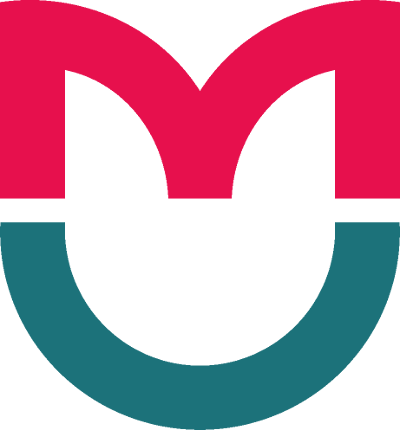
ORIGINAL RESEARCH
Study of the human brain potentials variability effects in P300 based brain–computer interface
Lomonosov Moscow State University, Moscow, Russia
Correspondence should be addressed: Ilya P. Ganin
Leninskie Gory, 1, str. 12, k. 246, Moscow, 119234, Russia; ur.liam@ninagpi
Funding: the study was funded by the Russian Science Foundation, project number 21-75-00021, https://rscf.ru/project/21-75-00021/
Author contribution: Ganin IP — research, data analysis and interpretation, literature analysis, preparation of the text; Kaplan AYa — data interpretation.
Compliance with ethical standards: the study was approved by Ethical Review Board at the Lomonosov Moscow State University (protocol number 2 of 11 October 2010) and carried out using EEG data (http://brain.bio.msu.ru/eeg_mov_matrix_BCI.htm) obtained by the authors and published earlier (https://doi.org/10.1016/j.neulet.2011.03.089).
The brain–computer interfaces (BCI) enable the use of executive devices without mediation of peripheral nerves and muscles. The technology involves recording and transformation of the electrical activity of the brain, most commonly by means of electroencephalography (EEG) [1]. The conventional scope of applications for BCI includes neurorehabilitation and replacement of speech and locomotion output in patients with severe motor impairments [2]. Other applications of BCI include their use as accessory means of instrumental diagnostics, e.g. in autism [3] or anorexia nervosa [4], as well as in cognitive training devices [5].
BCI systems based on exposure to external stimuli and detection of event-related potentials (ERP) by EEG are considered the most efficient in terms of communication and control [6]. A pioneering interface for text typing termed P300 BCI was firstly published in 1988 [7]. The user is presented with a letter matrix and receives the stimuli in the form of sequential highlighting of the letters. The mental response to the highlighting of target letters is accompanied by enhancement of certain ERP components, notably the P300 wave. Based on ERP analysis, the interface identifies the letter on which the user's attention is focused at the moment (target stimulus) [8].
The general classification principle in BCI (subdivision of EEG reactions into target and non-target classes) is based on the fundamental technique of ERP extraction and analysis. The technique employs the accumulation of epochs corresponding to identical repetitive stimuli as a substrate for ERP extraction. The averaging of these 'identical' epochs reveals a coherent ERP signal against the background noise which is incoherent to the moment of stimulation [6].
The variability of latency of individual EEG reactions from the moment of stimulation is a well-known neurophysiological phenomenon [9]. A failure to account for this source of variance can substantially distort the output of individual ERP components [10]. This effect involves both early and late components of EEG reactions [11, 12], with the averaged P300 wave being particularly vulnerable [13]. As a consequence, the amplitude of the component decreases and the width increases [14]. Beyond its fundamental interest, the temporal variability of ERP should be regarded as a major hindrance for P300 BCI classification accuracy.
The latency of ERP components, notably P300, is known to correlate with the age, cognitive status of the subject and other parameters [15, 16]. Deviations in characteristics of isolated responses to external stimuli can be observed in the divided attention tasks; the variability positively correlates with the complexity of the second task (i.e. its competitiveness for perception resources) [16]. The process of achieving a final goal with BCI (text typing) and execution of immediate instructions (reacting to stimuli) may be competing tasks themselves. In addition, the practical use of BCI technology in real-world settings is usually accompanied by collateral tasks and events that promote continuous variations in attention and perception [17]. It should be noted that additional source of multidirectional destabilization of ERP characteristics, including variability, involves the stimulation parameters per se: in BCI, the presentation rate is usually high, up to 4–5 stimuli per second [8], whereas the majority of standard protocols for ERP acquisition use presentation of one stimulus in 1–2 seconds [18].
