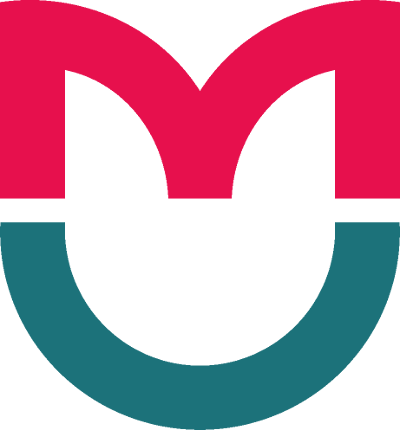
ORIGINAL RESEARCH
Altered neurometabolic potential of gut microbiome in healthy children of different age
1 Vavilov Institute of General Genetics, Moscow, Russia
2 Psychiatric Hospital № 1 Named after N. A. Alexeev, Moscow, Russia
Correspondence should be addressed: Alexey S. Kovtun
Gubkina, 3, Moscow, 119991; moc.liamg@52sanutvok
Funding: the study was supported by the Russian Science Foundation, project № 20-14-00132.
Compliance with ethical standards: the study was approved by the Ethics Committee of the Pirogov Russian National Research Medical University (protocol № 165 dated May 22, 2017). The informed consent was obtained from parents of all children.
Author contribution: Kovtun AS — algorithm development, bioinformatics analysis, catalogue creation, data interpretation and vizualization; Averina OV — method development, catalogue creation, data interpretation, manuscript writing; Poluektova EU — method development, catalogue creation; Kostyuk GP and Danilenko VN — study concept, method development, data interpretation.
Today, human gut microbiota is considered an important organ, which plays a vital part in preserving human health. Gut microbiota is the microbial population colonizing the gastrointestinal tract. The healthy gut microbial communities, which contribute to preserving the metabolic homeostasis, live inside the host maintaining the immunological tolerance. Due to symbiotic relationship, human gut microbiota carries out various tasks contributing to the host’s physiology [1]. The host co-evolves with the microbiota; the composition of gastrointestinal tract microbial community changes in response to various internal and external stimuli. Bacterial species colonizing the gastrointestinal tract in infancy affect the host’s health later in life [2]. The gut microbiota’s bacterial composition becomes stable after the first three years of life getting closer to the adult gut microbiota profile [3].
Clinical and experimental data demonstrate the significant impact of human gut microbiota on the broad range of behaviors, including the social behavior, mood, emotions, anxiety and nuitrition [4]. Gut bacteria affect various human neurological conditions via the microbiota-gut-brain axis [4]. The gut microbiota composition may affect the neural network formation during the early nervous system development [5]. Bacteria influence the central nervous system (CNS) and enteric nervous system (ENS) in a variety of ways via metabolites and hormones of immune system and afferent nerves. Bacteria produce hundreds of compounds, which may affect the host’s physiology. The gut microbiota composition alterations may result in major changes in metabolite production. Since the host is constantly exposed to such molecules, those may facilitate the development of various neuropsychiatric disorders, including depression [6].
Adolescence and puberty are critical periods for the developing nervous system with numerous structural, neurochemical and molecular changes occurring in response to genetic and environmental signals. At this age microbiota is also subject to significant shifts in composition and functioning. Steroid hormones cause the sex-specific differences in the gut microbial composition. Maturation of human gut microbiota runs alongside with the dynamic brain development; both processes have similar critical periods of development [7].
The use of next-generation sequencing (NGS) provides a better understanding of gut microbiota composition and allows one to explore its structural changes throughout the lifespan [8]. During our work, we used the shotgun metagenomic sequencing to study the human gut microbiota. The method is based on sequencing of the complete genomic material of the microbiota sample, which makes it possible both to obtain full data on bacterial composition, and to assess the overall microbiota metabolic functions and the functional capabilities of all bacteria. Moreover, the method may be used for strain-level microbiota analysis. The study was aimed to detect alterations in the taxonomic composition and content of bacterial genes encoding key enzymes involved in the metabolism of neuroactive compounds and biomarker metabolites in the metagenomes of healthy children of different age groups: 3–5 years old (children’s metagenomes (ChM) and 15 years old (adolescent metagenomes (AM).
