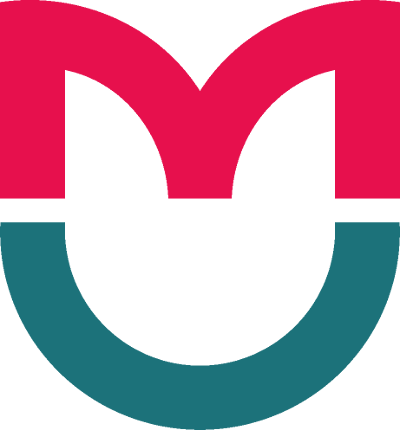
ORIGINAL RESEARCH
Cartographic atlas of frequency variation for 45 pharmacogenetic markers in populations of Russia and its neighbor states
1 Bochkov Research Center for Medical Genetics, Moscow, Russia
2 Biobank of North Eurasia, Moscow, Russia
3 Vavilov Institute of General Genetics, Moscow, Russia
4 Lomonosov Moscow State University, Moscow, Russia
5 Kuban State Medical University, Krasnodar, Russia
6 Russian Medical Academy of Continuous Professional Education, Moscow, Russia
Correspondence should be addressed: Elena V. Balanovska
Moskvorechie, 1, Moscow, 115478; ur.liam@aksvonalab
Funding: the study was carried out under the State Assignment of the Russian Ministry of Science and Higher Education for the Research Center for Medical Genetics and Vavilov Institute of General Genetics.
Acknowledgement: the authors thank the donors for their participation, the Institute of General Genetics for the access to the database of genotypes, the Center for Precision Genome Editing and Genetic Technologies for Biomedicine of Pirogov Russian National Research Medical University (Moscow, Russia) for the opportunity to usemolecular genetic technologies.
Author contribution: Balanovska EV — data analysis, manuscript draft; Balanovsky OP — study design and supervision; Petrushenko VS — bioinformatic analysis; Koshel SM — map analysis, manuscript editing; Chernevskiy DK, Pocheshkhova EA — tabular data analysis; Mirzaev KB, Abdullaev SP — description of pharmacogenetic markers.
Compliance with ethical standards: the study was approved by the Ethics Committee of the Research Center for Medical Genetics (Protocol № 3/1 dated September 5, 2018) and carried out on the samples obtained during the population genetic study of the gene pools of ethnic groups from Russia and its neighbor states. The donors gave voluntary informed consent to participate.
The key genes involved in the absorption, distribution, metabolism, and elimination (ADME) of drugs have been described in multiple studies. Although ADME genes are relatively modest in number, each of them is represented by a few functionally relevant polymorphic variants [1, 2]. In addition to ADME genes, research has identified drug target genes and the genes encoding the components of the hemostatic system. Assays and protocols are being developed to guide drug selection and dosing based on the patient’s genotype [3–5]. However, genetic differences between ethnicities remain the major hurdle impeding the widespread adoption of such assays: commercial panels designed to screen ADME genes for clinically significant polymorphisms do not account for their variation across races and ethnic groups and thus are poorly adapted for ethnically diverse regions.
Studies conducted in polyethnic regions across the world have demonstrated both quantitative (allele frequencies) and qualitative (population-specific alleles) differences in ADME genes and other pharmacogenetic markers between ethnic groups. Although interethnic differences have been well known since the inception of pharmacogenetics [6, 7], most pharmacogenetic studies are still carried out on Caucasian patients. Consequently, their results cannot be extrapolated to other ethnic groups. For example, it has been shown that standard algorithms for essential drug dosing are often ineffective for African and Hispanic patients, creating life-threatening situations or resulting in death or disability [8, 9].
Pharmacogenetic analysis can be applied not only to an individual but also to an entire population, in which case an average genotype based on the allele frequency in a studied population is analyzed. Indeed, individual genotypes may differ from the average population genotype. Even so, predictions based on the average genotype that reflects the characteristics of the population’s gene pool will work better than one-size-fits-all recommendations on drug selection and dosing.
