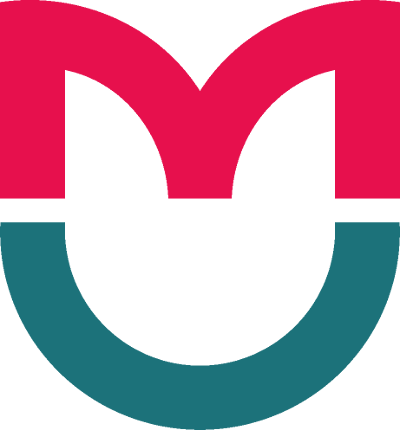
This article is an open access article distributed under the terms and conditions of the Creative Commons Attribution license (CC BY).
OPINION
Deep learning in modelling the protein–ligand interaction: new pathways in drug development
1 Shemyakin–Ovchinnikov Institute of Bioorganic Chemistry of the Russian Academy of Sciences, Moscow, Russia
2 Moscow Institute of Physics and Technology (MIPT), Dolgoprudny, Russia
3 Pirogov Russian National Research Medical University, Moscow, Russia
Correspondence should be addressed: Zinaida Mikhailovna Osipova
Miklukho-Maklaya, 16/10, Moscow, 117997, Russia; ur.hcbi@avoksakz
Funding: the study was supported by the Russian Science Foundation grant, project № 22-44-02024 (https://rscf.ru/project/22-44-02024/).
Author contribution: Barykin AD — literature review, manuscript writing, Chepurnykh TV — concept, literature review, manuscript writing and editing, Osipova ZM — project management, manuscript editing.
Computer (in silico) modelling of protein–ligand interaction plays a key role in biomedical research and represents one of the fundamental challenges of the ongoing process of developing new pharmaceuticals. The higher is the affinity and selectivity of the bioactive molecule binding with the receptor or enzyme, the more effective and safe the resulting drug candidate will be. The model reliability determines the quantity and quality of the candidate molecules, which will undergo the expensive procedure of chemical synthesis and testing in vitro and in vivo. Modelling often represents the key phase: this to the great extent determines the time and cost of the design, as well as the final price of the drug [1]. Until recently, there was no highly effective method for automated bioinformatics analysis of the protein–ligand interaction.
Conventional computer modelling methods
Molecular docking is a molecular modelling method predicting the best position of the ligand relative to the target protein that uses their 3D structures and the molecular interaction energy scoring functions. The scoring function learning (fig. 1А) is usually based on the set of the experimentally determined affinity values of protein binding to the ligands similar to the studied one. Thus, the prediction accuracy will depend directly on the similarity of the studied new candidate to the known ligands from the database.
The great diversity of scoring functions can be explained by insufficient reliability of each of these functions in case of solving a specific problem. Various scoring functions are more appropriate for various classes of ligands, however, there is no absolute guarantee of the result even when the method is selected correctly. That is why consensus assessment (using the data of several scoring functions at once) increases the likelihood of successful docking [2].
In case of rigid docking, the algorithms consider the ligand and target molecules as solids, while in case of dynamic docking the programs admit the possibility of conformational changes in the ligand associated with binding. The methods underlying the docking algorithms (fig. 1B) can be divided roughly into systematic and statistical. The systematic methods split molecule into several parts, thereby allowing one to assess affinity of interaction for each part, and then ensure covalent cross-linking of the parts in order to reassemble the ligand. Statistical methods to find the global energy minimum generate random changes, for each of which the thermodynamic state is estimated [3]. Statistical methods include the Monte Carlo method, tabu search, particle swarm optimization, and evolutionary algorithms. The systematic algorithms guarantee achievement of results in a finite number of steps (usually very large), while the statistical ones can “miss” the energy state of interest. However, in practice, statistical algorithms more often yield more reliable results than systematic ones.
In the last two decades, dozens of free and commercially available molecular docking programs have emerged: DOCK, AutoDock, Surflex, LigandFit, MCDock, LeDock, AutoDock Vina, rDock, UCSF Dock, and many more [4]. The programs usually use several algorithms at once, allowing one to adapt docking for specific enzyme–ligand pairs.
In the majority of cases, the modern protein–ligand docking methods correctly determine the ligand binding site and binding mechanism, however, these are unable to define its affinity accurately enough [5]. This significantly reduces the method applicability for discovery of new pharmaceuticals, since the candidate molecules are selected based specifically on the binding energy.
The molecular dynamics (MD) method is based on the use of the equations of atom motion and the empirical potential energy functions for calculation of interatomic interactions and characterization of the molecular system evolution over time. The interatomic interactions include elastic interactions (corresponding to covalent bonds) and Van der Waals forces. The most important post-processing methods to calculate free energy of the bond in the protein–ligand interaction complex also use the principles of molecular mechanics involving the Poisson–Boltzmann equation / Generalized Born model, as well as some additional approaches, such as thermodynamic integration and free analysis [6].