METHODS
Cohorts and metagenome sequencing
The study included the previously sequenced gut microbiota metagenomes isolated from 23 healthy neurotypical children aged 3–5 (ChM group) [9] and 7 adolescents aged 15 (AM group) [10] living in Moscow Region. Inclusion criteria: age; no gastrointestinal disorder prior to sampling; geographic region of origin — Moscow and Moscow Region; no exposure to antibiotics, probiotics and prebiotics within 2 months before sampling; no mental disorder (depression, schizophrenia, bipolar disorder, etc.); no diarrhea. Faecal specimens obtained from each volunteer were stored in sterile plastic containers before analysis at a temperature of –80 °C.
Metagenomic DNA isolation, library construction and sequencing using the Illumina HiSeq system (Illumina; USA) were carried out in accordance with the previously reported algorithm [9]. Metagenomic reads were deposited in the Sequence Read Archive (SRA) NCBI (the ChM BioProject ID was PRJNA516054, and the AM ID was PRJNA380118). The raw sequence data quality control was performed using the FastQC tool, and the Trimmomatic tool was used for trimming [11, 12]. The bases with quality score Q < 20 and sequences shorter than 50 bp were removed. In order to remove human DNA, all reads were mapped to the human genome (hg19 assembly) using the bowtie2 tool [13]. The metagenomic reads were assembled into contigs using the metaSPADes software [14].
Parameters of the sequenced samples and the resulting assemblies are presented in tab. 1.
Catalogue creation
The catalogue of gene homologs involved in synthesis and metabolism of various neuroactive compounds, which was introduced before [9, 15], was updated and expanded. The genes involved in synthesis and metabolism of various compounds and metabolites reported as biomarkers of depression were added to the catalogue [16]. The amino acid sequences of these genes’ homologs were selected in accordance with the previously reported algorithm [9] (tab. 2).
Taxonomic and statistical analysis
The taxonomic composition was defined using the Kraken2 [17] and TAGMA [18] software. The analyses were carried out separately for the taxonomic levels of phylum, genus and species. The alpha diversity (Shannon’s diversity index) was assessed using the R programming language.
The significant differences in the taxonomic composition at the genus and species level were defined using the Wilcoxon signed-rank test and the multiple testing correction based on permutation test (1000 permutations), the significance threshold was set to P-value < 0.1. This value was chosen due to the relatively small number of samples in the AM group.
Signature identification in metagenomic data
Metagenomic signature is a combination of genes found in the metagenome and bacteria containing such genes [9]. In order to define the signatures, the metagenomic assemblies were analyzed using the previously reported algorithm [15]. The search for open reading frames (ORF) was performed using the MetaGeneMark software (USA) [19]. The ORFs were annotated using the catalogue created and BLASTp with the following parameters: homology ≥ 60%; relative alignment score ≥ 80%. Bacterial origin of ORFs was defined at the taxonomic level of species using the Kraken2 software. All unclassified sequences were designated as “Unclassified”. Thus, a set of pairs was obtained for each species (species; gene). In order to assess the relative abundance of the pair, the reads were mapped to the appropriate ORFs using the Burrows-Wheeler Alignment (BWA) tool [20]. The counts were normalized by the Trimmed Mean of M-values (TMM) method using the edgeR library [21]. The comparison of relative abundance values for the ChM and AM groups was carried out using the Wilcoxon signed-rank test and the multiple testing correction based on permutation test (1000 permutations; P-value < 0.1).