From a neurophysiological perspective, variations in the brain output are rooted in the hierarchical complexity of the nervous system organization, so that these variations are generally considered inherent for the brain [19]. However, the elevated overall levels of such variation have been associated with certain pathologies. The abnormally high levels of neuronal noise and plasticity may interfere with the integrity of external stimuli processing and production of adequate behavioral responses, e.g. in autism [19, 20]. The increased variability of ERP was also demonstrated in patients with attention deficit hyperactivity disorder, especially under conditions of cognitive challenge [19, 21].
The cognitive fatigue of the user, a major cause of variability in EEG reactions [22], may negatively affect the neurocontrol efficacy in healthy users and even more so in patients. People with locomotion and speech impairments often have reduced attention capacities possibly accompanied by cognitive deficits. Such users tend to quickly get tired and may experience difficulties upon sustaining the control in BCI [23, 24].
Therefore, the effects of ERP variability in P300 BCI should be given immense consideration. On the one hand, proper understanding of the variability patterns will allow enhancement and optimization of the stimulus environment in terms of efficiency; on the other hand, it will mitigate the undesirable effects of variability to facilitate mastering of this technology by healthy users and notably by patients with neurocognitive impairments. This study aimed to analyze the effects of ERP variability in EEG reactions in order to minimize their influence on P300 BCI command classification accuracy.
METHODS
The study used EEG data obtained earlier in a modified version of P300 BCI with a stimulus matrix moving freely within the visual field. The details of this modification and some results obtained with its use were described by us previously [25]. The current study deals with identification and evaluation of ERP variability effects possibly encountered by users of such interfaces.
The recording was carried out at the Faculty of Biology, Lomonosov Moscow State University, and enrolled 12 participants (four men and eight women) aged 21–22 years. Inclusion criteria: healthy volunteers of both sexes, aged 18–35 years. Exclusion criteria: diagnosed neurological and/or mental conditions, a history of convulsive seizures episodes or diagnosed status epilepticus. The study initially intended to test the feasibility of ERP-based monitoring of the subject's attention to continuously moving target stimuli [25].
The participants (subjects) were presented with a 3 × 3 icon matrix, angular dimensions 7.4° × 7.4°, single stimulus size 2.2° × 2.2°. The stimulation was performed by highlighting (125 ms in every 500 ms) of the rows and columns of the matrix in random order.
The subjects were tasked with focusing their attention on a target stimulus within the matrix, carefully follow this stimulus, and mentally count the number of highlights encompassing this stimulus.
The study used various modes and velocities of matrix motion within the screen limits. The matrix moved at a constant speed in a straight direction inverted upon reaching the edge of the screen. A total of six modes were used in the study:
- 'static matrix' (motionless, positioned at the center of the screen);
- 'horizontal movement' (at 5°/s);
- 'vertical movement' (at 5°/s);
- 'random movement' (at 5°/s, the direction could change at random moments of time);
- 'velocity 10°/s' (horizontal movement);– 'velocity 20°/s' (horizontal movement).
Each participant was exposed to all modes succeeding in random order. Each mode encompassed presentations of 120 target and 240 non-target stimuli.
The EEG recordings were carried out with six scalp electrodes (Cz, Pz, PO7, PO8, O1 and O2) and a common reference electrode attached to ear lobule, using an NVX 24 electroencephalograph (Medical Computer Systems; Zelenograd, Russia) at 250 Hz discretization frequency. We used the CONAN-NVX software for the recording and original software written in Python 2.6 for the stimuli presentation. Synchronization of EEG recording with the highlightings involved a photodiode sensor. Simultaneously with the appearance of the target/non-target stimulus, a small white/ black square appeared in the upper right corner of the screen exactly beneath the sensor. The signal from the sensor was recorded along with the EEG data in a separate channel, and the change in brightness beneath the sensor made it possible to accurately determine the actual moments of presentation.