This problem has inspired a wealth of studies researching the possibility of optimizing pharmacogenetic recommendations for a given population based on its genetic characteristics. For example, the average effective warfarin dose was shown to be different between European and Caribbean populations [10, 11]. The situation is further compounded by the fact that many populations are genetically different from the “typical” representatives of their race: many African populations are genetically very different from African Americans; Siberian peoples are different from the Chinese; Eastern Slavs and the populations of the Caucasus differ from white Americans. A striking variation in the frequency of ADME genes is reported between the Han Chinese, who make up 98% of China’s population, and other Mongoloid minorities, including Uyghurs, Kyrgyz and Kazakhs [12]. Likewise, black African populations are characterized by great genetic variation in the genes coding for P450 isoenzymes, compared to Caucasian populations [9]. Undescribed or rare alleles of ADME genes can substantially contribute to the efficacy and safety of pharmacotherapy. For example, a recent study has identified a novel allele responsible for up to 31% of interpatient warfarin dose variability in African Americans [8]. In another study of variability in warfarin dose response, rare alleles typically found in African Americans were detected in a Puerto Rican population [13]. Another genetic marker (HLAB*15:02) associated with severe allergic response to carbamazepine occurs in 8% of Asians and only 1% of Europeans; the prevalence of carbamazepine allergy differs five- to sixfold between these 2 populations [14]. This indicates that direct extrapolation of data generated by foreign studies to the Russian population is absolutely unacceptable.
Russia is a multiethnic state, hence significant variation in responses to pharmacotherapy and the frequency of ADME, drug target and hemostasis-controlling gene variants in the patients of different ethnic/regional descent. In 2019, a systematic review of publications looking into the geographic distribution of alleles of ADME genes in the Russian population [15] discovered that not all ethnic groups and not all studied polymorphisms were equally represented in the literature. Most studies included in the review focused on the major polymorphisms in the genes coding for cytochrome P450 isoenzymes and some factors of the ADME system [16–25]. Many Russian regions and ethnic groups remain understudied.
In view of the foregoing, we set a goal to explore the frequencies of a few significant ADME gene variants across Russia and the neighbor states and to create a draft version of the pharmacogenetic atlas of Russia. The goal implies a systematic approach that ensures the even, wide coverage of the studied territory and can be implemented owing to the data generated by population studies using genome-wide SNP panels.
METHODS
We analyzed a total of 50 populations inhabiting the main regions of North Eurasia and its neighbor states. Samples that formed the basis for our study were provided by the Biobank of North Eurasia, the largest repository of biological specimens collected from indigenous Russian populations and the populations of Russia’s neighbor states [26]. The Biobank provided 2011 samples representing 40 different populations; the average sample size was n = 50. The initially small datasets of dozens ethnic groups were pooled to form a larger population sample. By contrast, the initially large sample of Russians was divided into 3 geographically different populations. The resultant datasets were genotyped using an Omni-Exome genome-wide array of 4.5 million SNPs (Illumina; USA).
The list of pharmacogenetic markers was compiled from 2 major sources containing some overlapping data: Very Important Pharmacogene (VIP) summaries [27], which describe pharmacogenetic markers involved in pharmacodynamics, pharmacokinetics and, ultimately, response to pharmaceuticals, and a list of markers from the popular commercial OpenArray™ PGx Express Panel (Thermo Fisher Scientific; USA). The resultant list was extended to include a few promising pharmacogenetic markers characterized by distinct racial/ ethnic variation in frequency (CES1, PON1, IFNL3, ITGB3) and, thus, comprised a total of 95 pharmacogenetic markers. Of them, 55 were present in the Omni-Exome Illumina panel [28].
This dataset was expanded with the published data on 10 populations from China and the European countries that share a border with Russia [29–44]. The total number of the samples representing those 10 populations was 186; the average sample size was n = 19. The samples had been previously genotyped using the genome-wide panels of 600 thousand to 1 million SNPs. In some cases, the data on Russia’s ethnic groups generated by the published studies were pooled with the corresponding Biobank data. The analysis of this literature-based dataset revealed the presence of 53 SNPs of 95 pharmacogenetic markers included in our list.
The two datasets (data from the Biobank of North Eurasia and the published genome-wide sequencing data) were compared and found to share 45 pharmacogenetic SNPs polymorphic (frequency above 1%) in the populations of North Eurasia. This paper analyzes their geographic distribution. A total of 2,197 samples representing 50 North Eurasian populations were genotyped for those 45 SNPs. Characteristics of the studied populations are provided in tab. 1 the SNPs are listed in tab. 2.
