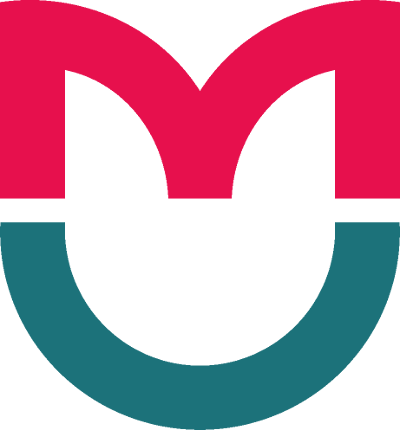
This article is an open access article distributed under the terms and conditions of the Creative Commons Attribution license (CC BY).
OPINION
Deep learning in modelling the protein–ligand interaction: new pathways in drug development
1 Shemyakin–Ovchinnikov Institute of Bioorganic Chemistry of the Russian Academy of Sciences, Moscow, Russia
2 Moscow Institute of Physics and Technology (MIPT), Dolgoprudny, Russia
3 Pirogov Russian National Research Medical University, Moscow, Russia
Correspondence should be addressed: Zinaida Mikhailovna Osipova
Miklukho-Maklaya, 16/10, Moscow, 117997, Russia; ur.hcbi@avoksakz
Funding: the study was supported by the Russian Science Foundation grant, project № 22-44-02024 (https://rscf.ru/project/22-44-02024/).
Author contribution: Barykin AD — literature review, manuscript writing, Chepurnykh TV — concept, literature review, manuscript writing and editing, Osipova ZM — project management, manuscript editing.
The deep learning technologies have become the driver of the revolutionary changes in scientific research in various fields. The AlphaFold-2 neural network software development that has solved the semicentennial problem of 3D protein structure prediction based on primary amino acid sequence is the most obvious example of using such technologies in structural biology and biomedicine. The use of deep learning methods for the prediction of protein–ligand interactions can considerably simplify predicting, speed up the development of new effective pharmaceuticals and change the concept of drug design.
Keywords: neural networks, deep learning, docking, protein–ligand interaction