The signal processing including ERP extraction and analysis was carried out in MATLAB 9.11 (R2021b) (MathWorks; USA). The EEG signal was band-pass filtered within 0.5–20 Hz range (0.5–10 Hz for working with single epochs and calculating classification) using a fourth-order Butterworth filter and split into epochs from –400 to 1200 ms time-locked to the stimulus onset. The artifact epochs containing +/– 50 µV excess of signal amplitude in any of the channels were excluded. The percentage of excluded epochs was usually within 10%.
The epochs were classified into target and non-target and averaged within each class, subject and mode. The procedure yielded target and non-target ERP in a reduced –200 to 800 ms window. The amplitude of P300 was determined as the maximum signal value in Pz lead within a 300–600 ms window. The amplitude of N1 component was determined as the minimum signal value in PO7, PO8, O1 and O2 leads within a 100–300 ms window. Peak latencies were measured from the stimulus onset.
To analyze the component variability, P300 and N1 peak latencies were calculated similarly in the same channels and windows, albeit using single, non-averaged epochs. The epochs within each lead, mode and subject were sorted (ordered) based on these latencies. To analyze the variability of latencies, the median absolute deviation (MAD) value was calculated within each mode for each subject individually. To analyze the effect of component variability on calculated ERP, all epochs were centered on the peak time prior to averaging: each epoch was shifted by the subtracted difference between the latency in the averaged ERP and its own specific latency, forward or backward along the time axis, after which the epochs were averaged conventionally in a –200 to 800 ms window. In Cz and Pz leads the epochs were corrected by P300 and in occipital leads the epochs were corrected by N1. The peak amplitudes were subsequently calculated for the averaged corrected ERP. For the group analysis of N1 amplitudes, the values were calculated using the curves averaged over four occipital leads (PO7, PO8, O1 and O2).
To assess the variability of the ERP components subjectwise, the amplitudes were calculated for individual EEG epochs (raw and latency-corrected); in each epoch, the average signal value was calculated in a 52 ms window centered on the peak latency for a particular lead and mode.
To identify the effects of ERP variability on the command classification accuracy in P300 BCI, offline classification scores were calculated for all subjects in each mode, separately for the initial averaged ERP and for the latency-corrected epochs. The feature vectors for the linear Fisher discriminant analysis in each mode were built based on signal amplitudes in all EEG channels, spanning 600 ms post-stimulation (one point per 50 ms). The classification accuracy was assessed by cross-validation (leave-one-out) with sequential testing of each epoch with a classifier trained on all other epochs of the same mode. The procedure was repeated for all epochs, and the classification accuracy was assessed as the percentage of correctly identified epochs (two classes: target and non-target). To correctly calculate the accuracy before classification, the quantities of target and non-target epochs were equalized by randomly deleting a subset of non-target epochs. To exclude sampling-related variations, this classification process was repeated 100 times with random elimination of non-target epochs and the accuracy values obtained over 100 iterations were averaged.
All quantitative data (amplitudes, latencies and classification accuracy values) were analyzed using STATISTICA 7.0 package. One- or two-way analysis of variance (ANOVA) was used for group analysis. The Tukey's or Benjamini–Hochberg's posthoc tests were applied in cases of significant main effects in pairwise comparisons. The analysis of component amplitudes within subjects involved the normality check by χ2 (Chi-square) test followed by paired Student's t-test.
RESULTS
To visualize the accumulations of single EEG epochs (before ERP averaging), the time was plotted horizontally, the epochs were plotted vertically one by one and the amplitude values were color-coded [11]. This method allows representation of different grouping options for individual epochs and accentuates the effects of their variability. Fig. 1 (fig. 1) shows an example of such representation of target epochs for a single participant, acquired in Pz and PO8 using the 'static matrix' mode. In fig. 1A, arrangement of the epochs from top to bottom corresponds to their actual chronological order. In Fig. 1B, the epochs are sorted by latency so that epochs with earlier peaks of P300 (in Pz) or N1 (in PO8) are located at the top. Fig. 1C (fig. 1) shows the latency-corrected epochs, i.e. adjusted with the use of averaged latency value for particular mode and channel. For better clarity, we applied vertical smoothing by the 'moving average' in 10 epoch series.
