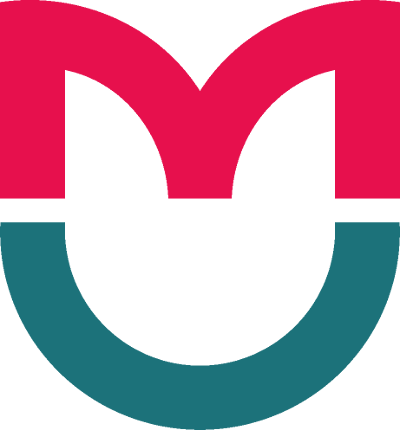
ORIGINAL RESEARCH
Predictive monitoring of secondary epidemic waves of COVID-19 in Iran, Russia and other countries
1 Nizhny Novgorod State Technical University n.a. R.E. Alekseev, Nizhny Novgorod, Russia
2 National Research Lobachevsky State University of Nizhny Novgorod, Nizhny Novgorod, Russia
Correspondence should be addressed: Svetlana P. Nikitenkova
Gagarina, 23, Nizhny Novgorod; 603950; moc.liamg@avoknetikins
Author contribution: Kovriguine DA — research planning, literature analysis, data analysis and interpretation, manuscript preparation; Nikitenkova SP — research planning, literature analysis, data analysis and interpretation, manuscript preparation.
The first epidemic wave in Iran had attracted the attention of observers and researchers by a relatively high rate of overall infections [1–5]. The processing of official statistics to predict the second waves based on a logistic model showed that the first wave was only a harbinger of the spread of a new, higher wave of the epidemic, which had already swept through this country and gave rise to the third wave in early June [6]. (We interpret an epidemic wave as the process either of transferring and spreading the infection to a new territory with a new population or returning to the old places partially or completely have recovered after the primary epidemic.)
Recently, the secondary waves of the epidemic have started in Sweden, the United States, Ukraine, Serbia, Romania, Czech Republic, Ecuador and some other countries. As yet, there are no rigorous mathematical models to shed light, in particular, on those critical points at which the secondary epidemic appears (we understand a strict theory as axiomatics based on the maximum principle. Any epidemic naturally "strives" to occupy the entire territory and cover the entire "available" population).
Today, there are more and more messages in the media about the expectation of second waves of the epidemic in Russia. However, this acute question remains open without a rigorous analysis of the input data.
The purpose of this work is to describe the secondary epidemic waves mathematically. We have chosen a simple dynamic logistic system with the unknown parameters which are determined by the official data (data source: https://covid. ourworldindata.org/). The aim is to identify the most favourable conditions for the occurrence of secondary waves.
METHODS
We use the tabular autocorrelation dependencies Ni + 1 = ƒc(Ni ), to construct the dynamic system. Here, Ni is the number of total cases, accumulated to the date i, in the country number c.
The least-squares method determines the polynomial approximations of tabular functions ƒc, denoted as Fc.
Numerical experiments confirm the sufficiency of the quadratic approximation of functions ƒs from the argument Ni that provides a logistic model. So, we have got the analytic point mapping having the right-hand side
Fc (Ni ) = a0 + a1Ni + a2Ni2,
where aj are the parameters determined from the tables (data source: https://covid.ourworldindata.org/). The transition time between the states Ni and Ni + 1 takes a day. The logistic mapping approaches the steady-state N = N*, as a positive solution to the equation
N* = a0 + a1N* + a2N*2.
The steady-state N* corresponds to almost zero dynamic increase in disease [7]. At the time of achieving this stable equilibrium, the logistic equation is no longer sufficient to describe the evolution of the disease in a developed human society. The fate of infected people depends on the quality of quarantine measures, medical care, etc.
We suppose that the logistic equation describes the temporal evolution of each wave of the epidemic adequately in countries with high-quality statistics. After processing the data, we select results using simple criteria and standard Maple software (Maplesoft; Canada).
There is no doubt that, theoretically, the epidemic can develop for the second and third time, etc. in an unpredictable way, if we neglect the appropriate anti-epidemic measures. As calculations show, the second wave of the epidemic, as a rule, rises after passing the critical point, which is the inflexion point of the logistic curve [8–10]. The linear equation a1 + 2a2N = 1 defines the threshold N.
In the case of secondary epidemic waves, we use the same dynamic system for calculations. The described above procedure calculates the current parameters a1 using a new, appropriately decomposed input data set. We remove the already calculated data, provided by the logistic curve of the first wave, from the old input data set. The computation process continues until the input data will represent a superposition of various individual logistic curves.
There is no doubt that all input is perfect. In case of failure of the specified decomposition of the input data, we decide that the algorithm described above does not work, and omit such input data from the analysis. Note that the idea of such an algorithm is not new — it first appeared in the socio-economic sciences [11].
There are examples of its successful application in mathematical biophysics [12]. In the context of the current pandemic, the work [13] is of interest. The authors take into account the secondary wave to adjust the results of their previous work [14].