Genome-wide genotyping data were pooled and analyzed; allele frequencies were calculated in PLINK 1.9 [45, 46]. A frequency matrix was generated for 45 pharmacogenetic markers in 50 populations of Russia and its neighbor states based on the genotypes of 2,197 samples (average sample size n = 44). Using the matrix, we constructed the gene geographic maps of allele frequencies in the populations of North Eurasia and bordering states. The maps were created in GeneGeo [47–49] using average weighted interpolation; the radius of influence was set to 2,000 km; the weight function power was set to 3 [48]. On the maps, the regions lying farther than 2,000 km away from the studied populations are highlighted in white. In population genetics, it is imperative to study an indigenous population in any given territory; it is this population’s habitat that is shown on a gene geographic map. The gene pools of immigrant populations can be easily reconstructed from the map using information about their migration sources.
To visualize the cartographic models, we applied a “universal” scale of frequency intervals developed for genetic markers with a broad variation in frequency [50]. Frequencies constituting the main body of the frequency “spectrum” (5–60%) are represented by equally sized intervals that follow each other at an еincrement of 5%; the space of low frequencies is represented by 3 intervals, while the space of high frequencies, by intervals with 10% increment. This grading approach allows keeping the number of intervals within a reasonable limit (it would be a challenge to distinguish between over 17 colors on a map). Frequencies below 1% (i.e., below the below the criterion of polymorphism 1%) are shown in the contrasting gray.
RESULTS
The gene geographic maps showing allele frequency variation for 45 pharmacogenetic markers in the populations of North Eurasia and its neighbor states are available on the web site (see the Appendix). The main characteristics of their allele frequency variation are provided in tab. 2. Although the geographic distribution of each marker is unique (there are no two identical maps), the analysis of the maps reveals 3 prominent patterns: clinal variation, uniform distribution and focal variation (see fig. 1– fig. 3). Since it would be impossible to describe all the maps in this publication, we selected 12 markers to illustrate each pattern (4 maps per pattern). These markers are briefly described in tab. 3.
Clinal variation
The clinal (gradient) pattern of allele frequency variation along the East–West axis dominates the gene pool of North Eurasian populations. The pattern was identified during the analysis of classic markers [49, 51] and then corroborated using the data on DNA markers [49]. Moreover, this pattern is typical for the most ancient populations of North Eurasia [49, 52]. This may suggest that the clinal variation pattern emerged in the earliest days of the North Eurasian population and has persisted ever since. Therefore, it is only natural that some of the studied pharmacogenetic markers are characterized by a very distinct clinal variation pattern (see fig. 1).
The distribution of VKORC1 rs9923231-T(А) frequencies on the gene geographic map follows a very distinct clinal pattern (fig. 1А): the lowest frequencies are observed in the West of European Russia and the Caucasus, increasing gradually toward Eastern Eurasia. Notably, the frequencies lying between the Western and Eastern “extremes” of the frequency spectrum are found in West Siberia (but not in the Ural region), similar to the first PCA component of the North Eurasian gene pool and the map of archeological Paleolithic sites [49, 52]. The lowest frequency is observed in the lands inhabited by Eastern Slavs and the populations of the Baltic region (0.3 ≤ q < 0.4; here and below frequency is designated by q). From there, the area of low frequencies stretches eastward to the Volga river and includes the habitat of the indigenous population of Mordovia. The frequency of the polymorphism gradually increases toward the West of Europe and southwards. The domain of high frequencies (q > 0.80) begins in the East of North Eurasia with Tuvan and Mongol populations; the polymorphism reaches its frequency peak (> 90%) on the Eastern coast of the continent in the indigenous peoples of the Amur region, Evens, Evenks and continental Buryats.
The map of COMT (rs4680-A) frequencies (fig. 1B) demonstrates the same pattern of clinal variation, but the frequency range is narrower (0.44 vs 0.65; see tab. 2). Peak values (0.6 ≤ q < 0.7) are observed in the West; however, in the East they fall by only 20–30%. Consequently, the genetic diversity there is much lower (FST = 0.02; see tab. 2) than on the previous map (FST = 0.07; see tab. 2). High frequencies (0.5 ≤ q < 0.7) are observed in the European part of the continent, but their domain expands to the East, covering the Mari, Chuvash, Udmurt, and Tatar populations of the Volga- Ural region. The region of average frequencies spreads further to the East, spanning the Yenisey River basin. But by and large, the area of high frequencies (0.2 ≤ q < 0.35) corresponds to the area of low frequencies on the previous map.