Fig. 2 (fig. 2) shows ERP obtained by averaging of raw and latencycorrected epochs for the same participant (subject). The latency-corrected amplitudes of both components significantly exceeded the initial values obtained by raw averaging without correction for the peak latency. These differences were significant for all subjects in all modes (p < 0.001, paired Student's t-test).
We further analyzed the influence of latency correction procedure on the calculated amplitudes of P300 and N1 components at the group level; the results are presented in fig. 3. The amplitudes of P300 and N1 obtained with the latency-corrected epochs were significantly higher compared to those calculated by conventional method. Two-way ANOVA ('latency correction' factor — two levels, 'motion type' factor — four levels including the 'static matrix' mode) revealed significant effect of latency correction on the amplitudes of P300: F(1,11) = 95.7 and λ = 0.10 at p = 0.000001, and N1: F(1,11) = 58.1 and λ = 0.16 at p = 0.00001. The 'motion type' factor had significant effect on P300: F(3,9) = 7.5, λ = 0.29 at p = 0.008 (a lower amplitude for horizontal movement), but not N1.
The analysis of 'latency correction' and 'velocity' factors (comprising, respectively, two and three levels) revealed significant influence of the 'latency correction' factor on the amplitudes of P300: F(1,11) = 88.5 and λ = 0.11 at p = 0.000001, and N1: F(1,11) = 46.6 and λ = 0.19 at p = 0.00003, despite the lack of significant influence from 'velocity', and significant interaction between the two factors for N1 component: F(2,10) = 10.4 and λ = 0.32 at p = 0.0036 (a tendency towards lower amplitude at the highest velocity identified with the use of conventional averaging of the epochs).
The group analysis of ERP variability using MAD indicator of the peak latency revealed certain statistically significant effects. The movement velocity factor (three levels) significantly affected N1 component in PO8, O1 and O2 leads: F(2,22) = 4.4 at p = 0.024, F(2,22) = 3.8 at p = 0.037 and F(2,22) = 4.9 at p = 0.017, respectively. Post-hoc analysis revealed a higher variability (expressed through MAD) upon using the highest velocity mode compared to slower movement. The effects observed in O1 and O2 leads were significant (p < 0.05), whereas in PO8 the differences amounted to a trend (p < 0.1). The analysis revealed no significant effects of the 'motion type' factor on N1 component and the 'motion type' and 'velocity' factors on P300 component.
Fig. 4 (fig. 4) compares the offline classification accuracy for the standard ERP extraction algorithm as compared to the use of P300 and N1 peak latency-corrected data. Two-way ANOVA ('latency correction' factor — two levels, 'motion type' factor — four levels) revealed significant effect of the latency correction procedure on classification accuracy: F(1,11) = 102.7 and λ = 0.09 at p = 0.00001. Post-hoc analysis revealed higher classification accuracies when using latency-corrected epochs in all four modes (94.7, 92.2, 93.2 and 94.8%) compared with the conventional ERP extraction procedure (respectively, 78.3, 78.1, 78.3 and 76.1%; p < 0.0001 for all modes). Two-way ANOVA ('latency correction' factor — two levels, 'velocity' factor — three levels) revealed significant effects of both the calculation method and the matrix movement velocity: F(1,11) = 110.0 and λ =0.09 at p = 0.00000; F(2,10) = 6.0 and λ = 0.46 at p = 0.0196, as well as significant interaction between these factors: F(2,10) = 11.5 and λ = 0.30 at p = 0.0026. Posthoc analysis revealed higher classification accuracy when using latency-corrected epochs in all three modes (92.2, 93.7 and 94.0%) compared with the conventional ERP extraction procedure (respectively, 78.1, 76.7 and 71.2%; p < 0.0005 for all modes). Of note, in the highest velocity mode, the accuracy was significantly lower than in two other modes (71.2% vs 78.1 and 76.7%; p < 0.05) unless the latency correction was applied.
