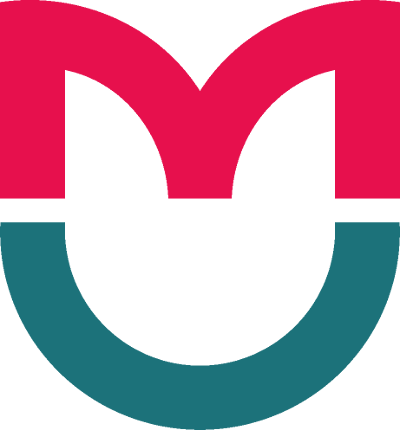
This article is an open access article distributed under the terms and conditions of the Creative Commons Attribution license (CC BY).
ORIGINAL RESEARCH
Labelling of data on fundus color pictures used to train a deep learning model enhances its macular pathology recognition capabilities
1 Pirogov Russian National Research Medical University, Moscow, Russia
2 OOO Innovatsioonniye Tekhnologii (Innovative Technologies, LLC), Nizhny Novgorod, Russia
3 Volga District Medical Center under the Federal Medical-Biological Agency, Nizhny Novgorod, Russia
4 Ivannikov Institute for System Programming of RAS, Moscow, Russia
5 L.A. Melentiev Energy Systems Institute, Irkutsk, Russia
Correspondence should be addressed: Pavel V. Gliznitsa
Belinskogo, 58/60, et. 5, 603000, Nizhny Novgorod; moc.duolci@pastinzilg
Funding: this work was financially supported by the Foundation for Assistance to Small Innovative Enterprises in Science and Technology (contract №150ГС1ЦТНТИС5/64226 dated December 22, 2020)
Author contribution: Takhchidi HP — manuscript editing; Gliznitsa PV — study concept and design, data collection and processing, results analysis, manuscript writing; Svetozarskiy SN — participation in data collection, literature and results analysis, manuscript writing; Bursov AI — literature analysis, algorithms development, manuscript editing; Shusterzon KA — algorithms development and validation, illustrations preparation, text writing.
- Nazarjan MG, Vertash OYu. Analiz pokazatelej pervichnoj i povtornoj invalidnosti vsledstvie boleznej glaza u lic pensionnogo vozrasta v Rossijskoj Federacii i Moskve. Uspehi gerontologii. 2019; 32 (1–2): 215–17. Russian.
- Armstrong GW, Lorch AC. A(eye): A Review of Current Applications of Artificial Intelligence and Machine Learning in Ophthalmology. Int Ophthalmol Clin. 2020; 60 (1): 57–71. DOI: 10.1097/IIO.0000000000000298. PMID: 31855896.
- Bird AC, Bressler NM, Bressler SB, Chisholm IH, Coscas G, Davis MD, et al. An international classification and grading system for agerelated maculopathy and age-related macular degeneration. The International ARM Epidemiological Study Group. Surv Ophthalmol. 1995; 39 (5): 367–74.
- Wong WL, Su X, Li X, et al. Global prevalence of age-related macular degeneration and disease burden projection for 2020 and 2040: a systematic review and meta-analysis. Lancet Glob Health. 2014; 2 (2): e106–e116. DOI: 10.1016/S2214-109X(13)70145-1.
- Schwartz R, Warwick A, Olvera-Barrios A, Pikoula M, Lee AY, Denaxas S, et al. Evolving treatment patterns and outcomes of neovascular age-related macular degeneration over a decade. Ophthalmol Retina. 2021; 5 (8): e11–e22. DOI: 10.1016/j.oret.2021.04.001. Epub ahead of print. PMID: 33866023.
- Danis RP, Domalpally A, Chew EY, et al. Methods and reproducibility of grading optimized digital color fundus photographs in the AgeRelated Eye Disease Study 2 (AREDS2 Report Number 2). Invest Ophthalmol Vis Sci. 2013; 54 (7): 4548–54.
- Klais C, et al. Photoscreening for diabetic retinopathy: a comparison of image quality between film photography and digital imaging. Clin Experiment Ophthalmol. 2004; 32: 393–6
- Ferris 3rd FL, Wilkinson CP, Bird A, et al. Clinical classification of age-related macular degeneration. Ophthalmology. 2013; 120 (4): 844–51.
- He K, Zhang X, Ren S, Sun J. Deep Residual Learning for Image Recognition. 2016 IEEE Conference on Computer Vision and Pattern Recognition (CVPR). 2016, pp. 770–778. DOI: 10.1109/ CVPR.2016.90.