For CES1 (rs2244613-C) (fig. 1C), the pattern of clinal variation is almost a copy of the VKORC1 (rs9923231-T) pattern (see fig. 1A): the domain of maximum frequencies (0.55 ≤ q < 0.8) is in the East of North Eurasia, but the domain of average frequencies (0.40 ≤ q < 0.50) is again in West Siberia. Minimum frequency values (0.10 ≤ q < 0.25) are observed in Western Europe and are lower than in the populations of Eastern Slavs.
For IFNL3 (rs8099917-C) (fig. 1D), the pattern of clinal variation is weakened by the narrow frequency range (0.25; see tab. 2). However, similar to the previous map in fig. 1B, the lowest frequencies of this polymorphism are concentrated in the East of North Eurasia, whereas its maximum frequencies (0.24 ≤ q ≤ 0.28) are found in Europe (from Karelia to Italy) and in the Caucasus, although they did not cover the entire European mainland. The narrow range of low frequencies (0.02 ≤ q ≤ 0.28) makes the pattern of clinal variation less distinct due the growing role of a sampling error.
Uniform distribution
A feature with generally uniform spatial distribution will not necessarily occur at the same frequency at all points in space. Uniform distribution characterizes alleles that occur in almost every population under study but do not exhibit distinct clinal variation on the regional scale, although certain patterns might be identified in some parts of the studied region. Surges or dramatic falls in frequency in some parts of the region suggest a sampling error and the need to revise the number of the populations representing these areas and the sample size. But if these extreme behaviors are observed in more than one neighboring population, they are not evident of random frequency fluctuations but rather indicate a distinct frequency variation pattern in the studied group of populations.
The distribution of CYP2C9 (rs1057910-C) across North Eurasia (fig. 2А) is strikingly uniform. The average frequency (q = 0.08; tab. 2) of the marker is low, slightly above 5%. But in most ethnic groups inhabiting North Eurasia, the marker occurs at nearly identical frequency which varies within a narrow range (0.03 ≤ q ≤ 0.10). The only identifiable pattern pertains to the increased frequency of this marker observed in almost all populations of the Caucasus and the Transcaucasian region. Single spikes in Karelia, Tadzhikistan and Chukotka and a steep decline below 1% (the gray zone of Yakutia) do not form distinct patterns.
Likewise, ITGB3 (rs5918-G) (fig. 2B) is characterized by uniform geographic distribution, its frequency varies within a very narrow range (0.02 ≤ q ≤ 0.28), and its average frequency is low (q = 0.11). However, deviations from the overall uniform distribution now form a pattern: the area of high frequencies stretches in an almost uninterrupted fashion from the Baltic region to the estuary of the Yenisey River. The second frequency peak occurs in the South of North Eurasia: in Tadzhikistan and South Siberia.
The map of MTFHR (rs1801131-С) frequency variation (fig. 2C) shows that the marker is uniformly distributed across the vast territory, although its average frequency (q = 0.31; see tab. 2) is 3 times higher than the average frequencies from the previous maps. The domain of average frequencies (0.25 ≤ q < 0.35) spreads throughout almost the entire North Eurasia, with two peaks in Yakutia and Tadzhikistan.
The average frequency of ABCB1 (rs1045642-G) (fig. 2D) is q = 0.48 (see tab. 2); the scope of frequency variation is the same as on the previous map (0.32; see tab. 2), slightly exceeding the scope of frequency variation in fig. 2A (0.19) and fig. 2B (0.26). Average frequencies (0.40 ≤ q < 0.55) cover almost the entire territory of North Eurasia and the neighbor states. Slightly lower frequencies are observed in the North of Middle Siberia in the cluster of Khanty, Mansi, Ket, Nenets and Selkup populations, as well as in two European populations (in Karelia and Moldova). Small areas of increased frequencies are scattered across North Eurasia: frequencies q > 0.6 occur in the South-West of Europe, in Ashkenazi Jews, Altaians, Evens and Amur region peoples. These local deviations do not disrupt the generally uniform distribution of the marker.
Similar to the previous set of maps, this set demonstrates that the variation pattern does not depend on the position of the frequency on the frequency spectrum: both high and low frequencies can follow a pattern of uniform distribution across vast territories.
Focal variation
Uniform distribution and clinal variation are typical for all pharmacogenetic markers that occur almost everywhere in North Eurasia and the neighbor states. If a marker occurs in only one (large as it may be) part of the studied region and is not found in its other parts, the pattern of its distribution can be arbitrarily called “focal variation”. The “focal” frequencies are normally low. On the map, populations that either do not carry this marker in their genome or have it at < 1% frequency are highlighted in gray.