The molecular trajectory length determined by the number of simulation steps is the main limitation of the molecular dynamics method. The simulation time step should be comparable with the fastest motion in the system, i.e. with the bond fluctuations (1–2 fs). Thus, modelling slow processes, such as large domain motions and binding (µs–ms), requires many MD steps, which significantly increases the amount of computation. That is why detection of actual protein–ligand binding is a very rare phenomenon [7]. It was expected that the MD modelling based on the binding computation involving the use of molecular mechanics and the Poisson–Boltzmann equation would contribute significantly to the solution of real problems, such as identification of the most advantageous combination for the protein–ligand pairs with their further optimization.
Deep learning: another chapter in modelling the protein–ligand interaction
The first deep learning methods emerged in the mid-1960s, however, these became popular by the mid-2000s due to the increase in processing power and the emergence of large experimental data sets. Today, application of deep learning technologies to problems in various disciplines has yielded the results that are not inferior, and sometimes even superior, to the results yielded by conventional methods. Creation of the AlphaFold-2 algorithm predicting the protein tertiary structure based on the primary amino acid sequence within only a few minutes is the most obvious example [8], which has become a revolution in the field of structural biology.
The deep learning models were proposed for the prediction of protein–ligand interaction as an alternative to conventional docking based on the search for the free energy minimum [9]. The advantage of deep learning is that there is a possibility of studying the protein–ligand interaction based directly on the spatial arrangement of atoms, without selecting the mathematical parameters that not always reflect the actual binding mechanism. This method to predict protein–ligand interaction is currently developing rapidly: the DeepSite neural network model published in 2017 [10] correctly determined 23.8% of ligand binding sites in the specific dataset, while the Kalasanty neural network published in 2020 yielded the result of 44.6% for the same sample. In 2021, PUResNet significantly improved the prediction results (53% success for PUResNet vs. 51% for Kalasanty) [11].
To date, a wide variety of the neural network operation and training algorithms has been developed (fig. 2). Convolutional neural networks (CNN), graph neural networks (GNN), and transformer neural networks are usually used to study protein– ligand interactions. Convolutional neural networks consider the relationships between pairs of atoms through the prism of their relative spatial positions. The operation principle of graph neural networks is based on recording the threshold values aimed to determine the type of interaction between atoms (covalent or non-covalent). The use of the lower number of parameters represents the potential advantage of this approach. The combinations of several algorithms are also used, or the other modules (such as denoising autoencoder) that improve the outcome are added [12].
CONCLUSION
No definite leader has been revealed among various neural network architectures: accuracy of the results yielded by each algorithm depends on the types of proteins and ligands, affinity, and binding mechanism. According to the latest data, the egGNN and saCNN graph neural networks turned out to be the most successful in terms of ligand affinity prediction [13, 14], however, the differences from convolutional neural networks are not critical. We believe that this is due to the fact that no optimal prediction algorithm has yet been developed. Creating such an algorithm, judging by the pace of AI development in computational biology, is more likely to take years, not decades. Just like AlphaFold-2 changed the paradigm in the area of protein structure investigation in 2020, the use of artificial intelligence in biomedical research opens a new chapter in pharmaceutical industry and drug design.
The prospects of using AI for drug discovery have become apparent for the industry, since the use of AI significantly accelerates and reduces the cost of the conventional 12-year lifecycle of drug development. Over the past five years, almost all large pharmaceutical companies announced a partnership with the leading AI companies (Sanofi — Aily Labs, Pfizer — IBM, Novartis — Microsoft, AstraZeneca — Benevolent, etc.). The research details are likely to be protected by trade secret for a long time, however, press releases are being regularly issued that suggest introduction of deep learning into the ongoing R&D processes. Furthermore, more and more reports appear of the success of drug candidates designed using AI that are being prepared for or are through clinical trials. The examples include halicin (the promising broad-spectrum antibiotic, preclinical trial) [15], INS018_055 (drug for idiopathic pulmonary fibrosis, phase 2 clinical trial), REC-2282, REC-994, REC-4881, BEN-2293, EXS-21546, RLY-4008, EXS-4318, BEN-8744, etc. [16].
We believe that the search for the pool of new bioactive molecules will accelerate dramatically in the near future, and deep learning will become an essential element of the process of developing new pharmaceuticals. However, competent selection of the data that are used to train a neural network model still represents one of the topical problems on the path to the widespread use of deep learning for drug discovery, since the quality of these data is critical for reliability of predictions made by the model. In this regard, the task of the most effective training involving the use of incomplete or small datasets remains the main challenge faced by AI in the field of drug design [17].