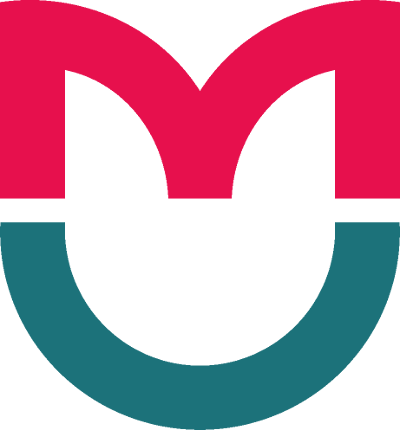
ORIGINAL RESEARCH
Predictive monitoring of secondary epidemic waves of COVID-19 in Iran, Russia and other countries
1 Nizhny Novgorod State Technical University n.a. R.E. Alekseev, Nizhny Novgorod, Russia
2 National Research Lobachevsky State University of Nizhny Novgorod, Nizhny Novgorod, Russia
Correspondence should be addressed: Svetlana P. Nikitenkova
Gagarina, 23, Nizhny Novgorod; 603950; moc.liamg@avoknetikins
Author contribution: Kovriguine DA — research planning, literature analysis, data analysis and interpretation, manuscript preparation; Nikitenkova SP — research planning, literature analysis, data analysis and interpretation, manuscript preparation.
- Moftakhar L, Mozhgan SEIF, Safe MS. Exponentially Increasing Trend of Infected Patients with COVID-19 in Iran: A Comparison of Neural Network and ARIMA Forecasting Models. Iranian Journal of Public Health. 2020; 49: 92–100.
- Tran TT, Pham LT, Ngo QX. Forecasting epidemic spread of SARS-CoV-2 using ARIMA model (Case study: Iran). Global Journal of Environmental Science and Management. 2020; 6 (Special Issue (Covid-19)): 1–10.
- Zareie B, Roshani A, Mansournia MA, Rasouli MA, Moradi G. A model for COVID-19 prediction in Iran based on China parameters. 2020; medRxiv.
- Ahmadi A, Fadai Y, Shirani M, Rahmani F. Modeling and forecasting trend of COVID-19 epidemic in Iran until May 13, 2020. Medical Journal of The Islamic Republic of Iran (MJIRI). 2020; 34 (1): 183–95.
- Li L, Yang Z, Dang Z, Meng C, Huang J, Meng H, Wang D, Chen G, Zhang J, Peng H, Shao Y. Propagation analysis and prediction of the COVID-19. Infectious Disease Modelling. 2020; 5: 282–92.
- Kovriguine D, Nikitenkova S. On the secondary waves of the pandemic launched in Iran and other countries. medRxiv. 2020; DOI: https://doi.org/10.1101/2020.06.20.20136283.
- Nejmark YuI. Matematicheskie modeli v estestvoznanii i tehnike. N. Novgorod: Izd-vo Nizhegorodskogo universiteta im. N. I. Lobachevskogo. 2004; 401 s. Russian.
- Source сode Maple-18. Available from: https://kovriguineda. ucoz.ru/COVID-19/COVID-19-Iran.mw.
- Source сode Maple-18. Available from: https://kovriguineda. ucoz.ru/COVID-19/COVID-19-Russia.mw.
- Source сode Maple-18. Available from: https://kovriguineda. ucoz.ru/COVID-19/COVID-19-Ukraine.mw.
- Meyer P. Bi-logistic growth. Technological forecasting and social change. 1994; 47 (1): 89–102.
- Lavrova AI, Postnikov EB, Manicheva OA, Vishnevsky BI. Bi-logistic model for disease dynamics caused by Mycobacterium tuberculosis in Russia. Royal Society open science. 2017; 4 (9): 171033.
- Dattoli G, et al. On the evolution of covid-19 in Italy: a follow up note. Available from: arXiv preprint arXiv:2003. 2020; 12667.
- Dattoli G, Di Palma E, Licciardi S, Sabia E. A Note on the Evolution of Covid-19 in Italy, arXiv:2003.08684v1 [q-bio.PE], 19 Mar 2020.
- Bhattacharya S, Islam MM, De A. Search for the trend of COVID-19 infection following Farr's law, IDEA model and power law. medRxiv. 2020; Available from: https://doi.org/10.1101/202 0.05.04.20090233.
- Singer HM. The COVID-19 pandemic: growth patterns, power law scaling, and saturation. medRxiv. 2020; Available from: https:// doi.org/10.1101/2020.07.12.20152140.
- Nikitenkova SP, Kovriguine DA. It's the very time to learn a pandemic lesson: why have predictive techniques been ineffective when describing long-term events? medRxiv. 2020; Available from: https://doi.org/10.1101/2020.06.01.20118869.
- Mummert A, et al. A perspective on multiple waves of influenza pandemics. PloS one. 2013; 8 (4): e60343.
- Lakman IA, Agapitov AA, Sadikova LF, Chernenko OV, Novikov SV, Popov DV, i dr. Vozmozhnosti matematicheskogo prognozirovanija koronavirusnoj infekcii v Rossijskoj Federacii. Arterial'naja gipertenzija. 2020; 26 (3): 288–94. Dostupno po ssylke: https:// doi.org/10.18705/1607-419X-2020-26-3-288-294. Russian.
- Holmdahl I, Buckee C. Wrong but useful — what covid-19 epidemiologic models can and cannot tell us. N Engl J Med. 2020 May 15. DOI: 10.1056/NEJMp2016822.
- Ivannikov YuG, Ogarkov PI. Opyt matematicheskogo komp'juternogo prognozirovanija jepidemij grippa dlja bol'shih territorij. Zhurnal infektologii. 2012; 4 (3): 101–06. Available from: https://doi. org/10.22625/2072-6732-2012-4-3-101-106. Russian.
- Luo Y, Schaposnik LP. Minimal percolating sets for mutating infectious diseases. Physical Review Research. 2020; 2 (2): 023001.
- de Arruda GF, Petri G, Moreno Y. Social contagion models on hypergraphs. Physical Review Research. 2020; 2 (2): 023032.