. This assumption is further confirmed by the fact that the P300 wave amplitude that reflects the frequency characteristics of the stimulus was the same for the two types of images, while all other ERP components and classifier accuracy depended on the type of stimulus.
One of the most important results of this work is the extremely high accuracy of classification of subjectively significant images based on the EEG-detectable response: for food images it was 59%, for images of body parts — 89%. It should be noted here that the tasks set before the participants did not require them to actively concentrate on the stimuli shown. This level of accuracy is far higher than the 16.7% random recognition rate, when participants select one of the 6 stimuli at random. Moreover, it is close to the values achieved with the help of P300 BCI, the use of which implies active concentration on the target stimulus-command and disregard of all other stimuli [22, 23].
The research described in this paper is largely a pilot study; its practical value is the effort to adapt the existing BCI approaches to the purposes of development of methods and systems to detect subjective foci of attention. Therefore, one of the limitations of this work is the absence of a control group of healthy subjects. At the same time, we can consider mixes with food images to be the control component compared to mixes with body part images: the two produced different ERP components, although they were equally likely to prove significant to the patients and featured the same set of insignificant stimuli (see above). The results are comparable to those attained by the authors in the context of another recent study that had healthy people performing a similar task: they were passively viewing various images on a screen, the mix featured some that were emotionally different from the rest but not subjectively significant to the participants [12]. The component amplitudes reflecting response to the different stimuli were lower than in this work, and the accuracy of classification was 40–45%. Some data suggest that anorexia nervosa patients respond weaker (as revealed by ERP) to the food-related visual stimuli than healthy people [24]. We have discovered that images of body parts of emaciated people triggered a significantly more intense EEG-detectable response than images of food. This may mean that the latter can attract attention better and are especially important to anorexia nervosa patients.
The N1 component reflects the processes of fixation on a certain stimulus; it is associated with emotional perception [25], which allows deducing the level of attention the patients pay to the images of food and body parts, as well as assuming that the significance of such stimuli is the result of an emotional reaction. The LPP component is associated with the late stages of emotional processing of visual stimuli [26], which is another fact supporting the statement that the special EEG-detectable response to the significant images used herein is the result of actual cognitive processing and not just a reaction to a stimulus that looks out of context. Overall, the higher N1 and LPP component amplitudes together with the high accuracy of classification registered for the emaciated body part images versus food images support the assumption that anorexia can largely be caused by the person's specific attitude to his/her body, faulty perception of its shape and size, obsessive desire to meet certain physical standards, while the actual denial to consume food may be only one of the secondary reasons [19].
CONCLUSIONS
The applied method of identification of involuntary attention to images of food and body parts of emaciated people shown to anorexia nervosa patients allowed receiving highly accurate results of classification of involuntary attention reactions to emotionally significant stimuli. Further development of this method can contribute to the design of systems to detect emotional foci of attention with the help of EEG readings. Such systems could be used to determine peculiarities of the patients' emotional perception (as part of diagnosing their overall condition) at different stages of treatment. We have registered a stronger response to the images of body parts of emaciated people than to the images of food; this result was confirmed by EEG experiments. On the one hand, this can support the known patterns of development of anorexia nervosa, and on the other hand, suggests applying the relevant stimuli materials in the above-mentioned systems.