Factor II (rs1799963-А) (fig. 3A) has a frequency range of 0 ≤ q ≤ 0.10 (see tab. 2). Its domain stretches uninterruptedly across the Southern regions of North Eurasia, from the Mediterranean to Turkmenia, covering all populations of the Caucasus, excluding the Ingush and Chechen peoples, and reaches its maximum frequency in the Transcaucasia region. Only two populations outside this area are carriers of the marker: Mordovians (q = 0.05) and Siberian Tatars (q = 0.02).
The frequency of factor V Leiden (rs6025-А) (fig. 3B) varies within almost the same range (0 ≤ q ≤ 0.13; tab. 2), but the area is concentrated in is much vaster, covering the territories on the previous map and the substantial share of the population in Eastern Europe and the Volga-Ural region. Peak frequencies are observed in the South of Europe, Caucasus, and the Transural region (Udmurt, Mari and Chuvash peoples). Outside this area, the polymorphism occurs only in the Altai region, where its frequency is below 1%.
Although the frequency range of TPMT (rs1800460-А) (fig. 3C) is extremely narrow (0 ≤ q ≤ 0.04) and its peak frequency is below 5%, its domain stretches across the entire Europe (except for the far South-West, which might be explained by the small sample size representing Basque, Spanish, and French populations; n = 29), Ural, West Siberia, Kazakhstan, to Khakassia. Interestingly, this marker is not found in most populations of the Caucasus, except for the Central Caucasus (the Ingush, Ossetians, Chechens) and North Dagestan.
On its genogeographic map, CYP2C19 (rs4986893-А) (fig. 3D) occupies an enormous territory that spans the Asian part of the region, except for Tadzhikistan and Turkmenia, protruding into the European mainland, including Ciscaucasia (Nogais), the Caucasus (Ossetians), and the Volga region (the Mari, Udmurt, Tatar, and Chuvash). The frequency peaks in the Far East in the populations of the Amur region (q = 0.14), Evens (q = 0.10) and in Khakassia (q = 0.10). For this marker, the scope of frequency variation is narrow (0 ≤ q ≤ 0.14; see tab. 2), and the frequencies themselves are comparable to the markers in fig. 3 that cover a much smaller area. Maps in fig. 3 demonstrate that markers with low frequencies and low variation in frequency can cover from small to very extensive areas.
DISCUSSION
There are two types of differences between populations that matter in pharmacogenetics: differences in the presence of pharmacogenetic markers and differences in their frequencies. The first type describes the alleles that are widespread in one population but almost non-existent in the other (some can even be region-specific). The second type pertains to different (sometimes contrasting) allele frequencies of the marker in different populations.
Of 3 patterns identified in our study, clinal variation and uniform distribution describe differences in allele frequencies between populations across the studied territory; importantly, the markers characterized by these two distribution patterns are found in almost every population of the studied territory. The focal variation pattern reflects differences in the presence of pharmacogenetic markers between different populations.
The clinal variation pattern (see fig. 1) is characterized by the presence of 2 extremes on the frequency spectrum, with an area of intermediate values between them. The extremes are more common for the markers that exhibit great variation in frequency, although this may not always be the case: markers that have a very narrow frequency range often form a geographically distinct gradient pattern. In North Eurasia, the clinal variation pattern was observed for 6 of 45 markers (13% of the maps in the atlas; see tab. 2).
With uniform distribution (see fig. 2), differences in frequencies are either small or their spikes and falls occur sporadically across the entire studied territory. However, there are areas where allele frequencies follow certain patterns, which might become the object of future research. In North Eurasia, 12 of 45 markers followed the pattern of uniform distribution (27% of the maps; see tab. 2).
A marker that follows the focal variation pattern is common for one population, but occurs at almost negligible frequency below 1% or is totally absent in another. The area where this marker occurs may vary in size (fig. 3): from small compact foci to vast territories. Attempts to identify the pattern of marker variation in the “focal” area should account for the sampling error since small sample sizes and low marker frequencies can skew the picture. In our study, focal variation was the most common pattern observed for 27 of 45 markers (60% of the maps; see tab. 2).
