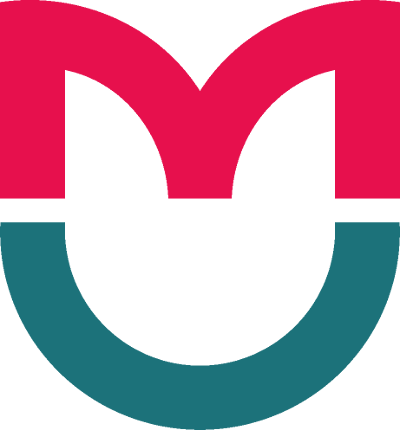
This article is an open access article distributed under the terms and conditions of the Creative Commons Attribution license (CC BY).
ORIGINAL RESEARCH
Adipokines and myokines as indicators of obese phenotypes and their association with the gut microbiome diversity indices
1 Rostov State Medical University, Rostov-on-Don, Russia
2 Center for Molecular Health, Institute of Digital and Translational Biomedicine, Moscow, Russia
3 Pirogov Russian National Research Medical University, Moscow, Russia
4 Kazan Federal University, Kazan, Republic of Tatarstan, Russia
Correspondence should be addressed: Lilia A. Ganenko
Nakhichevansky pereulok, 29, Rostov-on-Don, 344022, Russia; ur.xednay@ailil.oknenag
Funding: the study was conducted as part of the State Assignment № 0373100122119000041, the project “Creation of a bank of blood serum and fecal samples from healthy donors and patients with obesity, metabolic syndrome, type 2 diabetes mellitus, impaired mucosal barrier of the gastrointestinal tract with the aim of identifying candidate species-specific mediators of quorum sensing microbiota systems human modulating endocrine and metabolic function of adipose tissue”.
Author contribution: Ganenko LA — study concept, data acquisition and analysis, manuscript writing; Volkova NI — research planning and management, manuscript editing; Roumiantsev SA — study design, manuscript editing; Grigoryeva TV — working with the database, analysis of the results; Laikov AV — data analysis and interpretation of the results; Vasilyev IYu — study concept, manuscript editing; Gaponov AM — literature review, study design, manuscript editing; Naboka YuL — data analysis and interpretation of the results, manuscript editing; Shestopalov AV — search for sources of funding, working with data sets, statistical data processing, manuscript writing.
Compliance with ethical standards: the study was approved by the Ethics Committee of the Rostov State Medical University (protocol № 20/19 of 12 December 2019); the informed consent was submitted by all study participants.
Complications of obesity reduce the quality of life and life expectancy [1]. However, obesity is not always associated with metabolic disorders, that is why metabolically healthy obesity (MHO) and metabolically unhealthy obesity (MUO) are distinguished [2]. The prevalence of MHO among obese patients is 6–60% depending on the criteria of MHO [3]. Obesity classification that is based on BMI does not provide a full picture of the health status and potential risk of adverse outcome. This has been documented in the AACE/ACE (American Association of Clinical Endocrinologists and American College of Endocrinology) guidelines (USA, 2014) suggesting to take into account metabolic status when classifying obesity [4]. The adipose and muscle tissues play an important role in maintaining metabolic homeostasis via secretion of biologically active peptides: adipokines and myokines [5]. Imbalance between these insulin sensitivity and adipogenesis increasing and reducing adipokines and myokines may contribute to metabolic complications, such as insulin resistance, slow inflammatory adipose tissue remodeling, extracellular lipid deposition, atherosclerosis, etc. [6]. It is known that adiponectin exerts insulin sensitizing, anti-atherogenic and antiinflammatory effects [7]. In contrast, such adipokines as leptin and resistin are potential factors of insulin resistance that have pleiotropic effects on food intake, neuroendocrine control of hypothalamus, reproductive function, and energy metabolism [8]. Information about the recently discivered adipokine, asprosin, is extremely fragmented: it is believed that asprosin can affect glucose metabolism, that it is probably associated with obesity, and that asprosin levels can be used as a marker for early diagnosis of diabetes mellitus [9]. Currently, information about the role of myokine (myostatin) in the pathogenesis of obesity and insulin resistance is limited. Some authors suggest using myostatin for identification of MUO [10]. The insulinresponsive myokine, FGF21, functions as a metabolic factor involved in the glucose homeostasis control [11]. Recently the interest of researchers has been drawn to the osteocrin peptide that is secreted by fibroblasts and osteoblast-like cells. Its pathophysiological role is poorly understood [12]. Sporadic results obtained in obese patients with insulin resistance showed that had higher osteocrin levels than healthy individuals [13]. In the last decade, gut microbiota has been considered as a factor affecting the development of obesity. Microbiota can influence the metabolic phenotype via both direct effects on the energy and nutrient availability and modulation of the microorganisms' signalling and metabolic pathways. There is growing evidence that the