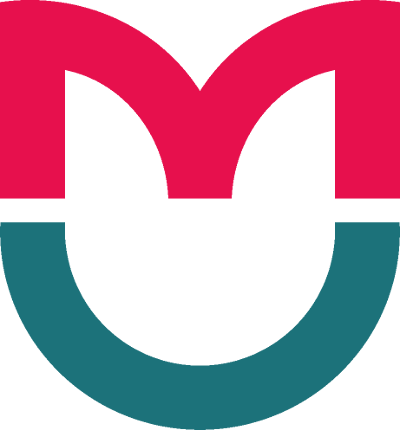
This article is an open access article distributed under the terms and conditions of the Creative Commons Attribution license (CC BY).
ORIGINAL RESEARCH
Study of the human brain potentials variability effects in P300 based brain–computer interface
Lomonosov Moscow State University, Moscow, Russia
Correspondence should be addressed: Ilya P. Ganin
Leninskie Gory, 1, str. 12, k. 246, Moscow, 119234, Russia; ur.liam@ninagpi
Funding: the study was funded by the Russian Science Foundation, project number 21-75-00021, https://rscf.ru/project/21-75-00021/
Author contribution: Ganin IP — research, data analysis and interpretation, literature analysis, preparation of the text; Kaplan AYa — data interpretation.
Compliance with ethical standards: the study was approved by Ethical Review Board at the Lomonosov Moscow State University (protocol number 2 of 11 October 2010) and carried out using EEG data (http://brain.bio.msu.ru/eeg_mov_matrix_BCI.htm) obtained by the authors and published earlier (https://doi.org/10.1016/j.neulet.2011.03.089).
The P300-based brain–computer interfaces (P300 BCI) allow the user to select commands by focusing on them. The technology involves electroencephalographic (EEG) representation of the event-related potentials (ERP) that arise in response to repetitive external stimulation. Conventional procedures for ERP extraction and analysis imply that identical stimuli produce identical responses. However, the floating onset of EEG reactions is a known neurophysiological phenomenon. A failure to account for this source of variability may considerably skew the output and undermine the overall accuracy of the interface. This study aimed to analyze the effects of ERP variability in EEG reactions in order to minimize their influence on P300 BCI command classification accuracy. Healthy subjects aged 21–22 years (n = 12) were presented with a modified P300 BCI matrix moving with specified parameters within the working area. The results strongly support the inherent significance of ERP variability in P300 BCI environments. The correction of peak latencies in single EEG reactions provided a 1.5–2 fold increase in ERP amplitude with a concomitant enhancement of classification accuracy (from 71–78% to 92–95%, p < 0.0005). These effects were particularly pronounced in attention-demanding tasks with the highest matrix velocities. The findings underscore the importance of accounting for ERP variability in advanced BCI systems.
Keywords: event-related potentials (ERP), brain–computer interface (BCI), electroencephalography (EEG), P300 component, N1 component, temporal variability of ERP