RESULTS
Expanded gene catalogue
The previously constructed reference catalogue of gene homologs involved in the various neuromodulators metabolism has been expanded by adding genes, which encode enzymes involved in the new compounds production and the various neuroactive metabolites destruction [9, 15]. The resulting catalogue comprises 742 amino acid sequences for gene homologs encoding the 68 bacterial enzymes. The new enzymes involved in γ-aminobutyric acid (GABA), nitric oxide, γ-hydroxybutyric acid and p-cresol decomposition, isovaleric acid, inositol and glutamate synthesis and decomposition, as well as antioxidant enzymes (superoxide dismutase, catalase and glutathione peroxidase) have been added to the catalogue. The full list of genes included in the updated catalogue is presented in tab. 2.
Core neurometabolic signature of the healthy children gut microbiota
In the first phase, the search for bacterial genes encoding the key enzymes involved in the synthesis of neuroactive compounds and biomarkers of depression, which may affect the development and functioning of the child’s nervous system during his/her early life was carried out in the metagenomes of the ChM group (fig. 1). Only those genes found in more than 50% of samples were taken into account. The most abundant genes were those encoding methylmalonyl-CoA decarboxylase (propionic acid production), phosphotransacetylase (acetic acid production), glutamate decarboxylase (GABA synthesis), gamma-aminobutyrate antiporter (GABA transport) and histidine ammonia-lyase (histidine destruction). Gene homologs involved in metabolic pathways of GABA, serotonin, melatonin, butyric acid, conjugated linoleic acid, spermidine, isovaleric acid, inositol, γ-hydroxybutyric acid, glutamate, creatinine, indole, tryptophan, superoxide dismutase, catalase and glutathione peroxidase were also detected.
Then, the bacterial origin of genes at the species level was defined, and the signature pairs were constructed (fig. 2A). The pairs defined in the vast majority of samples (over 70%) comprised the core neurometabolic signature of the ChM (fig. 2B). The ChM core neurometabolic signature included four species (Bacteroides uniformis, Faecalibacterium prausnitzii, Lachnospiraceae bacterium и Parabacteroides distasonis) and genes encoding 15 enzymes (glutamate decarboxylase, gamma-aminobutyrate antiporter, serotonin-N-acetyltransferase, phosphotransacetylase, butyrate kinase, butyryl-CoA dehydrogenase, methylmalonyl-CoA decarboxylase, linoleic acid isomerase, spermidine synthase, two subunits of glutamate synthase, histidine ammonia-lyase, tryptophanase, beta-subunit of tryptophan synthase and superoxide dismutase).
Age-related alterations in the neurometabolic signature of the human gut microbiota
Metagenomic samples for AM were analyzed using the same algorithm as the ChA samples. First, the search for gene homologs from the catalogue was carried out (see fig. 1). On average, the increased relative abundance was noted for all genes found in more than 50% of the AM group samples compared to the ChM group. No homologs of genes encoding 4-aminobutyrate aminotransferase, creatinine amidohydrolase and superoxide dismutase (gene sodC) were found in the AM, however, this could be due to small sample size. Thus, the statistical power of the tests was low. The significant (adjusted P-value < 0.1) abundance increase was detected in AM for genes encoding phosphotransacetylase, butyryl-CoA dehydrogenase, methylmalonyl-CoA decarboxylase, 4-hydroxyphenylacetate decarboxylase, alpha- and beta-subunits of tryptophan synthase, superoxide dismutase (gene sodB) and catalase.
Then the metagenomic signatures for AM were constructed, and the abundance of pairs (species; gene) was compared with ChM (fig. 2B). The significant abundance increase was detected for the following pairs: (Alistipes onderdonkii; catalase), (A. onderdonkii; glutamate decarboxylase), (A. onderdonkii; histidine ammonia-lyase), (A. onderdonkii; 4-hydroxyphenylacetate decarboxylase), (Bacteroides vulgatus; gamma-aminobutyrate antiporter), (Bacteroides thetaiotaomicron; methylmalonyl-CoA decarboxylase) and (Barnesiella viscericola; methylmalonyl-CoA decarboxylase). However, the observed alterations in the abundance of pairs comprising the core signature were not significant.