balance and diversity of microorganisms are essential for both optimal interaction with macroorganism and prevention of extraintestinal diseases [14]. The diversity of microbiome reflects the abundance of various bacterial species. Two types of diversity are distinguished: alpha diversity (within the same biotope) and beta diversity (between distinct biotopes). Alpha diversity is assessed based on the Chao 1, Shannon, Simpson (direct and inverse), Gini–Simpson, and Berger–Parker indices. The Chao 1 index is used to estimate the observed/hidden diversity, the Shannon index is an estimator of the microbiome diversity and evenness (higher index values correspond to wider diversity); the Simpson index describes the probability that any two specimens randomly selected in the indefinitely large community belong to different species (the diversity decreases with the increasing index); the Berger–Parker index is a measure of the species dominance in the microbiome (the larger is the index, the lower is alpha diversity) [15]. Previous studies have shown that the stool microbiome of obese individuals is less diverse than that of lean people [16]. The decrease in mocrobial diversity in 75% of patients with morbid obesity and 40% of patients with class I and II obesity has been also shown [17, 18]. Some authors have found a positive correlation between the decrease in the gut microbiome diversity and MUO [19]. Despite the fact that the number of studies aimed at assessing the role of adipokines and myokines in MHO, as well as the relationship between adipokines and myokines and the gut microbiome indicators, is limited, the associations of adiponectin, resistin, FGF21 with MHO reported in the literature [5, 6] have provided the basis for the scientific hypothesis used in our study: various profiles of adipokines and myokines may contribute to phenotypic dissociation of obesity. The study was aimed to reveal the features of adipokine and myokine levels and their association with the gut microbiome diversity indicators in patients with different obese phenotypes.
METHODS
The study was performed in the Rostov State Medical University, Kazan Federal University, and the Center for Molecular Health at the Institute of Digital and Translational Biomedicine. The patients were enrolled between 2019 and 2020. All the subjects were divided into two main groups based on the body mass index (BMI) and waist circumference (WC): healthy individuals (controls) were included in group 1, while obese patients were included in group 2. Then group 2 was divided into two subgroups depending on whether or not metabolic complications were present: subgroup 2а included patients with MHO and subgroup 2b included patients with MUO. Inclusion criteria: age over 18 years; taking no antibiotics, pre- or probiotics within three months before enrollment. Additional inclusion criteria for group 1: 18.5 kg/m2 < BMI ≤ 24.9 kg/m2; no dyslipidemia, hyperglycemia or arterial hypertension (AH). Additional inclusion criteria for group 2: BMI ≥ 30 kg/m2, WC > 102 cm in males and > 88 cm in females. Subgroup 2а included patients who met more than three criteria, while subgroup 2b included those who met ≥ three criteria of the National Cholesterol Education Program (NCEP), Adult Treatment Panel III (ATPIII) (NCEP-ATP III) including the following indicators: systolic blood pressure (SBP) > 130 and/or diastolic blood pressure (DBP) > 85 mm Hg, fasting plasma glucose (FPG) ≥ 6.1 mmol/L, triglycerides (TG) ≥ 1.7 mmol/L, high density lipoprotein cholesterol (HDL-C) < 1.29 (females) or < 1.03 (males), waist circumference (WC) > 102 cm (males) or > 88 cm (females). Exclusion criteria: severe somatic disease, any gastrointestinal disorder, acute condition, depression, alcohol abuse, pregnancy.
Study design
A cohort study was performed. Clinical interview and examination, as well as assessment of anthropometric indicators were performed in all subjects; all of them were through questionnaire survey and the laboratory tests: fasting plasma glucose (FPG), total cholesterol (TC), low density lipoprotein cholesterol (LDL-C), high density lipoprotein cholesterol (HDL-C), triglycerides (TG), adiponectin, leptin, asprosin, resistin, vascular endothelial growth factor (VEGF), irisin, fibroblast growth factor 21 (FGF21), myostatin, osteocrin, and insulin. The insulin resistance index (HOMA-IR) was calculated, the composition of gut microbiome was defined, and the alpha diversity indices of bacterial phylotypes were computed. Blood pressure was measured using the manual blood pressure monitor (Little Doctor LD-60; China) using the standard method by N.S. Korotkov. Biochemical parameters, such as FPG, HDL-C, LDL-C, TG, TC (mmol/L), were assessed by photometry using the Hitachi U-2900 spectrophotometer (Hitachi; Japan) and the Olvex Diagnosticum kits (Russia).