DISCUSSION
Overall, the obtained results confirm that ERP variability is inherent to P300 BCI and should be considered as a major influence on the shapes of ERP components; the exact impact depends on the degree of attention involvement. Correction of such variability at the level of single EEG reactions can substantially improve the command interpretation accuracy.
Despite the fact that ERP approach relies on the averaging of multiple EEG reactions to a stimulus, at certain signal processing parameters the detection of individual reaction peaks is quite feasible. This statement is illustrated well by our data (fig. 1) along with other studies [9, 10, 12]. Importantly, the analysis of single epochs allows correction for the variable peak latency in individual realizations of the response to ultimately afford a better extraction quality and enhanced amplitude for the components of interest (fig. 2). Despite the well-established phenomenon of variable latency, prediction of its specific impact in P300 BCI is nontrivial, as the conventional BCI stimulation rates are considerably higher compared with those typically used in psychophysiological studies. One one hand, this difference can mitigate the variability and stabilize the temporal heterogeneity of the reactions; on the other hand, it might also augment the heterogeneity and complicate correct interpretation of the stimulus at higher rates of presentation, as the suboptimal conditions for the attention/concentration activity may promote a concomitant increase in ERP variability [20]. The variable latency has been attributed to the inherent variance of time required for perception and categorization of the stimulus in every single presentation event [26]. We show that accounting for the variability effects allows significant enhancement of the amplitude for components that represent attention to target stimuli at both individual and group levels (fig. 2 and fig. 3).
Despite the lack of significant effects of different matrix motion modes on the amplitudes, MAD index showed increased variability of N1 component at higher velocities of the matrix. The decrease in visual acuity upon increase in the speed of tracked objects [27] has been associated with a concomitant increase in attention costs. Given the profound association of ERP components with specific features of oculomotor functionality [28], the observed increase in N1 component variability at higher velocities can be explained by the inherent attention variance combined to the pressing demand for tracking the matrix cells. The observation is also consistent with the decreased amplitude of N1 component in the difference (target — non-target) waveforms reported by us previously [25]. Noteworthy, the effect is characteristic of this earlier component, sensitive to target events at the eye fixation point [29], but not of the later P300 component.
Apart from its fundamental relevance, the developed correction procedure is of clear applied interest. The accounting for the latency factor in ERP components significantly rescued the accuracy of target stimuli classification in the modified P300 BCI environment used by us in this study (fig. 4). The temporal variability of ERP has been already featured as a putative cause of poor individual performance in BCI [17]. However, the authors suggested to improve the accuracy by using the amplitude values of the classifier output instead of the raw peaks in EEG epochs, which may seem less consistent and efficient, as the classifier output is more prone to external influences (noise, etc.). Another study has indeed succeeded in increasing the accuracy of P300 BCI output by means of latency correction for P300, albeit in a narrow window; overall, the method provided no classification benefits compared with the conventional approach [26]. Besides, the authors themselves emphasize irrelevance of their algorithm for online use, since it requires the explicit target/non-target labeling of each epoch. By contrast, our setting significantly enhances the classification accuracy through correction of the latency for both P300 and N1 components known to provide comparable contributions [30] and is equally suitable for the online mode as the correction is applied to both target and non-target epochs. It should be noted that the initial accuracy at the highest velocity was lower (71%) than in other modes (76–78%) and that introducing the latency correction rendered the accuracy ubiquitously high (92–95%) in all modes. This result underscores the utility of the developed correction approach in similar and even more attention-demanding BCI operation modes.
CONCLUSIONS
The dedicated analysis at the level of single EEG epochs enables overall correction for the variable latencies in a modified P300 BCI environment. Correction for this major source of variability refines the target ERP components with a concomitant improvement in the command classification accuracy. By using a movable stimulus matrix, we demonstrate particular relevance of the developed correction procedure under conditions of increased cognitive demand modeled by higher movement velocity. Taken together, our findings underscore the importance of accounting for ERP variability in the development of P300 BCI environments and provide the basis for creation of advanced ERP-based systems of neurocontrol, particularly those intended for people with reduced attention capacities.