RESULTS
Consider an example of the epidemic history of the countries that did not escape the risk of the second wave. Let it be the most vividly manifested country in this respect, Iran, the history of the epidemic of which is shown in fig. 1.
The graph above shows that around the middle of April, the second wave of the epidemic rose in Iran. The epidemic spread is still very far from complete since the growth of the third wave has been observed from the beginning of June. Notice that the mentioned above algorithm decomposing the secondary waves could not recognize the third wave, and interpreted the data as statistical errors for two or three weeks ago.
Let us now try identifying the first wave of the epidemic in Iran by estimating the absolute and relative errors between the input and forecast data, which are described by the logistic curve. The mean squared error is minimal if the first wave reaches the threshold at 93 days from the start of the epidemic (fig. 2). Note that the search for the minimum uses a priori dynamic data analysis that should not have to cover the entire history of the epidemic.
fig. 2 on the left displays that the input data, starting from the very beginning of the epidemic and up to the point of the maximum absolute error, obey the exponential dependence shown by the solid line. This fact is not a revelation: the behaviour of real data over time shown in graphs is not a specific feature of the history of the epidemic in Iran only. The authors of some works on the problem of the pandemic have noticed such a pattern, explaining this regular exponential component with useful medical intervention in the early epidemic.
However, it is easier to assume that this unavoidable regular error is due only to the specifics of data monitoring. Moreover, the analytical approximation of the input data set continues further, starting from the point of maximum absolute error up to the inflexion point using the power function [15, 16].
We have studied this regular monitoring error by analyzing the epidemic history in some countries of Western Europe, which had reached the first epidemic peak [17]. Notice that to reach the epidemic peak is necessary to apply a posteriori data analysis that leads to the same results that we obtain in the case of the dynamic a priori analysis. We have analytically revealed the regular component of the monitoring error. This error explained the reason for the failure of a priori mathematical forecasting of probable epidemic events in various countries of the world, undertaken in numerous studies. The statistical data processing of almost all countries that have reached the epidemic peak showed that the regular monitoring error is subject to a simple pattern: the higher the epidemic peak, the greater the time delay of the monitoring data compared to the forecast.
Let us identify the primary epidemic wave in Iran, following a priori approach. Thus, we believe that the discrete logistic equation
Ni +1 = 1,118Ni – 1,344 × 10-6Ni2
adequately describes the first wave. Notice that the parameters of the first wave no longer change throughout the epidemic when the second wave appears. fig. 3 shows the solution to this equation.
Now we decompose the input data taking into account that the logistic curve shown in the above figure adequately approximates the early history of the epidemic. At the next stage of processing the input data, we identify the second epidemic wave. Calculations are performed recursively in the same way as used above. The discrete logistic equation also adequately describes the second wave, but with a different right-hand side:
Ni + 1 = 1,056Ni – 3,202 × 10-7Ni2 .
The parameters of this equation slowly change in time until the formation of a sufficient height of the third wave. fig. 4 displays this decomposition graphically.
This figure displays that the residual data, corresponding to the secondary and tertiary waves, can no longer represent a single logistic line. Thus the epidemic in Iran has become a continuous wave cascade, the most unfavourable for combating the epidemic. In any case, Iran cannot avoid the third epidemic wave.
We can repeat the above arguments and calculations using other data on the epidemic history in other countries, for example, Sweden. The first epidemic wave of in Sweden, compared with Iran, had a relatively small height with the threshold of about 15,000 registered cases reached in 115 days after the start of the epidemic (fig. 5). Also, this illustration explains the input-data decomposition for detecting the secondary waves.
fig. 6 shows that a situation similar to that in Iran takes place in Ukraine.
In comparison with the other countries, the secondary epidemic wave in the United States has got a gigantic scale, growing exponentially (fig. 7).
Today, on the World Wide Web and television, there are more and more messages about the expectation of a second wave of the epidemic in Russia. These reports, as a rule, are based on speculation, which leaves open a burning question: should or not be the second wave of the epidemic in Russia?
Using our mathematical tools, we have found that the second epidemic wave in Russia had already risen in the first half of May. The height of this wave is about half the primary wave. The third wave has started in Russia at the end of June. Figure 8 represents a decomposition of the epidemic history in Russia as a superposition of the primary and secondary waves.
The appearance of the second wave in different countries possesses similar features despite some differences in the epidemic spread. We have admitted regularity in the rise of the second waves. These waves appear when passing through the critical point of the epidemic threshold of the current wave.
Pragmatically, passing through the threshold of a new wave should be the most responsible in terms of strict observance of the rules of self-isolation and other sanitary standards. The secondary waves of the epidemic are insidious in that they do not appear immediately but after a sufficiently long time. For example, in Iran, the second wave significantly showed itself in no less than 60 days.