Adiponectin, leptin, resistin, asprosin, VEGF, irisin, myostatin, FGF21, osteocrin, and insulin (ng/mL) were quantified by multiplex immunoassay using the Magpix analyzer (BioRad; USA) and the kits manufactured by Milliplex (USA). The concentration of asprosin was determined by ELISA using the ELISA KitForAsprosin (Cloud-Clone.; USA). Faecal samples were collected in accordance with the handbook edited by V.V. Menshikov [20]. Gut microbiome composition was defined by Next-Generation Sequencing (NGS) of the bacterial 16S rRNA gene (V3-V4 variable region) on the MiSeq platform (Illumina; USA) in the paired-end reading mode (2 × 300). Various alpha diversity indices of the bacterial community were used to characterize microbiota.
Statistical analysis
R environment (version 3.2, R Foundation for Statistical Computing; Vienna, Austria) was used for statistical processing. The following was calculated for quantitative indicators: mean ± standard deviation; median and quartiles (25%, 75%); minimum and maximum values of the sample. The average group levels were compared using the Mann–Whitney U test. The median values of the studied parameters were compared using the Kruskal–Wallis test, and the correlation analysis involved the use of Spearman's rank correlation coefficient. The differences were considered significant at р < 0.05. Cluster analysis: largescale correlation analysis was performed in Python v3.8 for each group of patients and the correlations between:
- BMI, WC, SBP, DBP, FPG, LDL-C, HDL-C, TG, TC and the levels of adipokines and myokines;
- each clinical parameter and microbiota parameters (alpha diversity: species richness (operational taxonomic units (OTUs) with non-zero abundance), Shannon entropy, Berger–Parker dominance index, Simpson index and inverse Simpson index, Gini–Simpson index, Chao1 index;
- microbiome parameters and the levels of adipokines and myokines.
The Spearman's rank correlation matrices obtained were clustered together within each group of patients based on the Manhattan distances.
Data availability
The cluster analysis scripts are publicly available from GitHub: https://github.com/ivasilyev/curated_projects/tree/master/ashestopalov/nutrition/obesity_elisa
RESULTS
Clinical and laboratory characteristics of the surveyed individuals of groups 1 and 2 are provided in tab. 1.
The control group and obese patients were matched for gender and the TC and LDL-C levels. Furthermore, the subjects of group 1 were younger (р = 0.032), they had lower BMI (р = 0.024) and WC (р = 0.011). Obese patients had higher SBP, DBP, TG, FPG, insulin levels, HOMA (р < 0.05) and lower HDL-C (р < 0.05) than the control group. Clinical and laboratory characteristics of the surveyed individuals of subgroups 2а and 2b are provided in tab. 2.
Subgroups 2а and 2b were matched for the number of males and females, subjects' age, BMI, and WC. When comparing patients with different obese phenotypes, significant differences in various indicators were revealed: SBP, DBP, TG, FPG, insulin, and HOMA were higher (р < 0.05) and HDL-C was lower (р < 0.05) in the MUO subgroup than in the MHO subgroup. Particularly noteworthy, however, was hyperglycemia in patients of the subgroup 2b that corresponded not to prediabetes, the early carbohydrate metabolism disorder, but to diabetes mellitus. By comparison, normal blood glucose levels were observed in the subgroup 2a, despite imsulin resistance revealed in both groups. In the subgroup 2b a more than twofold increase in blood levels of triglycerides suggesting triglyceride storage impairment due to the adipose tissue insulin resistance that resulted in lipotoxicity, i.e. extracellular triglyceride accumulation, was noteworthy.
Comparative analysis of the adipokine and myokine levels in the subjects of groups 1 and 2 revealed significant differences in the levels of adiponectin, leptin, and asprosin. Obese patients had lower adiponectin levels (р < 0.05) and higher leptin and asprosin levels (р < 0.05) than healthy individuals (tab. 3). It is known that leptin is a key mediator of interaction between the adipose tissue and the hypothalamic-pituitary axis that exerts orexigenic effect. High leptin levels in the group of obese patients suggest the leptin orexigenic effect inhbition due to resistance to appropriate signals developed by the central nervous system, i.e. these suggest the development of insulin resistance characterized by low satiety, excessive intake of nutrients, and weight gain.