A cartographic atlas is a systematic collection of intertwined, mutually complementary maps that form a single entity [52]. Our pharmacogenetic atlas includes maps for 45 ADME markers in 21 genes, but the total number of markers with proved pharmacogenetic significance approaches a few hundred [:lit_2, 53;]. This is one of the reasons why our collection of maps, which meets the formal requirements for a cartographic atlas [52], is referred to in this publication as the first draft version of the atlas. Our atlas covers only the key pharmacogenetic markers included in a genome-wide Illumina panel; other important markers are not included. The second reason is rooted in how comprehensive the study is. Our maps were based on a large number of populations (K = 50), but the average sample size was relatively small (n = 44); therefore, the maps reveal only general patterns of distribution for every studied marker, and the error in the frequency of a given marker in a given population might be substantial. This indicates the need for further data accumulation, which has already been initiated by a number of researchers [15].
Nevertheless, this first version of the pharmacogenetic atlas provides valuable information on the variation of each studied marker and allows for general conclusions to be drawn. For example, the atlas shows that the average frequency of the marker and its sporadic occurrence in individual populations should not be interpreted as an indication of its geographic distribution pattern. In order to identify the distribution pattern, other tools should be employed, of which a gene geographic map appears to be the most suitable. The analysis of all maps in aggregate can identify areas that display their own patterns of frequency distribution and therefore should be subjected to additional analysis. Among such areas are Western Europe, the Caucasus, the Far East, North Siberia, and some others. Other important patterns, not limited to the 3 patterns described in this paper are expected to be revealed across this vast territory as more populations are studied and more data is accumulated.
Our collection of maps provides a wealth of information that can be exploited in pharmacogenetic research: they show frequencies of each of 45 SNPs in different populations across the entire territory of Russia and the neighbor states. However, caution should be exercised when using the obtained figures, considering the following limitations: the sampling error; the fact that these frequencies represent indigenous populations; and the fact that they represent the entire population but not its single members.
The first limitation is an average sample size. In our study, it was 44 people (88 chromosomes). Consequently, if a marker has a frequency of 25%, the CI will include the margin of error of ± 6%. This CI is much narrower for the territory of Russia, where the samples are larger, and broader for its neighbor states (the samples of their populations available in the literature are quite small). To account for CI fluctuations, the maps for marker frequencies should be accompanied by reliability maps [55].
The second limitation is related to the fact that each region of the map is represented by the gene pool of its indigenous peoples. Therefore, the frequency of a pharmacogenetic marker in an ethnically Russian population of Siberia should be estimated from the maps of migration sources for this population, e.g. Central Russia, but not the maps of Siberia. Thus, knowing the genealogy of a patient, one can construct their map-based pharmacogenetic portrait in a situation when genotyping cannot be performed.
The third limitation is typical for all pharmacogenetic population studies: the genotype of an individual patient does not necessarily reproduce the average characteristics of the gene pool the patient represents; still, these average characteristics are the best possible approximation of an individual genotype. Therefore, it is possible to apply standard pharmacogenetic protocols to an individual patient, drawing on the average genotype based on the allele frequency in a given population. This approach has obvious limitations, but there is an internationally held opinion that such protocols are the best solution in cases when an individual genotype of the patient is unknown or genotyping poses a financial burden. In our future studies, we will assess the significance of population frequencies for the economy by investigating the costs of inadequate drug dosing.
CONCLUSION
Based on the data on 50 populations of Russia and its neighbor states, we created a cartographic atlas showing the geographic distribution of 45 pharmacogenetic markers. The gene geographic atlas is composed of 45 maps that demonstrate the frequency of the studied pharmacogenetic markers in a variety of populations across Russia. The maps are straightforward and easy to understand by specialists from different fields and with different qualifications.
The pharmacogenetic atlas of Russia and its neighbor states reveals 3 major spatial distribution patterns of ADME, drug target and hemostasis-controlling genes. Some of the studied markers follow the clinal variation pattern (a gradient change in frequency along the East–West axis), which is the main pattern of allele frequency variation in North Eurasian populations. Uniform distribution singles out a group of markers that occur at average frequency in most Russian regions. Focal variation is observed in the markers that are specific to a certain ethnicity/region and are absent on other populations.
The lack of information about the frequency of pharmacogenetic markers in Russia impedes the adoption of personalized treatment algorithms originally developed for West European populations. There are countless examples of insufficient efficacy of standardized dosing protocols in non- European populations. Adaptation of the existing protocols to Russian populations require information about the geographic distribution of clinically significant markers in Russian populations. This study has generated an extensive body of systematized data and thereby may contribute to research in this important clinical field that demands thorough planning and additional large-scale studies.