DISCUSSION
There are several different mechanisms, each of which can generate secondary waves in acute infectious disease. The first two mechanisms are due to the direct viral transmission and a change in its behaviour, which favours the emergence of additional ways of its spread. The third one is associated with the heterogeneity of the population. A new wave either occupates new territories and population or rolls over the previous places again. The fourth item is a mutation in the virus that causes delayed susceptibility to infection in humans. One more mechanism is weakening immunity. There is no problem to take these mechanisms into account in complex mathematical models to simulate secondary waves [18]. We may add that secondary waves are, not least, a consequence of the lax compliance with quarantine and sanitary rules by people who trust rumours and consume unreliable mass information.
In this work, the authors abandoned multivariate models, which, in ideal implementation, are designed to study the impact, for example, of border control at the beginning of an outbreak, predicting the timing of self-isolation, assessing the required amount of available drugs, and others. Indeed, the practice of recent months has shown that the well-tested and widely known mathematical methods, directly used to describe future events of the spread of the epidemic by processing the available statistical data, have demonstrated ineffectiveness.
We know the well-known logistic equation adequately describes the epidemic evolution. However, neither various versions of this mathematical model nor modifications of the logistic equation demonstrate usefulness. In any case, the simulation gives a satisfactory result only on a small-time horizon, within one or two weeks [19].
If so, then we are dealing with not predictive, but with monitoring models, which, for all their value, are still useless for developing medium-term and strategic action plans to overcome the pandemic [20]. Other promising predictive technologies based on graph theory [21], percolation theory [22], and stochastic processes [23] turned out to be too clumsy or incapable to produce a clear and concrete result on an urgent issue. In other words, the available forecast methods have demonstrated helpless when solving the problem.
There is a paradox. On the one hand, it could seem that everything about the dynamics of the pandemic is known qualitatively in advance. On the other hand, standard methods cannot provide an adequate prediction.
Let us try to shed light on the main reasons for such a failure and try to formulate the principles for overcoming the current situation. First of all, note that, in contrast to the experimental data, say, in physical experiments, data on the epidemic situation cannot be redundant, but only insufficient. Indeed, it is difficult to imagine a situation where redundant data regularly appears in the monitoring fixing the total number of newly detected cases since the case is too delicate to allow such sloppiness. It is natural to expect that the data has a lack of information. Thus, we can postulate that the regular error describes some deficit in the data. Indeed, we can obtain a comprehensive epidemic data only a posterior. However, a priori analysis requires to identify this regular error using the statistical data of those countries which already have passed the epidemic peak.
We had managed to find the specified regular component of the error inherent in many countries of the world in monitoring the spread of the current pandemic in terms of exponential and power-law approximating functions. This error influences the forecasting of epidemic events critically. However, statistical data of countries have reached an epidemic peak demonstrate the analytical regularity in the virtual time delay. This fact allows us to pose the following question. Is a country that already has passed the threshold close to the peak epidemic or is still far from it?
The regular error of monitoring causes failures of a priori mathematical modelling [17]. We may point out that the predicted and real data, as a rule, practically coincide from the threshold to the peak of the epidemic. The explanation is simple: when crossing the inflexion point, the growth rate of registered infections decreases while the maximum effort is involved in solving the monitoring problem.
Thus, we have decided to limit ourselves to the simplest logistic model in this paper. On the one hand, this model requires a minimum of information to construct it. We need to know the initial number of cases, the final peak number, and the time from the beginning of the epidemic to its peak. On the other hand, we must understand that the monitoring quality, first of all, determines the reliability of the model, not the number of unknown parameters that we should find out from the input data. In particular, the conservatism of the parameters of the logistic curves for the primary waves confirms the model reliability while the parameters of the current wave slowly change in time because of the indicated natural imperfection of the input data.
CONCLUSION
This text has focused on the appearance of the second epidemic wave in Iran, Sweden and the United States, Ukraine, and Russia. The analysis of the input data by the total cases shows that the most favourable time for the emergence of secondary waves is the critical point in which the rate of the epidemic increase approaches maximum. The pass of the threshold of the new epidemic wave should be the most responsible in terms of strict observance of the rules of self-isolation and other sanitary standards.
The mathematical model allows detecting the secondary waves much earlier than passively observing the epidemic history. An example is the not entirely obvious second wave in Russia, which arose in early May, and which gave rise to the third wave of the epidemic at the end of June. Nonetheless, today, we desire that Russia is still able to avoid the fourth epidemic wave if combating the epidemic. Iran also has such an opportunity.
The algorithm for studying the secondary waves represents a recursive decomposition of the input data to a set of different logistic curves, the parameters of which, unknown in advance, the calculation process determines. At the first step, we check the possibility of adequate approximation of the input data by a single logistic curve. If success, we attempt to identify the second logistic wave, etc.
Logistics decomposition in many features is similar to the well-known Fourier method or wavelet analysis. However, in our case, the decomposition algorithm does not end with a result if the input data is inappropriate.