When comparing serum levels of adipokines and myokines in patients with MHO, MUO and healthy donors the following earlier detected trends were observed: patients with MHO and MUO had significantly lower adiponectin levels (p < 0.05) and significantly higher leptin and asprosin levels (p < 0.05) compared to the control group. Furthermore, patients with MUO had lower adiponectin and leptin levels (p < 0.05) than patients with MHO. A significant increase in the levels of FGF21 was also observed in patients with MUO (tab. 4). Given its insulin sensitizing effect, the decrease in adiponectin levels fits into the general picture of insulin resistance. The lower leptin levels in patients with MUO that seem paradoxical at first glance are likely to result from the adipose tissue insulin resistance that leads to impaired terminal differentiation into adipocytes, abnormal extracellular triglyceride deposition, and lipotoxocity.
The correlation analysis has revealed positive correlations between the levels of adiponectin and resistin (r = 0.428; p = 0.001), FGF21 and myostatin (r = 0.543; p = 0.001), insulin and leptin (r = 0.429; p = 0.0001) in healthy donors. This suggests normal balance in the insulin sensitivity regulation system and the adipose and muscle tissue endocrine function.
Positive correlations between the adiponectin levels and the levels of insulin (r = 0.3; p = 0.008), leptin (r = 0.3; p = 0.008), resistin (r = 0.5; p < 0.0001), and VEGF (r = 0.49; p < 0.0001) were revealed in obese patients. Moreover, there were significant correlations between the levels of the FGF21 myokine and myostatin (r = 0.37; p = 0.0001), as well as between the levels of insulin and leptin (r = 0.84; p < 0.0001). The leptin levels were also correlated to the VEGF levels (r = 0.38; p = 0.002), while resistin levels were correlated to the osteocrin levels (r = 0.33; p = 0.0006). Such correlations are indicative of the imbalances in the regulatory system and the involvement of hypoxia-inducible factor in the regulatory system, since there are positive correlations between adiponectin and leptin and between leptin and VEGF.
Patients with MHO showed the following correlations of osteocrin levels: negative correlation with asprosin (r = –0.43; p= 0.02) and resistin (r = –0.39; p = 0.01), positive correlation with adiponectin (r = 0.43; p = 0.04). The relationships were revealed between the levels of adiponectin and myostatin (r = 0.34; p = 0.035), insulin and leptin (r = 0.59; p < 0.0001), FGF21 and adiponectin (r = 0.34; p = 0.0003).
The correlations of osteocrin with asprosin (r = –0.38; p = 0.04), resistin (r = 0.48; p = 0.001), and myostatin (r = 0,33; p = 0,03) were revealed in patients with MUO. There were significant positive correlations between FGF21 and myostatin (r = 0.37; p = 0.005), insulin and leptin (r = 0.37; p = 0.005). Thus, the role of osteocrin in regulation of the adipose and muscle tissue endocrine function becomes more and more important with the development of obesity. No correlations of osteocrin have been found in healthy individuals. There are two correlations of osteocrin with adipokines (asprosin and resistin) in the group with MHO and three such correlations that expand the range to the myostatin myokine in the group with MUO. Therefore, obesity is associated with the interrelated alterations in all three mesenchymal stem cell differentiation branches: adipogenic, myogenic, and osteogenic. It should be noted that positive correlations between insulin and leptin are observed in all studied groups. These are maximal in the group with MHO, which suggests that the effects of insulin on the adipose tissue are preserved, and are minimal in the group with MUO, which could be indirect evidence of the adipose tissue insulin resistance. Also noteworthy is the change of the FGF21 regulatory effects with the development of obesity. In healthy donors and patients with MUO, this myokine positively correlates with the other myokine, myostatin, while in patients with MHO is correlates with adiponectin and myostatin.
Perhaps the FGF21–adiponectin regulatory axis is one of the compensatory mechanisms in the adipose tissue–muscle tissue system that allows the adipose tissue to retain insulin sensitivity in obese patients and prevent lipotoxicity.
To assess alpha diversity of the bacterial community in the studied groups, we calculated the Simpson and Shannon phylogenetic diversity indices, Chao1 index, and OTUs. Significant differences in the phylogenetic diversity index and Chao1 index between controls and obese patients have been found, which suggest reduced alpha diversity in fecal samples of obese patients. However, there are no differences in the Shannon index between groups 1 and 2 [21, 22].