Comparative taxonomic analysis of gut microbiota in children of different age groups
All metagenomes were analyzed using the Kraken2 software. The alpha diversity comparison for ChM and AM is presented in fig. 3. The average value of the Shannon’s diversity index for AM was higher both at the genus (fig. 3A) and species level (fig. 3B).
The taxonomic composition of the ChM and AM was defined for the taxonomic levels of phylum, genus and species. At the phylum level, the AM were characterized by significant increase in abundance of Proteobacteria (8.99% vs. 3.37% in ChM and AM respectively, P-value = 0.001) (fig. 3C). The differences were also revealed for phyla Actinobacteria (4.85% vs. 2.77%; P-value = 0.735), Bacteroidetes (60.55% vs. 66.94%; P-value = 0.421), Firmicutes (21.08% vs. 24.42%; P-value = 0.758) and Verrucomicrobia (0.40% vs. 1.36%; P-value = 0.298), however, the differences were not significant.
The comparison of abundance values at the genus (tab. 3) and species (tab. 4) level included only taxa identified in more than 50% of samples. As a result, the significant abundance increase (P-value < 0.1) was detected for genera Butyrivibrio, Gordonibacter and Prevotella. At the species level, there was a significant (P-value < 0.1) increase in abundance of Alistipes communis, Alistipes megaguti, Alistipes sp. dk3624, Butyrivibrio fibrisolvens, Butyrivibrio proteoclasticus, Eggerthella sp. YY7918, Lactobacillus reuteri, Lactobacillus ruminis, Prevotella dentalis, Prevotella denticola, Prevotella enoeca, Prevotella jejuni, Prevotella oris and Prevotella ruminicola, and the decrease in abundance of Bacteroides sp. A1C1, Gordonibacter pamelaeae, Enterococcus faecalis and Streptococcus thermophiles.
In addition, the analysis of strain diversity for the studied samples was carried out using the TAGMA software (Russia) [18] (tab. 5). In AM, the increased median number of strains compared to ChM was observed for the Clostridium botulinum, Clostridium perfringens, Escherichia coli and Streptococcus pneumonia species. The larger number of strains per sample was also detected for the Enterococcus faecium species. However, the maximum number of strains was higher in the ChM group. Lower strain diversity in the AM group was observed for the Bacteroides fragilis species. Furthermore, in Klebsiella pneumonia, the median numbers of strains per sample were identical in both groups. However, the maximum number of strains was significantly higher in AM.
DISCUSSION
In order to study the possible mechanisms of the human gut microbiota impact on the early childhood neurostructural and neurocognitive development in healthy children, we focused on the group of bacterial genes encoding the enzymes involved in metabolism of neuroactive compounds, which correlate with dysregulations resulting in neurometabolic disorders and depression. The use of the constructed catalogue of homologs to genes of the selected group made it possible to define the neurometabolic signature of the ChM. The signature approach was used to reveal the bacterial species comprising the largest number of genes (more than seven genes) responsible for production of various neuroactive compounds and thus having greater potential to affect the child’s brain development and functioning. The revealed species were B. uniformis, F. prausnitzii, L. bacterium and P. distasonis being the gut commensals in healthy young children [22]. These bacteria contain genes encoding the proteins involved in production of acetic, propionic and butyric acids, GABA, as well as antioxidant enzymes having a positive impact on the people’s mental health. B. uniformis, F. prausnitzii and L. bacterium comprise the core neurometabolic signature of the healthy children gut microbiota, which may be used as an early childhood biomarker of normal microbiota.
Here we report the pilot study. This is the early stage of exploring the alterations in metabolic potential of gut microbiota in healthy children from early childhood to adolescence. So far, the small number of adolescents’ samples was used for comparison. We had to determine whether the alterations in microbiota occurred during the child’s development from infancy to adulthood. For this purpose, we compared taxonomic profiles and the content of bacterial genes encoding the key enzymes involved in the metabolism of neuroactive compounds.