- Deng J, Dong W, Socher R, Li L, Li K, Fei-Fei L. ImageNet: A large-scale hierarchical image database. 2009 IEEE Conference on Computer Vision and Pattern Recognition. 2009; pp. 248–255. DOI: 10.1109/CVPR.2009.5206848.
- Ren S, He K, Girshick R, Sun J. Faster R-CNN: Towards RealTime Object Detection with Region Proposal Networks. IEEE Transactions on Pattern Analysis and Machine Intelligence. 2017; 39 (6): 1137–49. DOI: 10.1109/TPAMI.2016.2577031.
- Lee CS, Baughman DM, Lee AY. Deep learning is effective for the classification of OCT images of normal versus Age-related Macular Degeneration. Ophthalmol Retina. 2017; 1 (4): 322–7. DOI: 10.1016/j.oret.2016.12.009.
- Burlina P, Joshi N, Pacheco KD, Freund DE, Kong J, Bressler NM. Utility of Deep Learning Methods for Referability Classification of Age-Related Macular Degeneration. JAMA Ophthalmol. 2018; 136 (11): 1305–7. DOI: 10.1001/jamaophthalmol.2018.3799.
- Burlina PM, Joshi N, Pekala M, Pacheco KD, Freund DE, Bressler NM. Automated Grading of Age-Related Macular Degeneration From Color Fundus Images Using Deep Convolutional Neural Networks. JAMA Ophthalmol. 2017 Nov 1; 135 (11): 1170–6. DOI: 10.1001/jamaophthalmol.2017.3782. PMID: 28973096; PMCID: PMC5710387.
- Keel S, Li Z, Scheetz J, Robman L, Phung J, Makeyeva G, et al. Development and validation of a deep-learning algorithm for the detection of neovascular age-related macular degeneration from colour fundus photographs. Clin Exp Ophthalmol. 2019 Nov; 47 (8): 1009–18. DOI: 10.1111/ceo.13575. Epub 2019 Jul 25. PMID: 31215760.
- Cheung R, Chun J, Sheidow T, Motolko M, Malvankar-Mehta MS. Diagnostic accuracy of current machine learning classifiers for age-related macular degeneration: a systematic review and metaanalysis. Eye (Lond). 2021 May 6. DOI: 10.1038/s41433-02101540-y. Epub ahead of print. PMID: 33958739.
- Dong L, Yang Q, Zhang RH, Wei WB. Artificial intelligence for the detection of age-related macular degeneration in color fundus photographs: A systematic review and meta-analysis. E Clinical Medicine. 2021 May 8; 35: 100875. DOI: 10.1016/j.eclinm.2021.100875. PMID: 34027334; PMCID: PMC8129891.
- Grassmann F, Mengelkamp J, Brandl C, Harsch S, Zimmermann ME, Linkohr B, et al. A Deep Learning Algorithm for Prediction of Age-Related Eye Disease Study Severity Scale for AgeRelated Macular Degeneration from Color Fundus Photography. Ophthalmology. 2018 Sep; 125 (9): 1410–20. DOI: 10.1016/j.ophtha.2018.02.037. Epub 2018 Apr 10. PMID: 29653860.
- Peng Y, Dharssi S, Chen Q, Keenan TD, Agrón E, Wong WT, et al. DeepSeeNet: A Deep Learning Model for Automated Classification of Patient-based Age-related Macular Degeneration Severity from Color Fundus Photographs. Ophthalmology. 2019 Apr; 126 (4): 565–75. DOI: 10.1016/j.ophtha.2018.11.015. Epub 2018 Nov 22. PMID: 30471319; PMCID: PMC6435402.
- Keenan TD, Dharssi S, Peng Y, Chen Q, Agrón E, Wong WT, et al. A Deep Learning Approach for Automated Detection of Geographic Atrophy from Color Fundus Photographs. Ophthalmology. 2019 Nov; 126 (11): 1533–40. DOI: 10.1016/j. ophtha.2019.06.005. Epub 2019 Jun 11. PMID: 31358385; PMCID: PMC6810830.
- Keenan TDL, Chen Q, Peng Y, Domalpally A, Agrón E, Hwang CK, et al. Deep Learning Automated Detection of Reticular Pseudodrusen from Fundus Autofluorescence Images or Color Fundus Photographs in AREDS2. Ophthalmology. 2020 Dec; 127 (12): 1674–87. DOI: 10.1016/j.ophtha.2020.05.036. Epub 2020 May 21. PMID: 32447042.