It should be noted that the Shannon index, Chao1 index, and OTUs were significantly higher in patients with MHO than in the group with MUO and the group of healthy donors. The increased alpha diversity is likely to be a protective mechanism of the microbial community that prevents metabolic disorders in obese individuals.
The large-scale correlation analysis that revealed positively related (r ≥ 0.3) clusters typical for each obese phenotype was performed in subgroups 2а and 2b. Thus, the following clustering type was typical for the subgroup of patients with MHO: 1) correlations of glucose levels with inverse gut microbiome diversity indices (Berger–Parker index, Gini–Simpson index, inverse Simpson index); 2) correlations of the levels of leptin, insulin, irisin and HOMA values with the VEGF concentrations; 3) correlations of the FGF21 and HDL-C concentrations with the direct gut microbiome diversity indices (Chao1 index, OTUs, Shannon index, Simpson index) (fig. 1).
Clustering of the correlation between glucose levels and inverse gut microbiome diversity indices demonstrates the relationship between microbiota and glucose levels, it suggests that gut microbiota of individuals with MHO affects glucose concentration and probably keeps glucose at normal levels, despite the presence of obesity. Clustering of the correlation between VEGF levels and the carbohydrate metabolism indicators together with insulin sensitivity shows that hypoxia has a regulatory effect in individuals with MHO. Clustering of the correlation of the direct gut microbiome diversity indices with the FGF21 and HDL-C levels suggests that microbial diversity has a protective effect in individuals with this obese phenotype, probably due to preservation of the microbiota–liver–adipose tissue regulatory axis.
It is important to note that no clustering of the gut microbiota diversity indicators with the glucose levels typical for MHO was observed in patients with the MUO phenotype. This suggests the loss of gut microbiota influence on the regulation of blood glucose levels (fig. 1). Furthermore, inverse diversity indices in individuals with MUO were highly correlated to BMI, SBP, DBP, LDL-C, TC, HOMA-IR, and insulin, while direct gut microbiome diversity indices were highly correlated to myostatin, TG, VEGF, FGF21, resistin, and leptin. This suggests that the decrease in the microbial community diversity in individuals with this type of obesity is directly related to the clinical and biochemical manifestations of metabolic syndrome and type 2 diabetes mellitus.
The results obtained suggest that gut microbiota plays different regulatory roles in the gut microbiome–liver– muscle/adipose tissue axis in individuals with various obese phenotypes. The findings also provide evidence of the emergence of abnormal relationships associated with MUO.
DISCUSSION
The roles of microbial community and endocrine factors of adipose and muscle tissues in obesity are quite fully investigated [2, 4, 5]. However, these studies rarely addressed the differences between the obese phenotypes and the correlations of the microbiome characteristics with the adipokine and myokine levels. Our study revealed alterations in the serum adipokine and myokine profiles consisting of the significant decrease in the adiponectin levels amid the increasing levels of insulin, leptin, and asprosin. This generally reflected the development of insulin resistance, leptin resistance, and, therefore, impaired storage function of adipose tissue and appetite regulation. Downregulation of adiponectin is consistent with the data obtained by other authors [1, 6]. Similar patterns have been previously shown for the levels of leptin and insulin [1, 23]. Higher adiponectin levels and lower leptin levels in healthy individuals are probably due to the lack of terminal adipocyte hypertrophy, preserved adipocyte storage function and metabolic plasticity. That is why such adipocytes must be characterized by high insulin resistance. At the same time, obese individuals may show hyperplasia and hypertrophy of adipocytes, the cytoplasm of which is completely filled with TG. These block further TG deposition in accordance with the negative feedback principle and, consequently, disrupt adipocyte metabolic plasticity [24]. Such remodeling results in ectopic fat accumulation and altered adipocyte morphology, being the triggers of insulin resistance associated with the with MUO decreased adiponectin levels and the increased leptin levels. Elevated leptin levels are probably associated with leptin resistance, in which smaller amounts of leptin would reach the brain, thereby decreasing activation of the signaling pathway for body weight regulation. Leptin resistance at the blood-brain barrier (BBB) level is due to the receptor saturation effects caused by excess leptin or reversible inhibition by circulating factors, such as TG [25].
