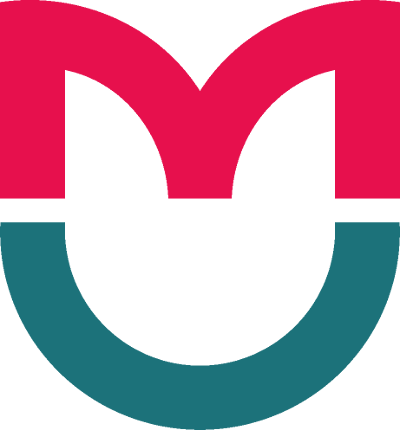
This article is an open access article distributed under the terms and conditions of the Creative Commons Attribution license (CC BY).
ORIGINAL RESEARCH
Labelling of data on fundus color pictures used to train a deep learning model enhances its macular pathology recognition capabilities
1 Pirogov Russian National Research Medical University, Moscow, Russia
2 OOO Innovatsioonniye Tekhnologii (Innovative Technologies, LLC), Nizhny Novgorod, Russia
3 Volga District Medical Center under the Federal Medical-Biological Agency, Nizhny Novgorod, Russia
4 Ivannikov Institute for System Programming of RAS, Moscow, Russia
5 L.A. Melentiev Energy Systems Institute, Irkutsk, Russia
Correspondence should be addressed: Pavel V. Gliznitsa
Belinskogo, 58/60, et. 5, 603000, Nizhny Novgorod; moc.duolci@pastinzilg
Funding: this work was financially supported by the Foundation for Assistance to Small Innovative Enterprises in Science and Technology (contract №150ГС1ЦТНТИС5/64226 dated December 22, 2020)
Author contribution: Takhchidi HP — manuscript editing; Gliznitsa PV — study concept and design, data collection and processing, results analysis, manuscript writing; Svetozarskiy SN — participation in data collection, literature and results analysis, manuscript writing; Bursov AI — literature analysis, algorithms development, manuscript editing; Shusterzon KA — algorithms development and validation, illustrations preparation, text writing.
Retinal diseases remain one of the leading causes of visual impairments in the world. The development of automated diagnostic methods can improve the efficiency and availability of the macular pathology mass screening programs. The objective of this work was to develop and validate deep learning algorithms detecting macular pathology (age-related macular degeneration, AMD) based on the analysis of color fundus photographs with and without data labeling. We used 1200 color fundus photographs from local databases, including 575 retinal images of AMD patients and 625 pictures of the retina of healthy people. The deep learning algorithm was deployed in the Faster RCNN neural network with ResNet50 for convolution. The process employed the transfer learning method. As a result, in the absence of labeling, the accuracy of the model was unsatisfactory (79%) because the neural network selected the areas of attention incorrectly. Data labeling improved the efficacy of the developed method: with the test dataset, the model determined the areas with informative features adequately, and the classification accuracy reached 96.6%. Thus, image data labeling significantly improves the accuracy of retinal color images recognition by a neural network and enables development and training of effective models with limited datasets.
Keywords: screening, machine learning, retinal diseases, fundus camera, biomedical visualization, data labeling