We revealed differences in the quantitative content of bacterial genes responsible for production and destruction of neuroactive compounds in the compared metagenomes of children of different age groups, which was considered the most important result of the study. In AM, the two-fold increase in abundance of genes encoding the enzymes involved in the propionic, acetic and butyric acids, glutamate, and tryptophan production, histidine degradation, as well as in production of conjugated linoleic acid and antioxidant proteins was detected. As is known, all the listed above compounds have a positive impact on the gut and brain functioning, and contribute to homeostasis maintenance. The impact of the short-chain fatty acid levels on the energy homeostasis of the host has been reported [23]. Tryptophan is a substrate for the synthesis of neurotransmitter, serotonin [24]. Neurotransmitters serotonin and glutamate play a critical part in depression [25]. Conjugated linoleic acid and antioxidant proteins are the important factors of defense against oxidative stress. The content of other detected genes was negligible and demonstrated small differences. These data are likely to represent the varying contribution of the studied bacterial genes to maintaining the normal nervous system development in healthy children. The further transcriptome and metabolome analyses will be carried out to validate the results of the metagenomes bioinformatics analysis.
Earlier studies (based on the analysis of the 16S rRNA genes) aimed at the comparison of gut microbiota in children of different age groups revealed significant differences in the taxonomic composition [26]. In our study we used the data obtained by the shotgun metagenomic sequencing for the comparative metagenomic analysis. Although a small sample of AM was used for comparison, the results obtained also demonstrated typical differences in the taxonomic composition of gut microbiota in children of different age groups. The significant increase in bacteria of the phylum Proteobacteria and no significant differences for phyla Actinobacteria, Bacteroidetes and Firmicutes were revealed in AM. The alpha diversity of AM was higher both at the genus and species level, which was consistent with the published data on the more diverse microbiota of adolescents compared to young children [26]. High biodiversity often correlates with the higher content of probiotic bacteria. Our study revealed higher content of bifidobacteria (В. adolescentis) and lactobacilli in AM. It is known that bifidobacteria and lactobacilli exhibit probiotic properties. Recently those were proposed as psychobiotics due to their ability to produce neuromodulators and affect the brain–gut interactions [27]. The significant relative content increase for the Prevotella genus representatives was detected in AM, as well as the decreased content of A. muciniphila, which demonstrated the negative correlation with obesity and inflammation [28]. Perhaps, the microbiota composition alterations observed in children as they mature are due to the impact of the diet and hormones. In turn, alterations in the composition if microbiota may affect the development of different brain areas [29].
Our findings revealed the strain diversity in both groups of metagenomes. The median increase of the bacterial strains in the AM for pathogenic bacteria C. botulinum, C. perfringens, E. coli and S. pneumonia was detected. Perhaps, that could be due to increased exposure to antibiotics during maturation. It is interesting that in the AM group there were more strains per sample (on average) for the E. faecium species, and less strains per sample for B. fragilis. Alterations in the strain-level microbiota composition may change its metabolic activity due to strain-specific production of various active compounds by bacteria. Combining the shotgun sequencing with the metagenome signature approach and the bioinformatics tools allowing one to perform the strain-level taxonomic analysis could put us closer to constructing the strain-level metagenomic signatures. This, in turn, would help to reveal the potential for specific production of neuroactive compounds in the new strains. This information could then be used to develop the methods for diagnosis of such neuropsychiatric disorders as depression, as well as to develop the targeted therapy for these disorders based on the use of pharmaceutical compounds, probiotics, prebiotics and/ or psychobiotics [30].
CONCLUSION
The study results confirm and expand the knowledge that the gut microbial communities become more diverse and functional as their human hosts become older. The gut microbial communities significantly enrich themselves with genes involved in metabolism of neuroactive compounds and compounds possessing anti-inflammatory and antioxidant activity necessary for the nervous system function. These alterations occur in response to external and internal factors, such as diet, antibiotics, hormones, stress, etc. The detected neurometabolic signature of the healthy children gut microbiota may be used as a marker of the normal human gut microbiota condition. Future research should be focused on identification of the gut microbiota metagenomic signature in healthy children of different age groups from different backgrounds.