Information about the role of asprosin in obesity is extremely fragmented [26]. The results of this study show higher asprosin levels in obese patients compared to healthy individuals. In the study the levels of asprosin in obese individuals are higher than that in healthy people. It is well-known that asprosin secreted by white adipose tissue increases appetite. Since obesity is associated with the increase in the number of adipocytes, then the asprosin levels increase, which, in turn, results in activation of orexigenic neurons by the agouti-related protein (AgRP) and increased appetite to form a vicious circle [27]. Therefore there are speculations that asprosin can contribute to the development of obesity by affecting food intake.
MHO is characterized by alterations that are similar in all types of obesity, however, lipoxicity manifestations are minimal. The maximum leptin levels, highest correlation between insulin and leptin, and the relationship between FGF21 and adiponctin are typical for this phenotype. On the one hand, this shows preserved effects of insulin in the adipose tissue, and on the other hand activation of the FGF21–adiponectin regulatory mechanism probably allowing to preserve insulin sensitivity of adipose tissue in individuals with this obese phenotype and to prevent lipotoxicity. The relationship between the gut microbial community diversity and the clinical and laboratory indicators suggests that microbiota is involved in regulation of glucose levels and that the diversity indices have a protective effect on the indicators of cholesterol metabolism, which is probably evidence of preserved microbiota–liver–adipose tissue regulatory axis.
As we have shown earlier, alterations in the share of the main microbiome members, Bacteroidetes and Firmicutes, associated with MHO are less severe than these associated with MUO. Perhaps these alterations are not critical for metabolic function of the microbial community as a whole, as well as for production of regulatory molecules and metabolites (indoles, kynurenines, short-chain fatty acids, secondary bile acids, vitamins, and essential amino acids), and are compensated by the increased diversity that consequently ensures preservation of the microbiota–liver–adipose tissue regulatory axis.
MUO is characterized by the most severe manifestations of the carbohydrate metabolism disorders, lipotoxicity, and dyslipoproteinemia. Furthermore, patients with MUO have lower adiponectin and leptin levels than patients with MHO. The significantly increased FGF21 levels have been also found in individuals with MUO. Perhaps, elevated FGF21 levels associated with MUO constutute a dysfunctional compensatory mechanism for hyperglycemia, since adipocytes that have reached critical hypertrophy show insulin resistance and are unable to utilise glucose. In this regard, there is a need to form an extra glucose metabolism pathway in order to relieve hyperglycemia. In particular, the increase in the muscle metabolism rate activates glucose utilization by the muscle tissue (myokine FGF21). It is important to note that the missing relationship between the glucose levels and the gut microbiome alpha diversity indicators is a feature of the MUO phenotype. This is indicative of the loss of regulatory effects by the gut microbiome in the microbiota‒liver‒adipose tissue axis and the emergence of abnormal relationships in individuals with this obese phenotype.
The cause of lower adiponectin concentrations in individuals with MUO compared to MHO is unknown, however, it can be related to the MUO-associated chronic hyperinsulinemia that suppresses adiponectin production by adipose tissue and thereby generates a feedback loop for the decreased insulin secretion caused by insulin resistance [3, 28]. In our study the leptin levels found in patients with MUO were significantly higher than these found in patients with MHO. Such results were consistent with the data obtained by other authors [29] and were possibly due to abnormal protein synthesis in hypertrophic adipocytes. However, the leptin contribution to the MUO pathogenesis needs to be clarified, since there are studies that have revealed significantly higher leptin levels in patients with MUO [30] or no differences at all [31]. In addition to the common significant correlations, the correlations typical for MUO have been revealed, such as a significant positive correlation between the myostatin and osteocrin levels. In contrast to patients with MHO, the gut microbiome alpha diversity indicators of patients with MUO significantly decreased and no longer correlated with blood glucose levels, while the inverse indices became correlated to the clinical and laboratory indicators of metabolic syndrome and type 2 diabetes mellitus.
CONCLUSIONS
Thus, the levels of leptin, asprosin, and insulin are sighificantly higher (р < 0.05) and adiponectin levels are lower in obese patients than in healthy individuals. Correlation analysis revealed imbalances in the regulatory system involving the hypoxia-inducible factor and interrelated changes in the adipogenic, myogenic, and osteogenic mesenchymal stem cell differentiation. Large-scale correlation analysis allowed us to reveal the loss of the microbiota diversity protective effects on metabolic processes in individuals with MUO. Further study of the relationship between gut microbiota and both lipid metabolism and carbohydrate indicators would contribute to further improvement of the therapeutic strategy for obesity and associated metabolic disorders through the development of the microbiome-based therapy of obesity. Furthermore, the data obtained may by used for early prevention of metabolic disorders and MUO conversion into MHO.